A log of experiments done on the site design, intended to render pages more readable, focusing on the challenge of testing a static site, page width, fonts, plugins, and effects of advertising.
- Background
- Problems With “Conversion” Metric
- Ideas For Testing
- Testing
-
Resumption: ABalytics
-
max-width
Redux - Fonts
- Line Height
- Null Test
- Text & Background Color
- List Symbol And Font-Size
- Blockquote Formatting
- Font Size & ToC Background
- Section Header Capitalization
- ToC Formatting
- BeeLine Reader Text Highlighting
- Floating Footnotes
- Indented Paragraphs
- Sidebar Elements
- Moving Sidebar Metadata Into Page
- CSE
- Banner Ad Effect on Total Traffic
-
- Deep Reinforcement Learning
- Indentation + Left-Justified Text
- Appendix
To gain some statistical & web development experience and to improve my readers’ experiences, I have been running a series of CSS A/B tests since June 2012. As expected, most do not show any meaningful difference.
Background
-
https://www.google.com/analytics/siteopt/exptlist?account=18912926
-
http://www.pqinternet.com/196.htm
-
https://support.google.com/websiteoptimizer/bin/answer.py?answer=61203 “Experiment with site-wide changes”
-
https://support.google.com/websiteoptimizer/bin/answer.py?answer=117911 “Working with global headers”
-
https://support.google.com/websiteoptimizer/bin/answer.py?answer=61427
-
https://support.google.com/websiteoptimizer/bin/answer.py?answer=188090 “Varying page and element styles” - testing with inline CSS overriding the defaults
-
https://stackoverflow.com/questions/2993199/with-google-website-optimizers-multivariate-testing-can-i-vary-multiple-css-cl
-
http://www.xemion.com/blog/the-secret-to-painless-google-website-optimizer-70.html
-
https://stackoverflow.com/tags/google-website-optimizer/hot
Problems With “Conversion” Metric
https://support.google.com/websiteoptimizer/bin/answer.py?answer=74345 “Time on page as a conversion goal” - every page converts, by using a timeout (mine is 40 seconds). Problem: dichotomizing a continuous variable into a single binary variable destroys a massive amount of information. This is well-known in the statistical and psychological literature (eg. Macet al 2002 ) but I’ll illustrate further with some information-theoretical observations.
According to my Analytics, the mean reading time (time on page) is 1:47 and the maximum bracket, hit by 1% of viewers, is 1801 seconds, and the range 1-1801 takes <10.8 bits to encode (log2(1801) → 10.81
), hence each page view could be represented by <10.8 bits (less since reading time is so highly skewed). But if we dichotomize, then we learn simply that ~14% of readers will read for 40 seconds, hence each reader carries not 6 bits, nor 1 bit (if 50% read that long) but closer to 2/3 of a bit:
p=0.14; q=1-p; (-p*log2(p) - q*log2(q))
# [1] 0.5842
This isn’t even an efficient dichotomization: we could improve the fractional bit to 1 bit if we could somehow dichotomize at 50% of readers:
p=0.50; q=1-p; (-p*log2(p) - q*log2(q))
# [1] 1
But unfortunately, simply lowering the timeout will have minimal returns as Analytics also reports that 82% of reader spend 0-10 seconds on pages. So we are stuck with a severe loss.
Ideas For Testing
CSS
differences from readability
every declaration in default.CSS?
-
test the suggestions in https://code.google.com/p/better-web-readability-project/ http://www.vcarrer.com/2009/05/how-we-read-on-web-and-how-can-we.html
Testing
max-width
CSS-3 property: set how wide the page will be in pixels if unlimited screen real estate is available. I noticed some people complained that pages were ‘too wide’ and this made it hard to read, which apparently is a real thing since lines are supposed to fit in eye saccades. So I tossed in 800px, 900px, 1300px, and 1400px to the first A/B test.
<script>
function utmx_section(){}function utmx(){}
(function(){var k='0520977997',d=document,l=d.location,c=d.cookie;function f(n){
if(c){var i=c.indexOf(n+'=');if(i>-1){var j=c.indexOf(';',i);return escape(c.substring(i+n.
length+1,j<0?c.length:j))}}}var x=f('__utmx'),xx=f('__utmxx'),h=l.hash;
d.write('<sc'+'ript src="'+
'http'+(l.protocol=='https:'?'s://ssl':'://www')+'.google-analytics.com'
+'/siteopt.js?v=1&utmxkey='+k+'&utmx='+(x?x:'')+'&utmxx='+(xx?xx:'')+'&utmxtime='
+new Date().valueOf()+(h?'&utmxhash='+escape(h.substr(1)):'')+
'" type="text/javascript" charset="utf-8"></sc'+'ript>')})();
</script>
<script type="text/javascript">
var _gaq = _gaq || [];
_gaq.push(['gwo._setAccount', 'UA-18912926-2']);
_gaq.push(['gwo._trackPageview', '/0520977997/test']);
(function() {
var ga = document.createElement('script'); ga.type = 'text/javascript'; ga.async = true;
ga.src = ('https:' == document.location.protocol ? 'https://ssl' : 'http://www')
+ '.google-analytics.com/ga.js';
var s = document.getElementsByTagName('script')[0]; s.parentNode.insertBefore(ga, s);
})();
</script>
<script type="text/javascript">
var _gaq = _gaq || [];
_gaq.push(['gwo._setAccount', 'UA-18912926-2']);
setTimeout(function() {
_gaq.push(['gwo._trackPageview', '/0520977997/goal']);
}, 40000);
(function() {
var ga = document.createElement('script'); ga.type = 'text/javascript'; ga.async = true;
ga.src = ('https:' == document.location.protocol ? 'https://ssl' : 'http://www') +
'.google-analytics.com/ga.js';
var s = document.getElementsByTagName('script')[0]; s.parentNode.insertBefore(ga, s);
})();
</script>
<script>utmx_section("max width")</script>
<style>
body { max-width: 800px; }
</style>
</noscript>
It ran from mid-June to 2012-08-01. Unfortunately, I cannot be more specific: on 1 August, Google deleted Website Optimizer and told everyone to use ‘Experiments’ in Google Analytics - and deleted all my information. The graph over time, the exact numbers - all gone. So this is from memory.
