Annual summary of 2019 Gwern.net newsletters, selecting my best writings, the best 2019 links by topic, and the best books/movies/anime I saw in 2019, with some general discussion of the year and the 2010s, and an intellectual autobiography of the past decade.
This is the 2019 summary edition of the Gwern.net newsletter (archives), summarizing the best of the monthly 2019 newsletters:
Previous annual newsletters: 2018, 2017, 2016, 2015.
Writings
2019 went well, with much interesting news and several stimulating trips. My 2019 writings included:
-
“Finetuning the GPT-2-117M Transformer for English Poetry Generation”
-
Danbooru2018 released: a dataset of 3.33m anime images (2.5tb) with 92.7m descriptive tags
-
“On Seeing Through ‘On Seeing Through: A Unified Theory’: A Unified Theory”
-
“Dog Cloning For Special Forces: Breed All You Can Breed”/NBA recruiting using height polygenic scores
I’m particularly proud of the technical improvements to the Gwern.net site design this year: along with a host of minor typographic improvements & performance optimizations, Inflation.hs
enables automatic updates of currencies (a feature I’ve long felt would make documents far less misleading), the link annotations/popups (popups.js
) are a major usability enhancement few sites have, sidenotes.js
eliminates the frustration of footnotes by providing sidenotes, collapsible sections help tame long writings by avoiding the need for hiding code or relegating material to appendices, and link icons & dropcaps & epigraphs are just pretty. While changes are never unanimously received, we have received many compliments on the overall design, and are pleased with it.
Site traffic (more detailed breakdown) was again up as compared with the year before: 2019 saw 1,361,195 pageviews by 671,774 unique visitors (lifetime totals: 7,988,362 pageviews by 3,808,776 users). I benefited primarily from TWDNE, although the numbers are somewhat inflated by hosting a number of popular archived pages from DeepDotWeb/OKCupid/Rotten.com, which I put Google Analytics on to keep track of referrals.
Overview
2019 was a fun year.
AI: 2019 was a great year for hobbyists and fun generative projects like mine, thanks to spinoffs and especially pretrained models. How much more boring it would have been without the GPT-2 or StyleGAN models! (There was irritatingly little meaningful news about self-driving cars.) More seriously, the theme of 2019 was scaling. Whether GPT-2 or StyleGAN 1+2, or the scaling papers, or AlphaStar, or MuZero, 2019 demonstrated the blessings of scale in scaling up models, compute, data, and tasks; it is no accident that the most extensively discussed editorial on DL/DRL was Rich Sutton’s “The Bitter Lesson”. For all the critics’ carping and goalpost-moving, scaling is working, especially as we go far past the regimes where they assured us years ago that mere size and compute would break down and we would have to use more elegant and intelligent methods like Bayesian program synthesis. Instead, every year it looks increasingly like the strong connectionist thesis is correct: much like humans & evolution, AGI can be reached by training an extremely large number of relatively simple units end-to-end for a long time on a wide variety of multimodal tasks, and it will recursively self-improve meta-learning efficient internal structures & algorithms optimal for the real world which learns how to generalize, reason, self-modify with internal learned reward proxies & optimization algorithms, and do zero/few-shot learning bootstrapped purely from the ultimate reward signals—without requiring extensive hand-engineering, hardwired specialized modules designed to support symbolic reasoning, completely new paradigms of computing hardware etc. (eg. 2019). Self-driving cars remain a bitter mystery (although it was nice to see in 2019 Elon Musk & Tesla snatch victory from the jaws of snatching-defeat-from-the-jaws-of-victory).
2019 for genetics saw more progress on genetic-engineering topics than GWASes; the GWASes that did come out were largely confirmatory—no one really needed more SES GWASes from Hill et al, or confirmation that the IQ GWASes work and that brain size is in fact causal for intelligence, and while the recovery of full height/BMI trait heritability from WGS is a strong endorsement of the long-term value of WGS, the transition from limited SNP data to WGS is foreordained (especially since WGS costs appear to finally be dropping again after their long stagnation). Even embryo selection saw greater mainstream acceptance, with a paper in Cell concluding (for the crudest possible simple embryo selection methods) that, fortunately, the glass was half-empty and need not be feared overmuch. (I am pleased to see that as of 2019, human geneticists have managed to reinvent 1918/1943’s breeder’s equation; with any luck, in a few years they may progress as far as reinventing 1943.) More interesting were the notable events along all axis of post-simple-embryo-selection strategies: Genomic Prediction claimed to have done the first embryo selection on multiple PGSes, genome synthesis saw E. coli achieved, multiple promising post-CRISPR or mass CRISPR editing methods were announced, gene drive progressed to mammals, gametogenesis saw progress (including at least two human fertility startups I know of), serious proposals for human germline CRISPR editing are being made by a Russian (among others), and while He Jiankui was imprisoned by a secret court there otherwise do not appear to have been serious repercussions such as reports of the 3 CRISPR babies being harmed or an ‘indefinite moratorium’ (ie. ban). Thus, we saw good progress towards the enabling technologies for massive embryo selection (breaking the egg bottleneck by allowing generation of hundreds or thousands of embryos and thus multiple-SD gains from selection), IES (Iterated Embryo Selection), massive embryo editing (CRISPR or derivatives), and genome synthesis.
VR’s 2019 launch of Oculus Quest proved quite successful, selling out occasionally well after launch, and appears to appeal to normal people, with even hardcore VR fans acknowledging how much they appreciate the convenience of a single integrated unit. Unfortunately… it is not successful enough. There is no VR wave. Selling out may have as much to do with Facebook not investing too much into manufacturing. Worse, there is still no killer app beyond Beat Saber. The hardware is adequate to the job, the price is nugatory, the experience unparalleled, but there is no stampede into VR. So it seems VR is doomed to the long slow multi-decade adoption slog like that of PCs: it’s too new, too different, and we’re still not sure what to do with it. One day, it would not be surprising if most people have a VR headset, but that day is a long way away.
Bitcoin: little of note. Darknet markets proved unusually interesting: Dream Market, the longest-lived DNM ever, finally expired; Reddit betrayed its users by wholesale purging of subreddits, including /r/DarkNetMarkets
, causing me a great deal of grief; and most shockingly, DeepDotWeb was raided by the FBI over affiliate commissions it received from DNMs (apparently into the tens of millions of dollars—you’d’ve thought they’d taken down those hideous ads all over DDW if the affiliate links were so profitable…)
What Progress?
As the end of a decade is a traditional time to look back, I thought I’d try my own version of Scott Alexander’s essay “What Intellectual Progress Did I Make In The 2010s?”, where he considered how his ideas/beliefs evolved over the past decade of blogging, as I started my own website a decade ago as well.
I’m not given to introspection, so I was surprised to think back to 2010 and realize how far I’ve come in every way—even listing them objectively would sound insufferably conceited, so I won’t. To thank some of the people who helped me, directly or indirectly, risks (to paraphrase Borges) repudiating my debts to the others; nevertheless, I should at least thank the following: Satoshi Nakamoto, kiba, Ross Ulbricht, Seth Roberts, Luke Muehlhauser, Nava Whiteford, Patrick McKenzie, SDr, ModafinilCat, Steve Hsu, Jack Conte, Said Achmiz, Patrick & John Collison, and Shawn Presser.
2010 was perhaps the worst of times but also best of times, because it was the year the future rebooted.
My personal circumstances were less than ideal. Wikipedia’s deletionist involution had intensified to the point where everyone could see it both on the ground and from the global statistics, and it was becoming clear the cultural shift was irreversible. Genetic engineering continued its grindingly-slow progress towards some day doing something, while in complex trait/behavioral genetics research, the early GWASes provided no useful polygenic scores but did reveal the full measure of the candidate-gene debacle: it wasn’t a minor methodological issue, but in some fields the false-positive rate approached 100%, and tens of thousands of papers were, or were based on, absolutely worthless research. AI/machine learning were exhausted, with state-of-the-art typically some sort of hand-engineered solution or complicated incremental tweaks to something crude like an SVM or random forest, with no even slightly viable path to interestingly powerful systems (much less AGI). At best, one could say that the stagnant backwater of AI, neural network research, showed a few interesting results, and the Schmidhuber lab had won a few obscure contests, and might finally prove useful for something over the coming years beyond playing backgammon or reading ZIP codes, assuming anyone could avoid eye-rolling at Schmidhuber’s website(s) long enough to read as far as his projections that computing power and NN performance trends would both continue (“what NN trends‽”); I noted to my surprise that Shane Legg had left an apparently successful academic career to launch a new startup called ‘DeepMind’, to do something with NNs. (Good for him, I thought, maybe they’ll get acquihired for a few million bucks by some corporation needing a small improvement from exotic ML variants, and then with a financial cushion, he can get back to his real work. After all, it’s not like connectionism ever worked before…) And reinforcement learning, as far as anyone need be concerned, didn’t work. Cryptography, as far as anyone need be concerned, consisted of the art of (not) protecting your Internet connection. Mainstream technology was obsessed with the mobile shift, of little interest or value to me, and a shift that came with severe drawbacks, such as a massive migration away from FLOSS back to proprietary technologies & walled gardens & ‘services’. All in all, there was not that much to draw interest in existential risk; if you had been interested, at the time, probably the best thing you could’ve done is focus on the standbys of nuclear war & pandemic (neither have been solved), or left your money invested to wait for more compelling charitable opportunities. The future had arrived, and had brought little with it besides 144 characters.