The results were initially very promising: ‘conversion’ was defined as staying on a page for 40 seconds (I reasoned that this meant someone was actually reading the page), and had a base of around 70% of readers converting. With a few hundred hits, 900px converted at 10-20% more than the default! I was ecstatic. So when it began falling, I was only a little bothered (one had to expect some regression to the mean since the results were too good to be true). But as the hits increased into the low thousands, the effect kept shrinking all the way down to 0.4% improved conversion. At some points, 1300px actually exceeded 900px.
The second distressing thing was that Google’s estimated chance of a particular intervention beating the default (which I believe is a Bonferroni-corrected p-value), did not increase! Even as each version received 20,000 hits, the chance stubbornly bounced around the 70-90% range for 900px and 1300px. This remained true all the way to the bitter end. At the end, each version had racked up 93,000 hits and still was in the 80% decile. Wow.
Ironically, I was warned at the beginning about both of these possible behaviors by a paper I read on large-scale corporate A/B testing: http://www.exp-platform.com/Documents/puzzlingOutcomesInControlledExperiments.pdf and http://www.exp-platform.com/Documents/controlledExperimentDMKD.pdf and http://www.exp-platform.com/Documents/2013%20controlledExperimentsAtScale.pdf It covered at length how many apparent trends simply evaporated, but it also covered later a peculiar phenomenon where A/B tests did not converge even after being run on ungodly amounts of data because the standard deviations kept changing (the user composition kept shifting and rendering previous data more uncertain). And it’s a general phenomenon that even for large correlations, the trend will bounce around a lot before it stabilizes (2013).
Oy vey! When I discovered Google had deleted my results, I decided to simply switch to 900px. Running a new test would not provide any better answers.
TODO
how about a blue background? see https://www.overcomingbias.com/2010/06/near-far-summary.html for more design ideas
-
table striping
tbody tr:hover td { background-color: #f5f5f5;}
tbody tr:nth-child(odd) td { background-color: #f9f9f9;}
-
link decoration
a { color: black; text-decoration: underline;}
a { color:#005AF2; text-decoration:none; }
Resumption: ABalytics
In March 2013, I decided to give A/B testing another whack. Google Analytics Experiment did not seem to have improved and the commercial services continued to charge unacceptable prices, so I gave the Google Analytics custom variable integration approach another trying using ABalytics. The usual puzzling, debugging, and frustration of combining so many disparate technologies (HTML and CSS and JS and Google Analytics) aside, it seemed to work on my test page. The current downside seems to be that the ABalytics approach may be fragile, and the UI in GA is awful (you have to do the statistics yourself).
max-width
Redux
The test case is to rerun the max-width
test and finish it.
Implementation
The exact changes:
Sun Mar 17 11:25:39 EDT 2013 gwern@gwern.net
* default.html: setup ABalytics a/b testing https://github.com/danmaz74/ABalytics
(hope this doesn't break anything...)
addfile ./static/js/abalytics.js
hunk ./static/js/abalytics.js 1
...
hunk ./static/template/default.html 28
+ <div class="maxwidth_class1"></div>
+
...
- <noscript><p>Enable JavaScript for Disqus comments</p></noscript>
+ window.onload = function() {
+ ABalytics.applyHtml();
+ };
+ </script>
hunk ./static/template/default.html 119
+
+ ABalytics.init({
+ maxwidth: [
+ {
+ name: '800',
+ "maxwidth_class1": "<style>body { max-width: 800px; }</style>",
+ "maxwidth_class2": ""
+ },
+ {
+ name: '900',
+ "maxwidth_class1": "<style>body { max-width: 900px; }</style>",
+ "maxwidth_class2": ""
+ },
+ {
+ name: '1100',
+ "maxwidth_class1": "<style>body { max-width: 1100px; }</style>",
+ "maxwidth_class2": ""
+ },
+ {
+ name: '1200',
+ "maxwidth_class1": "<style>body { max-width: 1200px; }</style>",
+ "maxwidth_class2": ""
+ },
+ {
+ name: '1300',
+ "maxwidth_class1": "<style>body { max-width: 1300px; }</style>",
+ "maxwidth_class2": ""
+ },
+ {
+ name: '1400',
+ "maxwidth_class1": "<style>body { max-width: 1400px; }</style>",
+ "maxwidth_class2": ""
+ }
+ ],
+ }, _gaq);
+
Results
I wound up the test on 2013-04-17 with the following results:
Width (px) |
Visits |
Conversion |
---|---|---|
1100 |
18,164 |
14.49% |
1300 |
18,071 |
14.28% |
1200 |
18,150 |
13.99% |
800 |
18,599 |
13.94% |
900 |
18,419 |
13.78% |
1400 |
18,378 |
13.68% |
109772 |
14.03% |
Analysis
1100px is close to my original A/B test indicating 1000px was the leading candidate, so that gives me additional confidence, as does the observation that 1300px and 1200px are the other leading candidates. (Curiously, the site conversion average before was 13.88%; perhaps my underlying traffic changed slightly around the time of the test? This would demonstrate why alternatives need to be tested simultaneously.) A quick and dirty R test of 1100px vs 1300px (prop.test(c(2632,2581),c(18164,18071))
) indicates the difference isn’t statistically-significant (at p = 0.58), and we might want more data; worse, there is no clear linear relation between conversion and width (the plot is erratic, and a linear fit a dismal p = 0.89):
rates <- read.csv(stdin(),header=TRUE)
Width,N,Rate
1100,18164,0.1449
1300,18071,0.1428
1200,18150,0.1399
800,18599,0.1394
900,18419,0.1378
1400,18378,0.1368
rates$Successes <- rates$N * rates$Rate
rates$Successes <- round(rates$Successes,0)
rates$Failures <-rates$N - rates$Successes
g <- glm(cbind(Successes,Failures) ~ Width, data=rates, family="binomial")
# ...Coefficients:
# Estimate Std. Error z value Pr(>|z|)
# (Intercept) -1.82e+00 4.65e-02 -39.12 <2e-16
# Width 5.54e-06 4.10e-05 0.14 0.89
## not much better:
rates$Width <- as.factor(rates$Width)
rates$Width <- relevel(rates$Width, ref="900")
g2 <- glm(cbind(Successes,Failures) ~ Width, data=rates, family="binomial"); summary(g2)
But I want to move on to the next test and by the same logic it is highly unlikely that the difference between them is large or much in 1300px’s favor (the kind of mistake I care about: switching between 2 equivalent choices doesn’t matter, missing out on an improvement does matter - maximizing β, not minimizing α).