But also in 2010, disillusioned with writing on Wikipedia, I registered Gwern.net. Some geneticists had begun buzzing over GCTA and the early GWASes’ polygenic scores, which indicated that, candidate-genes notwithstanding, the genes were there to be found, and simple power analysis implied there were simply so many of them that one would need samples of tens of thousands–no, hundreds of thousands—of people to start finding them, which sounded daunting, but fortunately the super-exponential curve in sequencing costs ensured that those samples would become available in mere years, through things like something called ‘The UK BioBank’ (UKBB). (Told of this early on, I was skeptical: that seemed like an awful lot of faith in extrapolating a trend, and when did mega-projects like that ever work out?) Even more obscurely, some microbial geneticists noted that an odd protein associated with ‘CRISPR’ regions seemed to be part of a sort of bacterial immune system and could cut DNA effectively. Connectionism suddenly started working, and the nascent compute and NN trends did continue for the next decade, with AlexNet overnight changing computer vision, after which NNs began rapidly expanding and colonizing adjacent fields (despite perennial predictions that they would reach their limits Real Soon Now), with Transformers+pretraining recently claiming the scalp of the great holdout, natural language processing—nous sommes tous connexionnistes. At the same time, Wikileaks reached its high-water mark, helping inspire Edward Snowden, Bitcoin was gaining traction (I would hear of it and start looking into it in late 2010), and Ross Ulbricht was making Silk Road 1 (which he would launch in January 2011). In VR, Valve had returned to tinkering with it, and a young Palmer Luckey had begun to play with using smartphone screens as cheap high-speed high-res small displays for headsets. As dominant as mobile was, 2010 was also close to the peak (eg. the launch of Instagram): a mobile strategy could now be taken for granted, and infrastructure & practices had begun to catch up, so the gold rush was over and attention could refocus elsewhere.
Progression of interests: DNB → QS + IQ → statistics + meta-analysis + Replication Crisis → Bitcoin + darknet markets → behavioral genetics → decision theory → DL+DRL.
To the extent there is any consistent thread through my writing, it is an interest in optimization, which in humans is limited primarily by intelligence. New discoveries, improvements, and changes must come from somewhere; no matter how you organize any number of chimpanzees, they will not invent the atomic bomb. Thus my interest in Dual n-Back (DNB), Quantified Self (QS), and Spaced Repetition Systems (SRS) in 2010: these seemed promising routes to improvement. Of these, the first was a complete and unmitigated failure, the second was somewhat useful, and the third is highly useful in relatively narrow domains (although experiments in extending & integrating it are worthwhile).
I’d come across in Wired the first mention of the study that put dual n-back on the map, et al 2008 and was intrigued. While I knew the history of IQ-boosting interventions was dismal, to say the least, Jaeggi seemed to be quite rigorous, had a good story about WM being the bottleneck consistent with what I knew from my cognitive psychology courses and reading, and it quickly attracted some followup studies which seemed to replicate it. I began n-backing, and discussing the latest variants and research. The repetition in discussions prompted me to start putting together a DNB FAQ, which was unsuitable for Wikipedia, so I hosted it on my shell account on code.haskell.org
for a while, until I wanted to write a few more pages and decided it was time to stop abusing their generosity and set up my own website, Gwern.net, using this new static website generator called Hakyll. I didn’t know what I wanted to do, but I could at least write about anything I found interesting on my own website without worrying about deletionists or whether it was appropriate for LessWrong, and it would be good for hosting the occasional PDF too.
While n-backing, I began reading about the Replication Crisis and became increasingly concerned, particularly when Moody criticized DNB on several methodological grounds, arguing that the IQ gains might be hollow and driven by the test being sped up (and thus like playing DNB) or by motivational effects (because the control group did nothing). I began paying closer attention to studies, null results began to come out from Jaeggi-unaffiliated labs, and I began teaching myself R to analyze my self-experiments & meta-analyze the DNB studies (because no one else was doing it).
What I found was ugly: a thorough canvassing of the literature turned up plenty of null results, researchers would tell me about the difficulties in getting their nulls published, such as how peer reviewers would tell them they must have messed up their experiment because “we know n-back works”, and I could see even before running my first meta-analysis that studies with passive control groups got much larger effects than the better studies run with active control groups. Worse, some methodologists finally caught up and ran the SEMs on full-scale IQ tests instead of single matrix subtests (which is what you are always supposed to do to confirm measurement invariance, but in psychology, measurement & psychometrics are honored primarily in the breach); the gain, such as it was, wasn’t even on the latent factor of intelligence. Meanwhile, Jaeggi et al began publishing about complex moderation effects from personality, and ultimately suggesting in their rival meta-analysis that passive vs active was simply because of country-level differences—because I guess brains don’t work in the USA the way they do everywhere else. They (Jaeggi had since earned tenure) conceded the debate largely by simply no longer running DNB studies and shifting focus to specialized populations like children with ADHD and other flavors of working memory training, although by this point I had long since moved on and I can’t say what happened to all the dramatis personae.
It was, in short, a prototypical case of the Replication Crisis: a convenient environmental “One Weird Trick” intervention, with extremely low prior probability, which made no quantitative predictions, supported by a weak methodology which could manufacture positive results and propped up by selective citation, systemic pressure towards publication bias, researcher allegiance & commercial conflicts of interest, which theory was never definitively disproven, but lost popularity and sort of faded away. When I later reread Meehl, I ruefully recognized it all.
Inspired by Seth Roberts, I began running personal self-experiments, mostly on nootropics. I think this is an underappreciated way of learning statistics, as it renders concrete all sorts of abstruse issues: blocking vs randomization, blinding, power analysis, normality, missingness, time-series & auto-correlation, carryover, informative priors & meta-analysis, subjective Bayesian decision theory—all of these arise naturally in considering, planning, carrying out, and analyzing a self-experiment. By the end, I had a nice workflow: obtain a hypothesis from the QS community or personal observation; find or make a meta-analysis about it; consider costs and benefits to do a power analysis for Value of Information; run a blinded randomized self-experiment with blocks to estimate the true personal causal effect purged of honeymoon or expectancy effects; run the analysis at checkpoints for futility/low VoI; and write it up.
The first breakthrough was blinding: having been burned by DNB’s motivational expectancy effects, I became concerned about that rendering all QS results invalid. Blinding is simple enough if you are working with other people, but involving anyone else is a great burden for a self-experimenter, which is one reason no one in QS ever ran blinded self-experiments. After some thought, I realized self-blinding is quite easy; all that is necessary is to invisibly mark containers or pills in some way, randomize, and then one can infer after data collection which was used. For example, one could color two pills by experimental/placebo, shake them in a bag, take one with one’s eyes closed, and look at the remaining pill after recording data, or one could use identical-looking pills but in two separate containers, marking the inside or bottom of one, and again looking afterwards. (Self-blinding is so simple I refuse to believe I invented it, but I have yet to come across any prior art.) This gave me the confidence to go forward with my self-experiments, and I think I had some interesting results, some of which are expected (red-tinting software affecting sleep schedule), have been supported by subsequent research (the null effects of LSD microdosing1), some which are plausible but not yet confirmed (vitamin D damaging sleep when taken at night), and some which are simply mysterious (the strikingly harmful effects of potassium but also apparently of magnesium?). Ironically, I’ve gradually become convinced that self-blinding is not that important because the majority of bias in self-experiments comes from selective data reporting & non-randomization—people fool themselves by attributing the good days to the new nootropic (omitting all the average or blah days), and good days cause one to do optional things (like take nootropics).
I wound down my QS & nootropics activities for a few reasons. First, I became more concerned about the measurement issues: I am interested in daily functioning and ‘productivity’, but how on earth does one meaningfully quantify that? What are the “energies of men”, as William James put it? Almost all models look for an average treatment effect, but given the sheer number of nulls and introspecting about what ‘productive’ days felt like, it seems like expecting a large increase in the average (treating it as a latent variable in being spread across many measured variables) is entirely missing the value of productivity. It’s not that one gets a lot done across every variable, but one gets done the important things. A day in which one tidies up, sends a lot of emails, goes to the gym, walks the dog, may be worse than useless, while an extraordinary day might entail 12 hours programming without so much as changing out of one’s pyjamas. Productivity, it seems, might function more like ‘mana’ in an RPG than more familiar constructs like IQ, but unfortunately, statistical models which infer something like a total sum or index across many variables are rare; I’ve made little progress on modeling QS experiments which might increase total ‘mana’. But if you can’t measure something correctly, there is no point in trying to experiment on it; my Zeo did not measure sleep anywhere close to perfectly, but I lost faith that measuring a few things like emails or my daily 1–5 self-ratings could give me meaningful results rather than constant false negatives due to measurement error. Secondly, nootropics seem to run into diminishing returns quickly. As I see it, all nootropics work in one of 4 ways:
-
stimulant
-
anxiolytic
-
nutritional deficiency fix
-
it doesn’t
You don’t need to run through too many stimulants or anxiolytics to find one that works for you, deficiencies are deeply idiosyncratic and hard to predict (who would think that iron supplementation could cure pica or that vegetarians seem to benefit from creatine?), and past that, you are just wasting money (or worse). The low-hanging fruit has generally been plucked already—yeah, iodine is great… if you lived a century, in a deficient region, but unfortunately, as a Western adult it probably does zilch for you. (Likewise multivitamins.) I lost interest as it looked increasingly likely that I wasn’t going to find a new nootropic as useful to me as melatonin, nicotine, or modafinil.
Why were there so few silver bullets? Why did DNB fail (as well as every other intelligence intervention), why was the Flynn effect hollow, why were good nootropics so hard to find, why was the base rate of correct psychological hypotheses (as Ioannidis would put it) so low? The best answer seemed to be “Algernon’s law”: as a highly polygenic fitness-relevant trait, human intelligence has already been reasonably well optimized, is not particularly maladapted to the current environment (unlike personality traits or motivation or caloric expenditure), and thus has no useful ‘hacks’ or ‘overrides’. Intelligence is highly valuable to increase, and certainly could be increased—just marry a smart person—but there are no simple easy ways to do so, particularly for adults. Gains would have to come from fixing many small problems, but that is the sort of thing that drugs and environmental changes are worst at. (It is telling that after decades of searches, there is still not a single known mutation, rare or common, which increases intelligence by even a few IQ points, aside from possibly that ultra-rare one which also makes you go blind. In contrast, plenty of large-effect mutations are known for more neutral traits like height.)
Going further, I wondered whether the disasters in nutrition & exercise research, so parallel to sociology & psychology, were merely cherry-picked. Yes, everyone knows ‘correlation ≠ causation’, but is this a serious objection, or is it a nitpicking one of the sort which only occasionally matters and is abused as a universal excuse? I found that first, the presence of a correlation is a foregone conclusion and hence completely uninformative: because “everything is correlated”, finding a correlation between two variables is useless, since of course you’ll find one if you look, so it’d be surprising only if you were didn’t detect one (and that might say more about your statistical power than the real world). Predicting their direction is not impressive either, since that’s just a coin-flip, and there is a positive manifold (from intelligence, SES, and health, if nothing else), making it even easier. Second, the simplest way to answer this question is to look at randomized experiments and compare—presumably only the best and most promising correlations, supported by many datasets and surviving all standard ‘controls’, will progress to expensive randomized experiments, and the conditional probability of the randomized experiment agreeing with a good correlation is what we care about most, since we in practice we already ignore the more dubious ones. There are a few such papers cited in the Replication Crisis literature, but I found that (Cowen’s Law!) there were actually quite a few such reviews/meta-analyses, particularly by the UK NICE (which does underappreciated work). The results are not good: it is not rare, but common, for the randomized result to be seriously different from the prior correlation results, and this holds true whether we look at economics, sociology, medicine, advertising… When we look at the track record of social interventions, paying particular attention to interventions which have gone down the memory hole and lessons from the Replication Crisis (sorry, no one believes your 20-year-followup where only females under 13 at the time of intervention show statistically-significant benefits), it’s not much better. Interventions also tend to be self-limited by their temporary and focused nature: “everything is correlated” and outcomes are driven by powerful latent variables which are maintained by unchangeable individual genetics (“everything is heritable”) which are responsible for longitudinal stability in traits (perhaps through mechanisms like “the environment is genetic”) and long-lasting individual differences which are set early in life. This produces the “gloomy prospect” for QS, and for social engineering in general: there may be useful interventions, but they will be of little value on average—if the benefit is universal, then it will be small; if it is large and predictable, then it will be limited to the few with a particular disease; otherwise, it will be unpredictably idiosyncratic so those who need it will not know it. Thus, the metallic laws: the larger the change you expect, the less likely it is; the low-hanging fruit, having already been plucked, will not be tested; and the more rigorously you test the leftovers, the smaller the final net effects will be.
Spaced repetition was another find in Wired, and made me feel rather dim. We had covered Ebbinghaus and the spacing effect in class, but it had never occurred to me that it could be useful, especially with a computer. (“How extremely stupid of me not to have thought of that.”) I immediately began using the Mnemosyne SRS for English vocabulary (sourcing new words from A Word A Day) and classwork. I stopped the English vocab when I realized that my vocabulary was already so large that if I needed SRS to memorize a word, I was better off never using that word because it was a bar to communication, and that my prose already used too many vocab words, but I was so impressed that I kept using it. (It is magical to use an SRS for a few weeks, doing boring flashcard reviews with some oddly fussy frills, and then a flashcard pops up and one realizes that one just remembers it despite having only seen the card 4 or 5 times and nothing otherwise.) It was particularly handy for learning French, but I also value it for storing quotes and poetry, and for helping correct persistent errors. For a LessWrong contest, I read entirely too many papers on spaced repetition and wrote an overview of the topic, which has been useful over the years as interest in SRS has steadily grown.
What drew in my interest next? A regular on IRC, one kiba, kept talking about something called Bitcoin, touting it to us in November 2010 and on. I was curious to see this atavistic resurgence of cypherpunk ideas—a decentralized uncensorable e-cash, really? It reminded me of being a kid reading the Jargon File and the Cyphernomicon and Phrack and &TOTSE. Bitcoin, surprisingly enough, worked (which was more than one could say of other cypherpunk infrastructure like remailers), and the core idea, while definitely a bit perverse, was a surprising method of squaring the circle; I couldn’t think of how it ought to fail in theory, and it was working in practice. In retrospect, being introduced to Bitcoin so early, before it had become controversial or politically-charged, was enormously lucky: you could waste a lifetime looking into longshots without hitting a single Bitcoin, and here Bitcoin walked right up to me unbidden, and all I had to do was simply evaluate it on its merits & not reflexively write it off. Still, some cute ideas and a working network hardly justified wild claims about it eating world currencies, until I read in Gawker about a Tor drug market which was not a scam (like most Tor drug shops were) and used only Bitcoin, called “Silk Road”, straight out of the pages of the Cyphernomicon: it used escrow, feedback, and had many buyers & sellers already. That got my attention, but subsequent coverage of SR1 drove me nuts with how incompetent and ignorant it was. Kiba gave me my first bitcoins to write an SR1 discussion and tutorial for his Bitcoin Weekly online magazine, which I duly did (ordering, of course, some Adderall for my QS self-experiments) The SR1 tutorial was my first page to ever go viral. I liked that. People kept using it as a guide to getting started on SR1, because there is a great gap between a tool existing and everyone using it, and good documentation is as underestimated as open datasets.