Fonts
The New York Times ran an informal online experiment with a large number of readers (n = 60750) and found that the Baskerville font led to more readers agreeing with a short text passage - this seems plausible enough given their very large sample size and Wikipedia’s note that “The refined feeling of the typeface makes it an excellent choice to convey dignity and tradition.”
Power Analysis
Would this font work its magic on Gwern.net too? Let’s see. The sample size is quite manageable, as over a month I will easily have 60k visits, and they tested 6 fonts, expanding their necessary sample. What sample size do I actually need? Their professor estimates the effect size of Baskerville at 1.5%; I would like my A/B test to have very high statistical power (0.9) and reach more stringent statistical-significance (p < 0.01) so I can go around and in good conscience tell people to use Baskerville. I already know the average “conversion rate” is ~13%, so I get this power calculation:
power.prop.test(p1=0.13+0.015, p2=0.13, power=0.90, sig.level=0.01)
Two-sample comparison of proportions power calculation
n = 15683
p1 = 0.145
p2 = 0.13
sig.level = 0.01
power = 0.9
alternative = two.sided
NOTE: n is number in *each* group
15000 visitors in each group seems reasonable; at ~16k visitors a week, that suggests a few weeks of testing. Of course I’m testing 4 fonts (see below), but that still fits in the ~2 months I’ve allotted for this test.
Implementation
I had previously drawn on the NYT experiment for my site design:
html {
...
font-family: Georgia, "HelveticaNeue-Light", "Helvetica Neue Light", "Helvetica Neue", Helvetica,
Arial, "Lucida Grande", garamond, palatino, verdana, sans-serif;
}
I had not used Baskerville but Georgia since Georgia seemed similar and was convenient, but we’ll fix that now. Besides Baskerville & Georgia, we’ll omit Comic Sans (of course), but we can try Trebuchet for a total of 4 fonts (falling back to Georgia):
hunk ./static/template/default.html 28
+ <div class="fontfamily_class1"></div>
...
hunk ./static/template/default.html 121
+ fontfamily: [
+ {
+ name: 'Baskerville',
+ "fontfamily_class1": "<style>html { font-family: Baskerville, Georgia; }</style>",
+ "fontfamily_class2": ""
+ },
+ {
+ name: 'Georgia',
+ "fontfamily_class1": "<style>html { font-family: Georgia; }</style>",
+ "fontfamily_class2": ""
+ },
+ {
+ name: 'Trebuchet',
+ "fontfamily_class1": "<style>html { font-family: 'Trebuchet MS', Georgia; }</style>",
+ "fontfamily_class2": ""
+ },
+ {
+ name: 'Helvetica',
+ "fontfamily_class1": "<style>html { font-family: Helvetica, Georgia; }</style>",
+ "fontfamily_class2": ""
+ }
+ ],
Results
Running from 2013-04-14 to 2013-06-16:
Font |
Type |
Visits |
Conversion |
---|---|---|---|
Trebuchet |
sans |
35,473 |
13.81% |
Baskerville |
serif |
36,021 |
13.73% |
Helvetica |
sans |
35,656 |
13.43% |
Georgia |
serif |
35,833 |
13.31% |
sans |
71,129 |
13.62% |
|
serif |
71,854 |
13.52% |
|
142,983 |
13.57% |
The sample size for each font is 20k higher than I projected due to the enormous popularity of an analysis of the lifetimes of Google services I finished during the test. Regardless, it’s clear that the results - with double the total sample size of the NYT experiment, focused on fewer fonts - are disappointing and there seems to be very little difference between fonts.
Analysis
Picking the most extreme difference, between Trebuchet and Georgia, the difference is close to the usual definition of statistical-significance:
prop.test(c(0.1381*35473,0.1331*35833),c(35473,35833))
# 2-sample test for equality of proportions with continuity correction
#
# data: c(0.1381 * 35473, 0.1331 * 35833) out of c(35473, 35833)
# X-squared = 3.76, df = 1, p-value = 0.0525
# alternative hypothesis: two.sided
# 95% confidence interval:
# -5.394e-05 1.005e-02
# sample estimates:
# prop 1 prop 2
# 0.1381 0.1331
Which naturally implies that the much smaller difference between Trebuchet and Baskerville is not statistically-significant:
prop.test(c(0.1381*35473,0.1373*36021), c(35473,36021))
# 2-sample test for equality of proportions with continuity correction
#
# data: c(0.1381 * 35473, 0.1373 * 36021) out of c(35473, 36021)
# X-squared = 0.0897, df = 1, p-value = 0.7645
# alternative hypothesis: two.sided
# 95% confidence interval:
# -0.00428 0.00588
Since there’s only small differences between individual fonts, I wondered if there might be a difference between the two sans-serifs and the two serifs. If we lump the 4 fonts into those 2 categories and look at the small difference in mean conversion rate:
prop.test(c(0.1362*71129,0.1352*71854), c(71129,71854))
# 2-sample test for equality of proportions with continuity correction
#
# data: c(0.1362 * 71129, 0.1352 * 71854) out of c(71129, 71854)
# X-squared = 0.2963, df = 1, p-value = 0.5862
# alternative hypothesis: two.sided
# 95% confidence interval:
# -0.002564 0.004564
Nothing doing there either. More generally:
rates <- read.csv(stdin(),header=TRUE)
Font,Serif,N,Rate
Trebuchet,FALSE,35473,0.1381
Baskerville,TRUE,6021,0.1373
Helvetica,FALSE,35656,0.1343
Georgia,TRUE,5833,0.1331
rates$Successes <- rates$N * rates$Rate
rates$Successes <- round(rates$Successes,0)
rates$Failures <-rates$N - rates$Successes
g <- glm(cbind(Successes,Failures) ~ Font, data=rates, family="binomial"); summary(g)
# ...Coefficients:
# Estimate Std. Error z value Pr(>|z|)
# (Intercept) -1.83745 0.03744 -49.08 <2e-16
# FontGeorgia -0.03692 0.05374 -0.69 0.49
# FontHelvetica -0.02591 0.04053 -0.64 0.52
# FontTrebuchet 0.00634 0.04048 0.16 0.88
With essentially no meaningful differences between conversion rates, this suggests that however fonts matter, they don’t matter for reading duration. So I feel free to pick the font that appeals to me visually, which is Baskerville.