Many criticisms of Bitcoin, including those from cryptographic or economic experts, were not even wrong, but showed they hadn’t understood the ‘Proof of Work’ mechanism behind Bitcoin. This made me eager to get Bitcoin because when someone like P— K— or C— S— made really dumb criticisms and revealed that they claimed Bitcoin would fail not because the actual mechanisms would break down but because they wanted Bitcoin to fail so governments can more easily manipulate the money supply, such wishful thinking, from otherwise decent thinkers implied that Bitcoin was undervalued. (Bitcoin’s price was either far too low or far too high, and reversed stupidity is intelligence when dealing with a binary like that.) The persistence of such lousy critics was interesting, as it meant that PoW fell into some sort of intellectual blind spot; as I noted, there was no reason Bitcoin could not have been invented decades prior, and unlike most successful technologies or startups where there is a long trail of corpses, Bitcoin came out of the blue—in terms of intellectual impact, “having had no predecessor to imitate, he had no successor capable of imitating him”. What was the key idea of Bitcoin, its biggest advantage, and why did people find it so hard to understand? I explained it to everyone in my May 2011 essay, “Bitcoin Is Worse Is Better”, which also went viral; I am sometimes asked, all these crazy years later, if I have changed my views on it. I have not. I nailed the core of Bitcoin in 2011, and there is naught to add nor take away.
Meanwhile, Bitcoin kept growing from a tiny seed to, years later, something even my grandmother had heard of. (She heard on the news that Bitcoin was bankrupt and asked if I was OK; I assured her I had had nothing on MtGox and would be fine.) I did not benefit as much financially as I should have, because of, shall we say, ‘liquidity constraints’, but I can hardly complain—involvement would have been worthwhile even without any remuneration, just to watch it develop and see how wrong everyone can be. One regret was the case of Craig Wright, which investigation turned out to be a large waste of my time and to backfire; I regret not working with Andy Greenberg to write an article, nor any of the possible findings we missed, but that when we learned Gawker was working on a Wright piece, that we assumed the worst, that it was a trivial blog post which would scoop our months of work, and so we jumped the gun by publishing an old draft article instead of contacting them to find out what they had. We had not remotely finished looking into Craig Wright, and it turned out that Gawker had gotten even further than we had, if anything, and if both groups had pooled their research, we would’ve had a lot more time before publishing, and more time to look into the many red flags and unchecked details. As it was, all we got for our trouble was to be dragged through the mud by Wright believers, who were furious at us monsters trying to unmask a great man, and by Wright critics, who were furious we were morons assisting a conman making millions and too dumb to see all the red flags in our own reporting. More positively, the revival of cypherpunk ideas in Bitcoin and Ethereum was really something to see, and gave rise to all sorts of crazy new ideas, as well as creating a boom in researching exotic new cryptography, particularly zero-knowledge proofs. There are two important lessons there. The first is the extent to which progress can be held back by a single missing piece: the basic problem with almost everything in Cyphernomicon is that they require a viable e-cash to work, and with the failure of Chaumian e-cash schemes, there was none; the ideas for things like black markets for drugs were correct, but useless without that one missing piece. The second is the power of incentives: the amount of money in cryptocurrency is, all things considered, really not that large compared to the rest of the economy or things like pet food sales, and yet, it is approximately ∞% more money than previously went into writing cypherpunk software or into cryptography, and while a shocking amount went into blatant scams, Ponzis, hacks, bezzles, and every other kind of fraud, an awful lot of software got written and an awful lot of new cryptography suddenly showed up or was matured into products possibly decades faster than one would’ve expected.
Media coverage of SR1 did not improve, honorable exceptions like Andy Greenberg or Eileen Ormsby aside, and I kept tracking the topic, particularly recording arrests because I was intensely curious how safe using DNMs was. SR1 worked so well that we became complacent, and the fall of SR1 was traumatic—all that information and forum posts lost Almost all DNM researchers selfishly guarded their crawls (sometimes to cover up bullshit), guaranteeing minimal use, and datasets were clearly a bottleneck in DNM research & history, particularly given the extraordinary turnover in DNMs afterwards (not helped by the FBI announcing just how much Ross Ulbricht had made). This led me to my most extensive archiving efforts ever, crawling every extent English DNM & forum on a weekly or more frequent basis. This was technically tricky and exhausting, especially as I moderated /r/DarkNetMarkets, and tried to track the fallout from SR1 & all DNM-related arrests (due not just to the SR1 raid or lax security at successors, but simply growing volume and time lags in learning of cases, as many had been kept secret or were tied up in legal proceedings).
As amusing as it was to be interviewed regularly by journalists, or to see DNMs pop up & be hacked almost immediately (I did my part when I pointed out to Black Goblin Market that they had sent my user registration email over the clearnet, deanonymizing their server in a German datacenter, and should probably cut their losses), DNM dynamics disappointed me. A few DNMs like The Marketplace tried to improve, but buyers proved actively hostile to genuine multisig, and despite all the documented SR1 arrests, PGP use in providing personal information seemed to, if anything, decline. In the end, SR1 proved not to be a prototype, soon obsoleted in favor of innovations like multisig and truly decentralized markets, but in some respects the peak of the centralized DNM, relatively efficient & well-run and with features rarely seen on successors like hedging the Bitcoin exchange rate. The carding fraud community moved in on DNMs, and the worst of the synthetic opiates like carfentanil soon appeared. In 2020, DNMs are little different than when they sprang fully-formed out of Ross Ulbricht’s forehead in January 2011. Users, it seems, are lazy; they are really lazy; even if they are doing crimes, they would rather risk going to jail than spend 20 minutes figuring out how to use PGP, and they would rather accept a large risk every month of losing hundreds or thousands of dollars rather than spend time figuring out multisig. By 2015, I had grown weary; the final straw was when ICE, absurdly, subpoenaed Reddit for my account information. (I could have told them that the information they were interested in had never been sent to me, and if it had, it would almost certainly have been lies or a frame, not that they ever bothered to ask me.) So, I shut down my spiders, neatly organized and compressed them, and released them as a single public archive. I had hoped that by releasing so many datasets, it would set an example, but while >46 publications use my data as of January 2020, few have seen fit to release their own. The eventual success of my archives reinforced my view that public permission-less datasets are often a bottleneck to research: you cannot guarantee that people will use your dataset, but you can guarantee that they won’t use it. Hardly any of the people who used my data ever so much as contacted me (so I do annual deep dives in Google Scholar to try to figure out who’s using my stuff), and the number of uses stands in stark contrast to Nicolas Christin & Kyle Soska’s DNM datasets, which were released either censored to the point of uselessness or using a highly onerous data archive service called IMPACT. And that was that. The FBI would later pay me some visits, but I was long done with the DNMs and had moved on.
For genetics was experiencing its own revival. Much more dramatically than my DNM archives, human genetics was demonstrating the power of open data as an obscure project called the UK BioBank (UKBB) came online, with n = 500k SNP genotypes & rich phenotype data; UKBB was only a small fraction of global genome data (sharded across a million silos and little emperors), and much smaller than 23andMe (disinclined to do anything controversial or which might impede drug commercialization), but it differed in one all-important respect: they made it easy for researchers to get all the data. The result is that—unlike 23andMe, All Of Us, Million Veteran Program, or the entire nation of China—papers using UKBB show up literally every day on BioRxiv alone, and it would be hard to find human genetics research which hasn’t benefited, one way or another, from UKBB. (How did UKBB happen? I don’t know, but there must be many unsung heroes behind it.) Emphasizing this even more was the explosion of genetic correlation results. I read much of the human genetic correlation literature, and it went from a few genetic correlation papers a year, to hundreds. Why? Simple: previously, genetic correlations required individual personal data, as you either needed the data from twin pairs to calculate cross-twin correlations or run the SEM, or you needed the raw SNP genotypes to use GCTA; twin registries keep their data close to their chest, and everyone with SNP data guards those even more jealously (aside from UKBB). Like a dog in the manger, if the owners of the necessary datasets couldn’t get around to publishing them, no one would be allowed to. But then a methodological breakthrough happened: LD Score Regression was released with a convenient software implementation, and LDSC worked around the broken system by only requiring the PGSes, not the raw data. Now a genetic correlation could be computed by anyone, for any pair of PGSes, and many PGSes had already been released as a concession to openness. An explosion of reported genetic correlations followed, to the point where I had to stop compiling them for the Wikipedia entry because it was futile when every other paper might run LDSC on 50 traits.