Line Height
I have seen complaints that lines on Gwern.net are “too closely spaced” or “run together” or “cramped”, referring to the line height (the CSS property line-height
). I set the CSS to line-height: 150%;
to deal with this objection, but this was a simple hack based on rough eyeballing of it, and it was done before I changed the max-width
and font-family
settings after the previous testing. So it’s worth testing some variants.
Most web design guides seem to suggest a safe default of 120%, rather than my current 150%. If we try to test each decile plus one on the outside, that’d give us 110, 120, 130, 140, 150, 160 or 6 options, which combined with the expected small effect, would require an unreasonable sample size (and I have nothing in the pipeline I expect might catch fire like the Google analysis and deliver an excess >50k visits). So I’ll try just 120/130/140/150, and schedule a similar block of time as fonts (ending the experiment on 2013-08-16, with presumably >70k datapoints).
Implementation
hunk ./static/template/default.html 30
- <div class="fontfamily_class1"></div>
+ <div class="linewidth_class1"></div>
hunk ./static/template/default.html 156
- fontfamily:
+ linewidth:
hunk ./static/template/default.html 158
- name: 'Baskerville',
- "fontfamily_class1": "<style>html { font-family: Baskerville, Georgia; }</style>",
- "fontfamily_class2": ""
+ name: 'Line120',
+ "linewidth_class1": "<style>div#content { line-height: 120%;}</style>",
+ "linewidth_class2": ""
hunk ./static/template/default.html 163
- name: 'Georgia',
- "fontfamily_class1": "<style>html { font-family: Georgia; }</style>",
- "fontfamily_class2": ""
+ name: 'Line130',
+ "linewidth_class1": "<style>div#content { line-height: 130%;}</style>",
+ "linewidth_class2": ""
hunk ./static/template/default.html 168
- name: 'Trebuchet',
- "fontfamily_class1": "<style>html { font-family: 'Trebuchet MS', Georgia; }</style>",
- "fontfamily_class2": ""
+ name: 'Line140',
+ "linewidth_class1": "<style>div#content { line-height: 140%;}</style>",
+ "linewidth_class2": ""
hunk ./static/template/default.html 173
- name: 'Helvetica',
- "fontfamily_class1": "<style>html { font-family: Helvetica, Georgia; }</style>",
- "fontfamily_class2": ""
+ name: 'Line150',
+ "linewidth_class1": "<style>div#content { line-height: 150%;}</style>",
+ "linewidth_class2": ""
Analysis
From 2013-06-15–2013-08-15:
line % |
n |
Conversion % |
---|---|---|
130 |
18,124 |
15.26 |
150 |
17,459 |
15.22 |
120 |
17,773 |
14.92 |
140 |
17,927 |
14.92 |
71,283 |
15.08 |
Just from looking at the miserably small difference between the most extreme percentages (15.26 - 14.92 = 0.34%), we can predict that nothing here was statistically-significant:
x1 <- 18124; x2 <- 17927; prop.test(c(x1*0.1524, x2*0.1476), c(x1,x2))
# 2-sample test for equality of proportions with continuity correction
#
# data: c(x1 * 0.1524, x2 * 0.1476) out of c(x1, x2)
# X-squared = 1.591, df = 1, p-value = 0.2072
I changed the 150% to 130% for the heck of it, even though the difference between 130 and 150 was trivially small:
rates <- read.csv(stdin(),header=TRUE)
Width,N,Rate
130,18124,0.1526
150,17459,0.1522
120,17773,0.1492
140,17927,0.1492
rates$Successes <- rates$N * rates$Rate
rates$Successes <- round(rates$Successes,0)
rates$Failures <-rates$N - rates$Successes
rates$Width <- as.factor(rates$Width)
g <- glm(cbind(Successes,Failures) ~ Width, data=rates, family="binomial")
# ...Coefficients:
# Estimate Std. Error z value Pr(>|z|)
# (Intercept) -1.74e+00 2.11e-02 -82.69 <2e-16
# Width130 2.65e-02 2.95e-02 0.90 0.37
# Width140 9.17e-06 2.97e-02 0.00 1.00
# Width150 2.32e-02 2.98e-02 0.78 0.44
Null Test
One of the suggestions in the A/B testing papers was to run a “null” A/B test (or “A/A test”) where the payload is empty but the A/B testing framework is still measuring conversions etc. By definition, the null hypothesis of “no difference” should be true and at an alpha of 0.05, only 5% of the time would the null tests yield a p < 0.05 (which is very different from the usual situation). The interest here is that it’s possible that something is going wrong in one’s A/B setup or in general, and so if one gets a “statistically-significant” result, it may be worthwhile investigating this anomaly.
It’s easy to switch from the lineheight test to the null test; just rename the variables for Google Analytics, and empty the payloads:
hunk ./static/template/default.html 30
- <div class="linewidth_class1"></div>
+ <div class="null_class1"></div>
hunk ./static/template/default.html 158
- linewidth: [
+ null: [
+ ...]]
hunk ./static/template/default.html 160
- name: 'Line120',
- "linewidth_class1": "<style>div#content { line-height: 120%;}</style>",
+ name: 'null1',
+ "null_class1": "",
hunk ./static/template/default.html 165
- { ...
- name: 'Line130',
- "linewidth_class1": "<style>div#content { line-height: 130%;}</style>",
- "linewidth_class2": ""
- },
- {
- name: 'Line140',
- "linewidth_class1": "<style>div#content { line-height: 140%;}</style>",
- "linewidth_class2": ""
- },
- {
- name: 'Line150',
- "linewidth_class1": "<style>div#content { line-height: 150%;}</style>",
+ name: 'null2',
+ "null_class1": "",
+ ... }
Since any difference due to the testing framework should be noticeable, this will be a shorter experiment, from 15 August to 29 August.
Results
While amusingly the first pair of 1k hits resulted in a dramatic 18% vs 14% result, this quickly disappeared into a much more normal-looking set of data:
option |
n |
conversion |
---|---|---|
null2 |
7,359 |
16.23% |
null1 |
7,488 |
15.89% |
14,847 |
16.06% |
Analysis
Ah, but can we reject the null hypothesis that [] == []? In a rare victory for null-hypothesis-significance-testing, we do not commit a Type I error:
x1 <- 7359; x2 <- 7488; prop.test(c(x1*0.1623, x2*0.1589), c(x1,x2))
# 2-sample test for equality of proportions with continuity correction
#
# data: c(x1 * 0.1623, x2 * 0.1589) out of c(x1, x2)
# X-squared = 0.2936, df = 1, p-value = 0.5879
# alternative hypothesis: two.sided
# 95% confidence interval:
# -0.008547 0.015347
Hurray—we controlled our false-positive error rate! But seriously, it is nice to see that ABalytics does not seem to be broken & favoring either option and any results driven by placement in the array of options.
Text & Background Color
As part of the generally monochromatic color scheme, the background was off-white (grey) and the text was black:
html { ...
background-color: #FCFCFC; /* off-white */
color: black;
... }
The hyperlinks, on the other hand, make use of an off-black color: #303C3C
, partially motivated by Ian Storm Taylor’s advice to “Never Use Black”. I wonder - should all the text be off-black too? And which combination is best? White/black? Off-white/black? Off-white/off-black? White/off-black? Let’s try all 4 combinations here.
Implementation
The usual:
hunk ./static/template/default.html 30
- <div class="underline_class1"></div>
+ <div class="ground_class1"></div>
hunk ./static/template/default.html 155
- underline: [
+ ground: [
hunk ./static/template/default.html 157
- name: 'underlined',
- "underline_class1": "<style>a { color: #303C3C; text-decoration: underline; }</style>",
- "underline_class2": ""
+ name: 'bw',
+ "ground_class1": "<style>html { background-color: white; color: black; }</style>",
+ "ground_class2": ""
hunk ./static/template/default.html 162
- name: 'notUnderlined',
- "underline_class1": "<style>a { color: #303C3C; text-decoration: none; }</style>",
- "underline_class2": ""
+ name: 'obw',
+ "ground_class1": "<style>html { background-color: white; color: #303C3C; }</style>",
+ "ground_class2": ""
+ },
+ {
+ name: 'bow',
+ "ground_class1": "<style>html { background-color: #FCFCFC; color: black; }</style>",
+ "ground_class2": ""
+ },
+ {
+ name: 'obow',
+ "ground_class1": "<style>html { background-color: #FCFCFC; color: #303C3C; }</style>",
+ "ground_class2": ""
... ]]
Data
I am a little curious about this one, so I scheduled a full month and half: 10 September - 20 October. Due to far more traffic than anticipated from submissions to Hacker News, I cut it short by 10 days to avoid wasting traffic on a test which was done (a total n of 231,599 was more than enough). The results:
Version |
n |
Conversion |
---|---|---|
bw |
58,237 |
12.90% |
obow |
58,132 |
12.62% |
bow |
57,576 |
12.48% |
obw |
57,654 |
12.44% |
Analysis
rates <- read.csv(stdin(),header=TRUE)
Black,White,N,Rate
TRUE,TRUE,58237,0.1290
FALSE,FALSE,58132,0.1262
TRUE,FALSE,57576,0.1248
FALSE,TRUE,57654,0.1244
rates$Successes <- rates$N * rates$Rate
rates$Successes <- round(rates$Successes,0)
rates$Failures <-rates$N - rates$Successes
g <- glm(cbind(Successes,Failures) ~ Black * White, data=rates, family="binomial")
summary(g)
# ...Coefficients:
# Estimate Std. Error z value Pr(>|z|)
# (Intercept) -1.9350 0.0125 -154.93 <2e-16
# BlackTRUE -0.0128 0.0177 -0.72 0.47
# WhiteTRUE -0.0164 0.0178 -0.92 0.36
# BlackTRUE:WhiteTRUE 0.0545 0.0250 2.17 0.03
#
# (Dispersion parameter for binomial family taken to be 1)
#
# Null deviance: 6.8625e+00 on 3 degrees of freedom
# Residual deviance: -1.1758e-11 on 0 degrees of freedom
# AIC: 50.4
summary(step(g))
# same thing
So we can estimate the net effect of the 4 possibilities:
-
Black, White: -0.0128 + -0.0164 + 0.0545 = 0.0253
-
Off-black, Off-white: 0 + 0 + 0 = 0
-
Black, Off-white: -0.0128 + 0 + 0 = -0.0128
-
Off-black, White: 0 + -0.0164 + 0 = -0.0164
The results exactly match the data’s rankings.
So, this suggests a change to the CSS: we switch the default background color from #FCFCFC
to white
, while leaving the default color
its current black
.
Reader Lucas asks in the comment sections whether, since we would expect new visitors to the website to be less likely to read a page in full than a returning visitor (who knows what they’re in for & probably wants more), whether including such a variable (which is something Google Analytics does track) might improve the analysis. It’s easy to ask GA for “New vs Returning Visitor” so I did:
rates <- read.csv(stdin(),header=TRUE)
Black,White,Type,N,Rate
FALSE,TRUE,new,36695,0.1058
FALSE,TRUE,old,21343,0.1565
FALSE,FALSE,new,36997,0.1043
FALSE,FALSE,old,21537,0.1588
TRUE,TRUE,new,36600,0.1073
TRUE,TRUE,old,22274,0.1613
TRUE,FALSE,new,36409,0.1075
TRUE,FALSE,old,21743,0.1507
rates$Successes <- rates$N * rates$Rate
rates$Successes <- round(rates$Successes,0)
rates$Failures <-rates$N - rates$Successes
g <- glm(cbind(Successes,Failures) ~ Black * White + Type, data=rates, family="binomial")
summary(g)
# Coefficients:
# Estimate Std. Error z value Pr(>|z|)
# (Intercept) -2.134459 0.013770 -155.01 <2e-16
# BlackTRUE -0.009219 0.017813 -0.52 0.60
# WhiteTRUE 0.000837 0.017798 0.05 0.96
# BlackTRUE:WhiteTRUE 0.034362 0.025092 1.37 0.17
# Typeold 0.448004 0.012603 35.55 <2e-16
-
B/W: (-0.009219) + 0.000837 + 0.034362 = 0.02598
-
0 + 0 + 0 = 0
-
B: (-0.009219) + 0 + 0 = -0.009219
-
W: 0 + 0.000837 + 0 = 0.000837
And again, 0.02598 > 0.000837. So as one hopes, thank to randomization, adding a missing covariate doesn’t change our conclusion.