The predictions of some behavioral geneticists & human geneticists (particularly those with animal genetics backgrounds) came true: increasing sample sizes did deliver successful GWASes, and the ‘missing heritability’ problem was a non-problem. I had remained agnostic on the question of IQ and genetics, because while IQ is too empirically successful to be doubted (not that that stops many), the genetics have always been fiercely opposed; on looking into the question, I had decided that GWASes would be the critical test, and in particular, sibling comparisons would be the gold standard—as R. A. Fisher pointed out, siblings inherit randomized genes from their parents and also grow up in shared environments, excluding all possibilities of confounding or reverse causation or population structure: if a PGS works between-siblings, it must be tapping into causal genes.2 The critics had always concentrated their fire on IQ, as, supposedly, the most biased, racist, and rotten keystone in the overall architecture of behavioral genetics, and evinced utter certitude; as went IQ, so went their arguments. I decided years ago that a successful sibling test of an IQ GWAS with genome-wide statistically-significant hits (not candidate-genes) is what it would take to change my mind.
The key result was et al 2013 , the first truly successful IQ GWAS. et al 2013 found GWAS hits; further, it found between-sibling differences. (This sibling test would be replicated easily a dozen times by 2020.) Reading it was a revelation. The debate was over: behavioral genetics was right, and the critics were wrong. Kamin, Gould, Lewontin, Shalizi, the whole sorry pack—annihilated. IQ was indeed highly heritable, polygenic, and GWASes would only get better for it, and for all the easier traits as well. ( “To see the gods dispelled in mid-air and dissolve like clouds is one of the great human experiences. It is not as if they had gone over the horizon to disappear for a time; nor as if they had been overcome by other gods of greater power and profounder knowledge. It is simply that they came to nothing.”) Among other implications, embryo selection was now proven feasible (embryos are, after all, just siblings), and suddenly far-distant future speculations like iterated embryo selection (IES) no longer seemed to rest on such a rickety tower of assumptions. This was concerning. Also concerning was the willful blindness of many, including respectable geneticists and scientists, who happily made up arguments about polygenicity, invented genetic correlations, conflated hits with PGS with SNP heritability with heritability, claimed GWASes wouldn’t replicate, ignored all inconvenient animal examples, and simply dismissed out of hand all possibilities as minor without so much as a single number mentioned; in short, if there was any way to be confused or one could invent any possible obstacle, then that immediately became a fatal objection.3 2014 finally provided an adult perspective on embryo selection possibilities and was good as far as it went in a few pages, but I felt neglected a lot of practical questions and didn’t go beyond the simplest possible kind of embryo selection.
Since no one was willing to answer my questions, I began answering them myself. I first began by replicating 2014’s results with the simplest model of embryo selection, and began working in the various costs and attrition in the IVF pipeline, to create a realistic answer. I then began looking at the scaling: which is more important, PGS or number of embryos? How much can either be boosted in the foreseeable future? Surprising, n is more important than PGS, despite PGS being what everyone always debated, and I detoured a bit into order statistics, since the importance of ‘massive embryo selection’ was underrated. Diminishing returns do set in, but there are two major improvements: multi-stage selection, where one selects at multiple stages in the process, which turns out to be absurdly more effective, and selecting on an index of multiple traits using the countless PGSes now available, which is substantially more efficient and also addresses the bugaboo of negative genetic correlations—selecting on a trait like intelligence will not ‘backfire’ because when you look at human phenotypic and genotypic correlations as a whole, almost every good trait is correlated with (not merely independent of) other good traits, and likewise for bad traits, and this supercharges selection. There were a number of other interesting avenues, but I largely answered my question: embryo selection is certainly possible, will be soon (and has since) been done, is profitable already, albeit modestly so, genetic editing like CRISPR is probably drastically overrated barring breakthroughs in doing hundreds or thousands of edits safely, but there are multiple pathways to far more effective and thus disruptive changes in the 2020s–2030s through massive embryo selection or IES or genome synthesis (with a few wild cards like gamete or chromosome selection), particularly as gains accumulate over generations. Modeling IES or genome synthesis is almost unnecessary because the potential gains are so large. (There are still some interesting questions in constrained optimization and modeling breeding programs with the existing haplotypes/LD, but I’m not sure they’re important to know at this point.)
I kept an eye on deep learning the entire time post-AlexNet, and was perturbed by how DL just kept on growing in capabilities and marching through fields, and in particular, how its strengths were in the areas that had always historically bedeviled AI the most and how they kept scaling as model sizes improved—improve as models with millions of parameters were, people were already talking about training NNs with as many as a billion parameters. Crazy talk? One couldn’t write it off so easily. Back in 2009 or so, I had spent a lot of time reading about Lisp machines and AI in the 1980s, going through old journals and news articles to improve the Wikipedia article on Lisp machines, and I was amazed by the Lisp machine OSes & software, so superior to Linux et al, but also doing a lot of eye-rolling at the expert systems and robots which passed for AI back then; in following deep learning, I was struck by how it was the reverse, GPU were a nightmare to program for and the software ecosystem was almost actively malicious in sabotaging productivity, but the resulting AIs were uncannily good and excelled at perceptual tasks. Gradually, I became convinced that DL was here to stay, and offered a potential path to AGI: not that anyone was going to throw a 2016-style char-RNN at a million GPUs and get an AGI, of course, but that there was now a nontrivial possibility that further tweaks to DL-style architectures of simple differentiable units combined with DRL would keep on scaling to human-level capabilities across the board. (Have you noticed how no one uses the word “transhumanist” anymore? Because we’re all transhumanists now.) There was no equivalent of et al 2013 for me, just years of reading Arxiv and following the trends, reinforced by occasional case-studies like AlphaGo (let’s take a moment to remember how amazing it was that between October and May, the state of computer Go went from ‘perhaps a MCTS variant will defeat a pro in a few years, and then maybe the world champ in a decade or two’ to ‘untouchably superhuman’; technology and computers do not follow human timelines or scaling, and 9 GPUs can train a NN in a month).
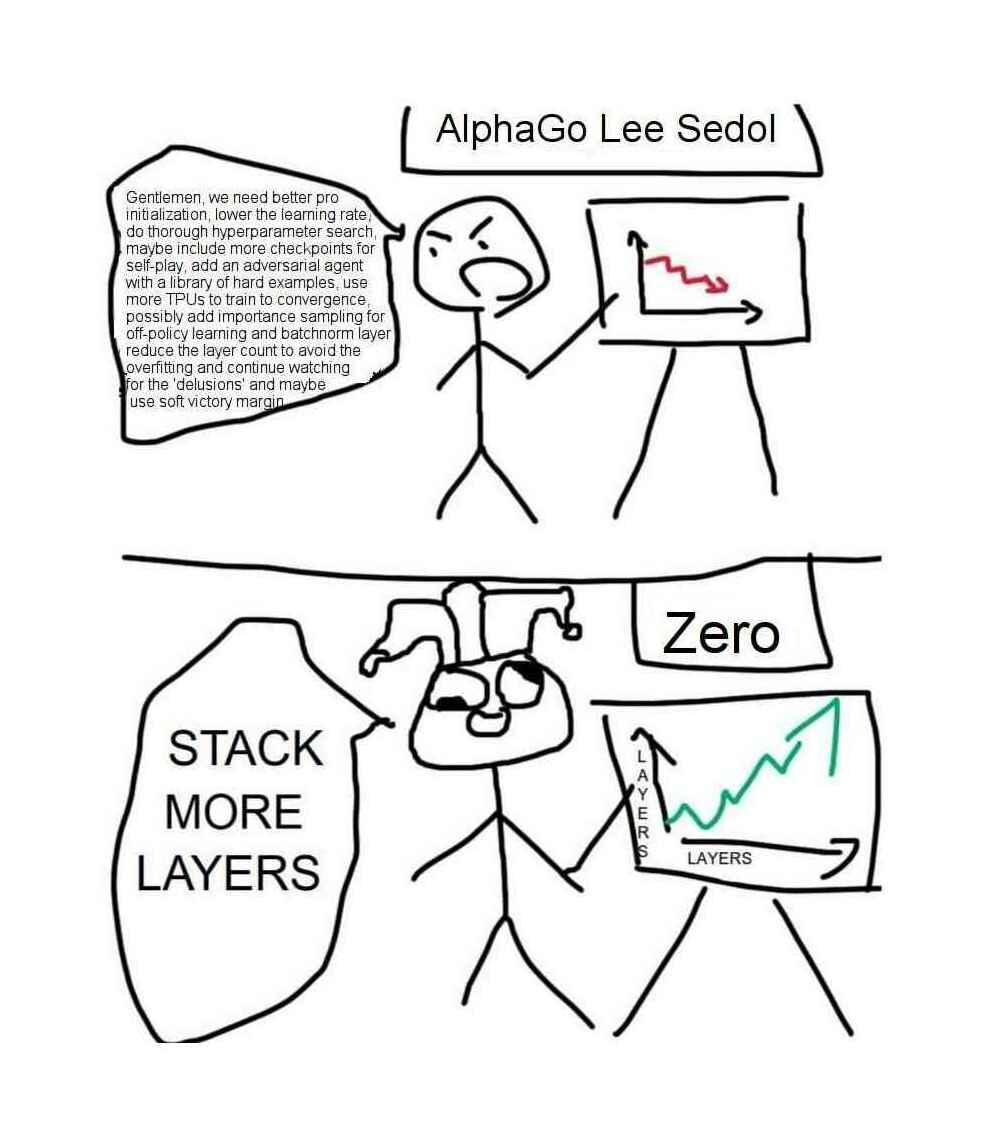
The “bitter lesson” encapsulates the long-term trends observed in DL, from use in a few limited areas, often on pre/post-processed data or as part of more conventional machine learning workflows, heavy on symbolic techniques, to ever-larger (and often simpler) neural net models. MuZero is perhaps the most striking 2019 example of the bitter lesson, as AlphaZero had previously leapt past all previous symbolic Go (and chess, and shogi) players using a hybrid of neural nets (for near-superhuman intuition) and then symbolic tree search to do planning over thousands or millions of scenarios in an exact simulation of the environment, but then MuZero threw all that away in favor of just doing a lot of training of an RNN, which does… whatever it is an RNN does internally, with no need for explicit planning or even an environment simulator—and quite aside from beating AlphaZero, also applies directly to playing regular ALE video games. (To update Frederick Jelinek’s quip, “every time I fire a machine learning researcher & replace him with a TPU pod, the performance of our Go-playing system goes up.”)