List Symbol And Font-Size
I make heavy use of unordered lists in articles; for no particular reason, the symbol denoting the start of each entry in a list is the little black square, rather than the more common little circle. I’ve come to find the little squares a little chunky and ugly, so I want to test that. And I just realized that I never tested font size (just type of font), even though increasing font size one of the most common CSS tweaks around. I don’t have any reason to expect an interaction between these two bits of designs, unlike the previous A/B test, but I like the idea of getting more out of my data, so I am doing another factorial design, this time not 2x2 but 3x5. The options:
ul { list-style-type: square; }
ul { list-style-type: circle; }
ul { list-style-type: disc; }
html { font-size: 100%; }
html { font-size: 105%; }
html { font-size: 110%; }
html { font-size: 115%; }
html { font-size: 120%; }
Implementation
A 3x5 design, or 15 possibilities, does get a little bulkier than I’d like:
hunk ./static/template/default.html 30
- <div class="ground_class1"></div>
+ <div class="ulFontSize_class1"></div>
hunk ./static/template/default.html 146
- ground: [
+ ulFontSize: [
hunk ./static/template/default.html 148
- name: 'bw',
- "ground_class1": "<style>html { background-color: white; color: black; }</style>",
- "ground_class2": ""
+ name: 's100',
+ "ulFontSize_class1": "<style>ul { list-style-type: square; }; html { font-size: 100%; }</style>",
+ "ulFontSize_class2": ""
hunk ./static/template/default.html 153
- name: 'obw',
- "ground_class1": "<style>html { background-color: white; color: #303C3C; }</style>",
- "ground_class2": ""
+ name: 's105',
+ "ulFontSize_class1": "<style>ul { list-style-type: square; }; html { font-size: 105%; }</style>",
+ "ulFontSize_class2": ""
hunk ./static/template/default.html 158
- name: 'bow',
- "ground_class1": "<style>html { background-color: #FCFCFC; color: black; }</style>",
- "ground_class2": ""
+ name: 's110',
+ "ulFontSize_class1": "<style>ul { list-style-type: square; }; html { font-size: 110%; }</style>",
+ "ulFontSize_class2": ""
hunk ./static/template/default.html 163
- name: 'obow',
- "ground_class1": "<style>html { background-color: #FCFCFC; color: #303C3C; }</style>",
- "ground_class2": ""
+ name: 's115',
+ "ulFontSize_class1": "<style>ul { list-style-type: square; }; html { font-size: 115%; }</style>",
+ "ulFontSize_class2": ""
+ },
+ {
+ name: 's120',
+ "ulFontSize_class1": "<style>ul { list-style-type: square; }; html { font-size: 120%; }</style>",
+ "ulFontSize_class2": ""
+ },
+ {
+ name: 'c100',
+ "ulFontSize_class1": "<style>ul { list-style-type: circle; }; html { font-size: 100%; }</style>",
+ "ulFontSize_class2": ""
+ },
+ {
+ name: 'c105',
+ "ulFontSize_class1": "<style>ul { list-style-type: circle; }; html { font-size: 105%; }</style>",
+ "ulFontSize_class2": ""
+ },
+ {
+ name: 'c110',
+ "ulFontSize_class1": "<style>ul { list-style-type: circle; }; html { font-size: 110%; }</style>",
+ "ulFontSize_class2": ""
+ },
+ {
+ name: 'c115',
+ "ulFontSize_class1": "<style>ul { list-style-type: circle; }; html { font-size: 115%; }</style>",
+ "ulFontSize_class2": ""
+ },
+ {
+ name: 'c120',
+ "ulFontSize_class1": "<style>ul { list-style-type: circle; }; html { font-size: 120%; }</style>",
+ "ulFontSize_class2": ""
+ },
+ {
+ name: 'd100',
+ "ulFontSize_class1": "<style>ul { list-style-type: disc; }; html { font-size: 100%; }</style>",
+ "ulFontSize_class2": ""
+ },
+ {
+ name: 'd105',
+ "ulFontSize_class1": "<style>ul { list-style-type: disc; }; html { font-size: 105%; }</style>",
+ "ulFontSize_class2": ""
+ },
+ {
+ name: 'd110',
+ "ulFontSize_class1": "<style>ul { list-style-type: disc; }; html { font-size: 110%; }</style>",
+ "ulFontSize_class2": ""
+ },
+ {
+ name: 'd115',
+ "ulFontSize_class1": "<style>ul { list-style-type: disc; }; html { font-size: 115%; }</style>",