An open question: why was I and everyone else wrong to ignore connectionism when things have played out much as Schmidhuber and 1998 and a few others predicted? Were we wrong, or just unlucky? What was, ex ante, the right way to think about this, even back in the 1990s or 1960s? I am usually pretty good at bullet-biting on graphs of trends, but I can’t remember any performance graphs for connectionism; what graph should I have believed, or if it didn’t exist, why not?
If there had always been a loud noisy contingent, perhaps a minority like a quarter of ML researchers, who watched GPU progress with avid interest and repeatedly sketched out the possibilities of scaling in 2010–2020, and eagerly leapt on it as soon as resources permitted and advocated for ever larger investments, one could write this off as a natural example of a breakthrough: surprises happen, that’s why we do research, to find out what we don’t know. But instead, there were perhaps a handful who truly expected it, even they seemed surprised by how it happened; and no matter how much progress was made, the naysayers never changed their tune (only their goalposts). (The most striking example was offered midway in 2020 with GPT-3.) A systematic, comprehensive, field-wide failure of prediction & updating like that demands explanation.
The best explanation I’ve come up with so far is working backwards from the excuses, that this may be yet another manifestation of the human bias against reductionism & prediction: “it’s just memorization”, “it’s just interpolation”, “it’s just pattern-matching”, etc, perhaps accompanied by an example problem that DL can’t (yet) solve which supposedly demonstrates the profound gulf between ‘just X’ and real intelligence. It is, in other words, fundamentally an anti-reductionist argument from incredulity: “I simply cannot believe that intelligence like a human brain has could possibly be made up of a lot of small parts”. (That the human brain is also made of small parts is irrelevant to them because one can always appeal to the mysterious and ineffable complexity of biological neurons, with all their neurotransmitters and synapses and whatnot, so the brain feels adequately complex and part-less.) If so, deep learning merely joins the long pantheon of deeply unpopular reductionist theories throughout intellectual history: Atomism, materialism, atheism, gradualism, capitalism, evolution, germ theory, elan vital & ‘organic’ matter, polygenicity, Boolean logic, Monte Carlo/simulation methods… All of these inspired enormous resistance and deep visceral hatred, despite proving to be true or more useful. Humans seem cognitively hardwired to believe that everything is made up of a few fundamental units which are ontologically basic and not reducible to (extremely) large numbers of small uniform discrete objects.4 If the outputs are beautifully complex and intricate, the inputs must also be that way: things can’t be made of a few kinds of atoms, they must instead be made of a different type for every object like a plenum of bone-particles for bones or water-particles for water; you can’t be a physical brain, your mind must instead be a single indivisible ontologically-basic ‘soul’ existing on an immaterial plane; economies can’t be best run as many independent agents independently transacting, it would be much better for the philosopher-king & his cadres to plan out everything; etc. Similarly, intelligence can’t just be a relatively few basic units replicated trillions of times & trained with brute force, it must be thousands of intricate units performing arithmetic, logic, memory, pattern-matching, program synthesis, analogizing, reinforcement learning, all in different ways and carefully organized with enormous expertise… But for all the biases, reductionism still ultimately wins out. So why didn’t it win sooner?
What went wrong? There is a Catch-22 here: with the right techniques, impressive proof-of-concepts could have been done quite a few years ago on existing supercomputers and successful prototypes would have justified the investment, without waiting for commodity gaming GPUs; but the techniques could not be found without running many failed prototypes on those supercomputers in the first place! Only once the prerequisites fell to such low costs that near-zero funding sufficed to go through those countless iterations of failure, could the right techniques be found, and justify the creation of the necessary datasets, and further justify scaling up. Hence, the sudden deep learning renaissance—had we known what we were doing from the start, we would have simply seen a gradual increase in capabilities from the 1980s.
The flip side of the bitter lesson is the sweet shortcut: as long as you have weak compute and small data, it’s always easy for the researcher to smuggle in prior knowledge/bias to gain greater performance. That this will be disproportionately true of the architectures which scale the worst will be invisible, because it is impossible to scale any superior approach at that time. Appealing to future compute and speculating about how “brain-equivalent computing power” arriving by 2010 or 2030 will enable AI sounds more like wishful thinking than good science. A connectionist might scoff at this skepticism, but they have no compelling arguments: the human brain may be an existence proof, but most connectionist work is a caricature of the baroque complexity of neurobiology, and besides, planes do not flap their wings nor do submarines swim. How would they prove any of this? They can’t, until it’s too late and everyone has retired.
TODO: candidate-gene debacle
Thus, there is an epistemic trap. The very fact that connectionism is so general and scales to the best possible solutions means that it performs the worst early on in R&D and compute trends, and is outcompeted by its smaller (but more limited) competitors; because of this competition, it is starved of research, further ensuring that it looks useless; with a track record of being useless, the steadily decreasing required investments don’t make any difference because no one is taking seriously any projections; until finally, a hardware overhang accumulates to the point that it is doomed to success, when 1 GPU is enough to iterate and set SOTAs, breaking the equilibrium by providing undeniable hard results.
This trap is intrinsic to the approach. There is no alternate history where connectionism somehow wins the day in the 1970s and all this DL progress happens decades ahead of schedule. If Minsky hadn’t pointed out the problems with perceptrons, someone else would have; if someone had imported convolutions in the 1970s rather than LeCun in 1990, it would have sped things up only a little; if backpropagation had been introduced decades earlier, as early as imaginable, perhaps in the 1950s with the development of dynamic programming, that too would have made little difference because there would be little one could backprop over (and residual networks were introduced in the 1980s decades before they were reinvented in 2015, to no effect); and so on. The history of connectionism is not one of being limited by ideas—everyone has tons of ideas, great ideas, just ask Schmidhuber for a basket as a party favor!—but one of results; somewhat like behavioral & population genetics, all of these great ideas fell through a portal from the future, dropping in on savages lacking the prerequisites to sort rubbish from revolution. The compute was not available, and humans just aren’t smart enough to either invent everything required without painful trial-and-error or prove beyond a doubt their efficacy without needing to run them.
GANs in 2014 caught my attention because I knew the ultra-crude 64px grayscale faces would improve constantly, and in a few years GANs would be generating high-resolution color images of ImageNet. I wasn’t too interested in ImageNet per se, but if char-RNNs could do Shakespeare and GANs could do ImageNet, they could do other things… like anime and poetry. (Why anime and poetry? To épater la bourgeoisie, of course!) However, there was no anime dataset equivalent to ImageNet, and as I knew from my DNM archives, datasets are often a bottleneck, so after looking around for a while, I began laying the groundwork for what would become Danbooru2017. Karpathy also famously put char-RNNs on the map, and I began experimenting with poetry generation. Anime didn’t work well with any GAN I tried, and I had to put it aside. I knew a useful GAN would come along, and when it did, Danbooru2017 would be ready—the pattern with deep learning is that it doesn’t work at all, and one layers on complicated hand-engineered architectures to eek out some performance, until someone finds a relatively simple approach which scales and then one can simply throw GPUs & data at the problem. What I’ve learned about NNs is that they scale almost indefinitely; we don’t have any idea how to train NNs well, and they are grossly overparameterized, with almost all of a NN’s parameters being unnecessary; breakthroughs are made by trial-and-error to a degree scrubbed from research papers, and ‘algorithmic’ progress is primarily due to compute enabling enormous amounts of experiments; theory is almost useless in guiding NN design, with papers actively misleading the reader about this (eg. the ResNet paper); even subtle details of initialization or training can have shockingly large implications on performance—a NN which seems to be working fine may in fact be badly broken but still work OK because “NNs want to work”; “NNs are lazy” and will solve any given task in the laziest possible way unless the task is hard (eg. they can do disentanglement or generalization or reasoning just fine, but only if we actually force them to solve those tasks and not something easier).
Finally, in 2017, ProGAN showed that anime faces were almost doable, and then with StyleGAN’s release in 2019, I gave it a second shot (expecting a modest improvement over ProGAN, which is what was reported on photographic faces etc) and was shocked when almost overnight StyleGAN created better anime faces than ProGAN, and soon was generating shockingly-good faces. As a joke, I put up samples as a standalone website TWDNE, and then a million Chinese decided to pay a visit. 2019 also saw GPT-2, and if the char-RNN poetry was OK, the GPT-2 poetry samples, especially once I collaborated with Shawn Presser to use hundreds of TPUs to finetune GPT-2-1.5b (Google TRC paid for that and other projects), were fantastic. Overnight, the SOTA for both anime and poetry generation took huge leaps. I wrote extensive tutorials on both StyleGAN and GPT-2 to help other people use them, and I’m pleased that, like my SR1 tutorial, a great many people found them useful, filling the gap between ordinary people and a repo dumped on Github. (A followup project to use an experimental DRL approach to improve poetry/music quality further didn’t work out.)
I’d always spent time tweaking Gwern.net’s appearance and functionality over the years, but my disinclination to learn JS/CSS/HTML (a tech stack that manages to be even more miserable than the deep learning Python ecosystem) limited how far I could go. Said Achmiz volunteered, and redesigned it almost from the ground up 2018–2020, and is responsible for everything from the dropcaps to the collapsible sections to sidenotes to link annotations. (I can take credit for relatively few things, like the Haskell backends and writing annotations; I mostly just request features while trying to educate myself about typography & design.) What was a utilitarian static site is now almost a design showcase, and I can’t help but feel from watching traffic and mentions that the improvements have made Gwern.net more respectable and readers receptive, despite most of the contents remaining the same; synchronously, I began working on my appearance by getting LASIK, picking up weightlifting as a hobby, upgrading my wardrobe etc, and there too I can’t help but feel that people were judging by my appearance to an even greater extent that I (thought I had cynically) supposed. This reminds me of how AI risk developed in the 2010s; it went from a wingnut fringe concern to broad acceptance, as far as I can tell, starting in 2014 when Nick Bostrom’s Oxford University Pressed-published Superintelligence (which largely repackages the thought of Eliezer Yudkowsky and other Singularitarians for a general audience) became a NYT bestseller and then was endorsed by Elon Musk. What does Elon Musk know about AI risk? What does the NYT bestseller list know about anything? And yet, I noticed at the time the sea change in attitude from such high-status endorsements. Not, of course, that it helped Yudkowsky’s reputation, as newcomers to the topic promptly did their best to erase him and plant their flags by coining ever new neologisms to avoid using terms like “Friendly AI” (I lost track of them all, like “Beneficent AI” or “human-aligned AI” or “AI alignment” or “Provably Beneficial AI” or “AI For Good” or “AI safety”). A lesson there for pioneers expecting to at least get credit for being right: if you succeed in becoming a bridge to a better future, it may be because the ‘respectable’ ‘serious’ people knifed you in the back and walked across. (AI risk was also promptly hijacked by “what if the real AI risk is capitalism & not enough identity-politics” types, but it hasn’t been a total loss.)
I think it would have been a mistake to focus too much on design and appearance early on, however. There is no point in investing all that effort in tarting up a website which has nothing on it, and it makes sense only when you have a substantial corpus to upgrade. One early website design flaw I do regret is not putting an even greater emphasis on detailed citation and link archiving; in retrospect, I would have saved myself a lot of grief by mirroring all PDFs and external links as soon as I linked them. I thought my archiver daemon would be enough, but the constant cascade of linkrot, combined with the constant expansion of Gwern.net, made it impossible to keep up with linkrot manually, and by omitting basic citation metadata like titles & authors, I had a hard time dealing with links that escaped archiving before dying. Likewise, treating social media as a platform for drafting and publicizing is important for any writer to remember. Platforms are not your friend, because you are the complement they are trying to commoditize. A platform like Google+ or Twitter or Reddit can and will demote or delete you and your content, or disappear entirely, for trivial reasons. (Who can forget the New Yorker’s chilling account of watching young college-grad Reddit employees flippantly decide which subreddits to erase, crowing as they settled scores? Or how many subreddits were deleted in their entirety in various purges because of a coincidence in names, showing no employees had so much as looked at their front page? Google+, of course, was erased in its entirety; I had predicted a substantial chance of its death, but was still dismayed.) The probability may be small each year, but it adds up. In the next decade, I don’t know what website I use will go under or crazy: HN, Twitter, Reddit, WP, LW? But I will try to be ready and ensure that anything of value is archived, exported, or moved to Gwern.net.
Another underrated activity, along with creating datasets and methods, is providing fulltexts. “Users are lazy”, and if it isn’t in Google/Google Scholar, then it doesn’t exist for researchers either. I noticed on Wikipedia that while there were few overt signs that my articles were having any influence—who cites a Wikipedia article?—there were continuous suspicious coincidences. I would dig up a particularly recherché article or interview, scan or host it, quote it on WP, and years later, notice that some article would cite it; without any reference to me or WP. (“Oh ho, you just happened to dig it up independently in your doubtless thorough literature review, did you? A likely story!”) This is equally true for other topics; how many times have I dug up some paper or thesis from the 1950s and—mirabile dictu!—other researchers suddenly began to cite it in papers? It would be interesting to pull citation metadata from Semantic Scholar and cross-reference it with my revision history to look at the time-series and see how much my hosting increases total citation count once Google Scholar indexed it.
Overall, I think I have a good track record. My predictions (whether on IEM, Intrade, PredictionBook, or Good Judgment Project) are far above average, and I have taken many then-controversial positions where contemporary research or opinion has moved far closer to me than the critics, often when I was in a minute minority. Examples include Wikipedia’s editing crisis, anti-DNB, the Replication Crisis, Bitcoin, darknet markets, modafinil & nicotine, LSD microdosing, AI risk, behavioral genetics, embryo selection, and advertising harms. If I have not always been right from the start, I have at least been less wrong than most in updating faster than most (DNB, behavioral genetics, DL/DRL).