+ "ulFontSize_class2": ""
+ },
+ {
+ name: 'd120',
+ "ulFontSize_class1": "<style>ul { list-style-type: disc; }; html { font-size: 120%; }</style>",
+ "ulFontSize_class2": ""
... ]]
Data
I halted the A/B test on 27 October because I was noticing clear damage as compared to my default CSS. The results were:
List icon |
Font zoom |
n |
Reading conversion rate |
---|---|---|---|
square |
100% |
4,763 |
16.38% |
disc |
100% |
4,759 |
16.18% |
disc |
110% |
4,716 |
16.09% |
circle |
115% |
4,933 |
15.95% |
circle |
100% |
4,872 |
15.85% |
circle |
110% |
4,920 |
15.53% |
circle |
120% |
5,114 |
15.51% |
square |
115% |
4,815 |
15.51% |
square |
110% |
4,927 |
15.47% |
circle |
105% |
5,101 |
15.33% |
square |
105% |
4,775 |
14.85% |
disc |
115% |
4,797 |
14.78% |
disc |
105% |
5,006 |
14.72% |
disc |
120% |
4,912 |
14.56% |
square |
120% |
4,786 |
13.96% |
73,196 |
15.38% |
Analysis
Incorporating visitor type:
rates <- read.csv(stdin(),header=TRUE)
Ul,Size,Type,N,Rate
c,120,old,2673,0.1650
c,115,old,2643,0.1854
c,105,new,2636,0.1392
d,105,old,2635,0.1613
s,110,old,2596,0.1749
s,120,old,2593,0.1678
s,105,new,2582,0.1243
d,120,old,2559,0.1649
c,110,new,2558,0.1298
d,110,new,2555,0.1307
c,100,old,2553,0.2002
c,105,old,2539,0.1713
d,115,old,2524,0.1565
s,115,new,2516,0.1391
c,110,old,2505,0.1741
d,100,new,2502,0.1431
c,120,new,2500,0.1284
s,110,new,2491,0.1265
c,115,new,2483,0.1228
d,120,new,2452,0.1277
d,105,new,2448,0.1364
c,100,new,2436,0.1199
d,115,new,2435,0.1437
s,100,new,2411,0.1497
s,120,new,2411,0.1161
s,105,old,2387,0.1571
s,115,old,2365,0.1674
d,100,old,2358,0.1735
s,100,old,2329,0.1803
d,110,old,2235,0.1888
rates$Successes <- rates$N * rates$Rate
rates$Successes <- round(rates$Successes,0)
rates$Failures <-rates$N - rates$Successes
g <- glm(cbind(Successes,Failures) ~ Ul * Size + Type, data=rates, family="binomial"); summary(g)
# ...Coefficients:
# Estimate Std. Error z value Pr(>|z|)
# (Intercept) -1.389310 0.270903 -5.13 2.9e-07
# Uld -0.103201 0.386550 -0.27 0.789
# Uls 0.055036 0.389109 0.14 0.888
# Size -0.004397 0.002458 -1.79 0.074
# Uld:Size 0.000842 0.003509 0.24 0.810
# Uls:Size -0.000741 0.003533 -0.21 0.834
# Typeold 0.317126 0.020507 15.46 < 2e-16
summary(step(g))
# ...Coefficients:
# Estimate Std. Error z value Pr(>|z|)
# (Intercept) -1.40555 0.15921 -8.83 <2e-16
# Size -0.00436 0.00144 -3.02 0.0025
# Typeold 0.31725 0.02051 15.47 <2e-16
#
## examine just the list type alone, since the Size result is clear.
summary(glm(cbind(Successes,Failures) ~ Ul + Type, data=rates, family="binomial"))
# ...Coefficients:
# Estimate Std. Error z value Pr(>|z|)
# (Intercept) -1.8725 0.0208 -89.91 <2e-16
# Uld -0.0106 0.0248 -0.43 0.67
# Uls -0.0265 0.0249 -1.07 0.29
# Typeold 0.3163 0.0205 15.43 <2e-16
summary(glm(cbind(Successes,Failures) ~ Ul + Type, data=rates[rates$Size==100,], family="binomial"))
# ...Coefficients:
# Estimate Std. Error z value Pr(>|z|)
# (Intercept) -1.8425 0.0465 -39.61 < 2e-16
# Uld -0.0141 0.0552 -0.26 0.80
# Uls 0.0353 0.0551 0.64 0.52
# Typeold 0.3534 0.0454 7.78 7.3e-15
The results are a little confusing in factorial form: it seems pretty clear that Size
is bad and that 100% performs best, but what’s going on with the list icon type? Do we have too little data or is it interacting with the font size somehow? I find it a lot clearer when plotted:
library(ggplot2)
qplot(Size,Rate,color=Ul,data=rates)
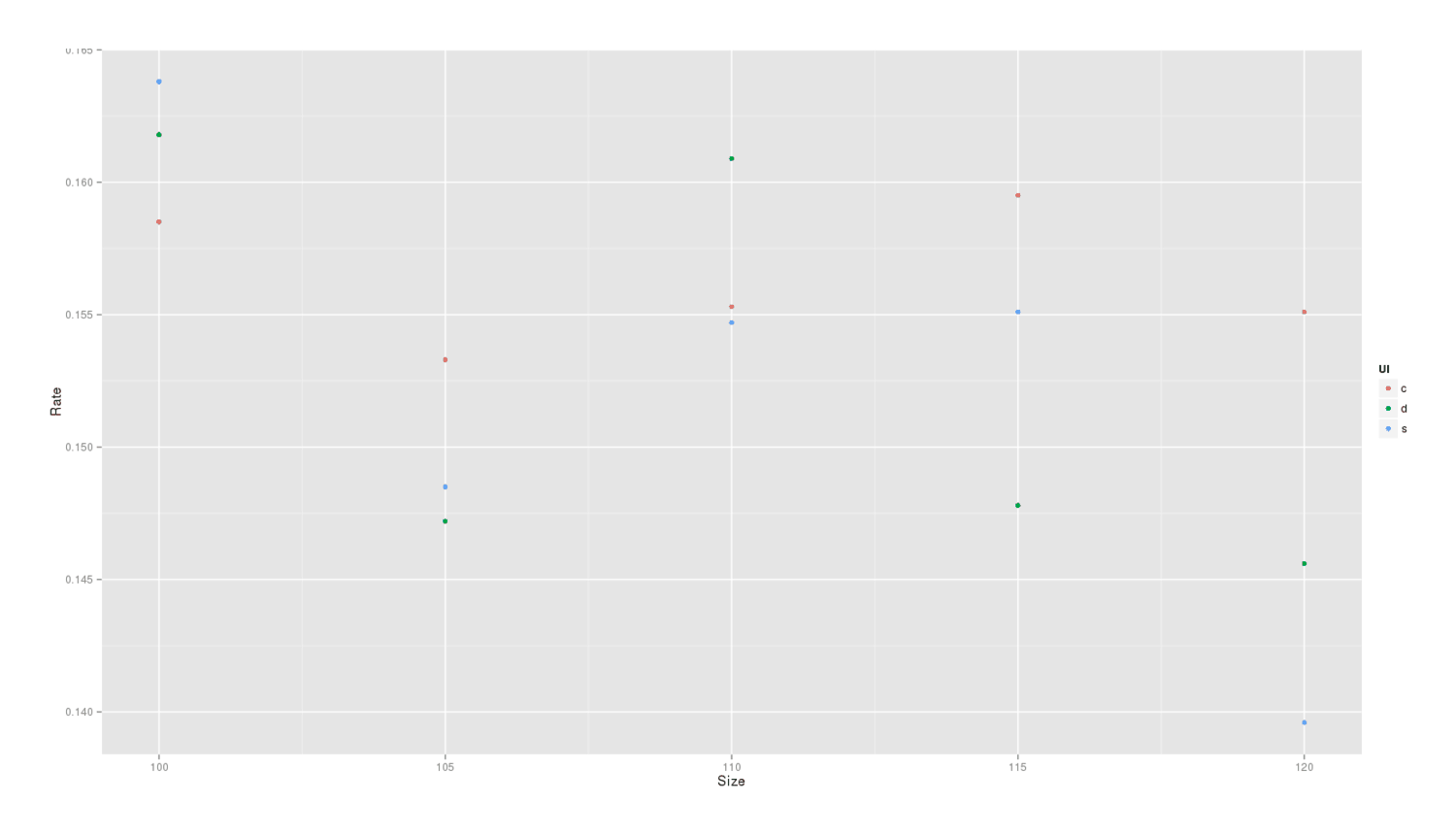
Immediately the negative effect of increasing the font size jumps out, but it’s easier to understand the list icon estimates: square performs the best in the 100% (the original default) font size condition but it performs poorly in the other font sizes, which is why it seems to do only medium-well compared to the others. Given how much better 100% performs than the others, I’m inclined to ignore their results and keep the squares.