Media
Links
Genetics:
-
Everything Is Heritable:
-
“Recovery of [full height/BMI] trait heritability from whole genome sequence data”, et al 2019
-
“Genetic analysis identifies molecular systems and biological pathways associated with household income”, et al 2019a; “Genome-wide analysis identifies molecular systems and 149 genetic loci associated with income”, et al 2019b
-
“Comparing within. and between-family polygenic score prediction”, et al 2019
-
“Large-scale GWAS reveals insights into the genetic architecture of same-sex sexual behavior”, et al 2019
-
“Extreme inbreeding in a European ancestry sample from the contemporary UK population”, et al 2019
-
-
Engineering:
-
full genome synthesis of E. coli: 4 million base-pairs; “Technological challenges and milestones for writing genomes: Synthetic genomics requires improved technologies”, et al 2019
-
“Extensive Mammalian Germline Genome Engineering”, et al 2019; “Enabling large-scale genome editing by reducing DNA nicking”, et al 2019
-
“Functional Oocytes Derived from Granulosa Cells”, et al 2019; “Controlled modelling of human epiblast and amnion development using stem cells”, et al 2019
-
“Synthetic glycolate metabolism pathways stimulate crop growth and productivity in the field”, et al 2019
-
“Super-Mendelian inheritance mediated by CRISPR-Cas9 in the female mouse germline”, et al 2019
-
“Livestock 2.0—genome editing for fitter, healthier, and more productive farmed animals”, Tait-et al 2018
-
Recent Evolution:
-
“Highly Heritable and Functionally Relevant Breed Differences in Dog Behavior”, Macet al 2019; “Absolute brain size predicts dog breed differences in executive function”, et al 2019; “Breed differences of heritable behavior traits in cats”, et al 2019
-
“Evolutionary Perspectives on Genetic and Environmental Risk Factors for Psychiatric Disorders”, 2018
-
“Thinking About the Evolution of Complex Traits in the Era of Genome-Wide Association Studies”, 2019
-
“Genetic contributions to variation in human stature in prehistoric Europe”, et al 2019
AI:
-
“The Bitter Lesson”, 2019
-
Language models: “Better Language Models and Their Implications”, OpenAI 2019 (“Language Models are Unsupervised Multitask Learners”, et al 2019)
-
“EfficientNet: Rethinking Model Scaling for Convolutional Neural Networks”, 2019
-
“Adversarial Examples Are Not Bugs, They Are Features”, et al 2019 ( discussion)
-
MuseNet: large Sparse Transformers for MIDI music generation (et al 2019 )
-
-
“MuZero: Mastering Atari, Go, Chess and Shogi by Planning with a Learned Model”, et al 2019
-
“Grandmaster level in StarCraft II using multi-agent reinforcement learning”, et al 2019 (blog: [1](https://deepmind.google/discover/blog/alphastar-mastering-the-real-time-strategy-game-starcraft-ii/ “AlphaStar: Mastering the Real-Time Strategy Game StarCraft II”/)/2)
-
“Dactyl: Solving Rubik’s Cube With A Robot Hand”, et al 2019 ( blog)
-
“Dota 2 with Large Scale Deep Reinforcement Learning”, et al 2019 ( blog)
-
‘Pluribus’: “Superhuman AI for multiplayer poker”, 2019
-
“Meta-learning of Sequential Strategies”, et al 2019; “Reinforcement Learning, Fast and Slow”, et al 2019; “Meta-learners’ learning dynamics are unlike learners’”, 2019; “Ray Interference: a Source of Plateaus in Deep Reinforcement Learning”, et al 2019
-
On “Meta Reinforcement Learning”, Lilian Weng
-
“Learning to Predict Without Looking Ahead: World Models Without Forward Prediction”, et al 2019
-
“ICML 2019 Notes”, David Abel
-
Statistics/meta-science:
-
“Most Rigorous Large-Scale Educational RCTs are Uninformative: Should We Be Concerned?”, Lortie-2019
-
“Meta-Research: A comprehensive review of randomized clinical trials in three medical journals reveals 396 [13%] medical reversals”, Herrera-et al 2019
-
“Comparing meta-analyses and preregistered multiple-laboratory replication projects”, et al 2019
-
“The Big Crunch”, David 1994; “1960: The Year The Singularity Was Cancelled”, Scott Alexander
-
“We Need a New Science of Progress: Humanity needs to get better at knowing how to get better”, Patrick Collison & Tyler Cowen
-
“Ingredients for creating disruptive research teams”, Stefan Torges
-
“Evidence on good forecasting practices from the Good Judgment Project”
-
“The maddening saga of how an Alzheimer’s ‘cabal’ thwarted progress toward a cure for decades”; amyloid does not even predict cognitive decline
-
“Registered reports: an early example and analysis”, et al 2019
-
“bioRxiv: the preprint server for biology”, et al 2019
Politics/religion:
-
“The Real War 1939–1945”, Paul 1989; “Why Men Love War”, 1984
-
“How Gullible are We? A Review of the Evidence from Psychology and Social Science”, 2017
-
“Aztec Political Thought”; “Nggwal, who travels in structures of fiber and bone atop rivers of blood.”
-
“Anthropology’s Science Wars: Insights from a New Survey”, et al 2019 ( visualizations)
Psychology:
-
“The impact of background music on adult listeners: A meta-analysis”, et al 2011
-
“Debunking the Stanford Prison Experiment”, Le 2019; Did psychologist David Rosenhan fabricate his famous 1973 “Being Sane in Insane Places” mental hospital exposé?; “A real-life Lord of the Flies: the troubling legacy of the Robbers Cave experiment”
-
“What the voice inside your head says about you: We tend to assume that our internal monologue ‘speaks’ in words—but it turns out that, for many of us, it’s much more complicated” (see also “Toward a phenomenology of inner speaking”, et al 2013)
-
“Doing Despite Disliking: Self-regulatory Strategies in Everyday Aversive Activities”, et al 2018 (see also Structured Procrastination)
-
“Enjoy It Again: Repeat Experiences Are Less Repetitive Than People Think”, 2019
Biology:
-
“Restoration of brain circulation and cellular functions hours post-mortem”, et al 2019
-
“A Look Back at 2019: Progress Towards the Treatment of Aging as a Medical Condition”, Reason
-
“Widespread associations between grey matter structure and the human phenome”, Couvy-et al 2019
-
“Invisible Designers: Brain Evolution Through the Lens of Parasite Manipulation”, Del 2019
Technology:
-
“STEPS Toward Expressive Programming Systems: ‘A Science Experiment’”, et al 2012
-
“How can we develop transformative tools for thought?”, Andy Matuschak & Michael Nielsen 2019
-
“Measuring Long-term Impact of Ads on LinkedIn Feed”, et al 2019
Economics:
-
“Roads and Bridges: The Unseen Labor Behind Our Digital Infrastructure”, Nadia 2016
-
“The changing structure of American innovation: Some cautionary remarks for economic growth”, et al 2020
-
“End-To-End Arguments In System Design”, et al 1984; “Observations on Errors, Corrections, & Trust of Dependent Systems”, James Hamilton (the end-to-end principle)
-
“Labour repression—the Indo-Japanese divergence”, Pseudoerasmus
-
“The Great Shift in Japanese Pop Culture—Part One”/Part Two/Part Three/Part Four/Part Five
-
“Managing an iconic old luxury brand in a new luxury economy: Hermès handbags in the US market”, 2014 (summary; see also “Birkin Demand: A Sage & Stylish Investment”, 2016)
-
“The Price of Nails since 1700: Even Simple Products Experienced Large Price Declines”, 2017
-
“The Corporate Governance of Benedictine Abbeys: What can Stock Corporations Learn from Monasteries?”, et al 2010
Philosophy:
-
“Two Arms and a Head: The Death of a Newly Paraplegic Philosopher”, 2008
-
“Possible Girls”, 2008
-
“Consider the Lobster: For 56 years, the Maine Lobster Festival has been drawing crowds with the promise of sun, fun, and fine food. One visitor would argue that the celebration involves a whole lot more”, David Foster Wallace 2004
Fiction:
-
RIP Gene Wolfe
-
“A Colder War”, Charles 2000
-
“The Proverbial Murder Mystery”, SSC, SSC (see also “The Study of Anglophysics”)
-
“A science fiction writer of the Fifties”, Brad Leithauser 2006
-
“Killing Rabbits”, Miroslav 1963
-
“The First Sally (A), or, Trurl’s Electronic Bard”, Stanisław Lem
-
“Neon Genesis Evangelion: Graphic designer Peiran Tan plumbs the typographic psyche of the celebrated anime franchise”; Typography in Alien & Blade Runner
Books
Nonfiction:
TV/movies
Nonfiction movies:
Fiction:
-
Invasion of the Body Snatchers, 1978 (review)
-
Rurouni Kenshin 2012/Rurouni Kenshin: Kyoto Inferno 2014/Rurouni Kenshin: The Legend Ends 2014 (review)
Anime:
-
Neon Genesis Evangelion Concurrency Project, 2013 (review)
-
Psychedelics enthusiasts have never forgiven me for this, no matter how much I have been vindicated by subsequent studies.↩︎
-
Barring, of course, additional factors like publication bias or fraud or software errors, the latter of which have happened.↩︎
-
This should not have been a surprise to me, after seeing how, after the disastrous imperial presidency of George W. Bush, liberals fell over themselves to defend all administration policies and abuses once a Democrat was in office, and so on with Trump. Politics is the mindkiller.↩︎
-
The psychology of religion in small children is revealing in this way: small children do not believe people are made of carefully-arranged parts. They just are. So if someone dies, they must go somewhere else—that’s just basic object-persistence and conservation!↩︎