100% and squares, however, were the original CSS settings, so this means I will make no changes to the existing CSS based on these results.
Blockquote Formatting
Another bit of formatting I’ve been meaning to test for a while is seeing how well Readability’s pull-quotes next to blockquotes perform, and to check whether my zebra-striping of nested blockquotes is helpful or harmful.
The Readability thing goes like this:
blockquote: : before {
content: "\201C";
filter: alpha(opacity=20);
font-family: "Constantia", Georgia, 'Hoefler Text', 'Times New Roman', serif;
font-size: 4em;
left: -0.5em;
opacity: .2;
position: absolute;
top: .25em }
The current blockquote striping goes thusly:
blockquote, blockquote blockquote blockquote,
blockquote blockquote blockquote blockquote blockquote {
z-index: -2;
background-color: rgb(245, 245, 245); }
blockquote blockquote, blockquote blockquote blockquote blockquote,
blockquote blockquote blockquote blockquote blockquote blockquote {
background-color: rgb(235, 235, 235); }
Implementation
This is another 2x2 design since we can use the Readability quotes or not, and the zebra-striping or not.
hunk ./static/css/default.css 271
-blockquote, blockquote blockquote blockquote,
- blockquote blockquote blockquote blockquote blockquote {
- z-index: -2;
- background-color: rgb(245, 245, 245); }
-blockquote blockquote, blockquote blockquote blockquote blockquote,
- blockquote blockquote blockquote blockquote blockquote blockquote {
- background-color: rgb(235, 235, 235); }
+/* blockquote, blockquote blockquote blockquote, */
+/* blockquote blockquote blockquote blockquote blockquote { */
+/* z-index: -2; */
+/* background-color: rgb(245, 245, 245); } */
+/* blockquote blockquote, blockquote blockquote blockquote blockquote, */
+/*blockquote blockquote blockquote blockquote blockquote blockquote { */
+/* background-color: rgb(235, 235, 235); } */
hunk ./static/template/default.html 30
- <div class="ulFontSize_class1"></div>
+ <div class="blockquoteFormatting_class1"></div>
hunk ./static/template/default.html 148
- ulFontSize: [
+ blockquoteFormatting: [
hunk ./static/template/default.html 150
- name: 's100',
- "ulFontSize_class1": "<style>ul { list-style-type: square; }; html { font-size: 100%; }</style>",
- "ulFontSize_class2": ""
+ name: 'rz',
+ "blockquoteFormatting_class1": "<style>blockquote: : before { content: '\201C';
filter: alpha(opacity=20);
font-family: 'Constantia', Georgia, 'Hoefler Text', 'Times New Roman', serif; font-size: 4em;left: -0.5em;
opacity: .2; position: absolute; top: .25em }; blockquote, blockquote blockquote blockquote,
blockquote blockquote blockquote blockquote blockquote { z-index: -2; background-color: rgb(245, 245, 245); };
blockquote blockquote, blockquote blockquote blockquote blockquote,
blockquote blockquote blockquote blockquote blockquote blockquote { background-color: rgb(235, 235, 235); }</style>",
+ "blockquoteFormatting_class2": ""
hunk ./static/template/default.html 155
- name: 's105',
- "ulFontSize_class1": "<style>ul { list-style-type: square; }; html { font-size: 105%; }</style>",
- "ulFontSize_class2": ""
+ name: 'orz',
+ "blockquoteFormatting_class1": "<style>blockquote, blockquote blockquote blockquote,
blockquote blockquote blockquote blockquote blockquote { z-index: -2; background-color: rgb(245, 245, 245); };
blockquote blockquote, blockquote blockquote blockquote blockquote,
blockquote blockquote blockquote blockquote blockquote blockquote { background-color: rgb(235, 235, 235); }</style>",
+ "blockquoteFormatting_class2": ""
hunk ./static/template/default.html 160
- name: 's110',
- "ulFontSize_class1": "<style>ul { list-style-type: square; }; html { font-size: 110%; }</style>",
- "ulFontSize_class2": ""
+ name: 'roz',
+ "blockquoteFormatting_class1": "<style>blockquote: : before { content: '\201C';
filter: alpha(opacity=20);
font-family: 'Constantia', Georgia, 'Hoefler Text', 'Times New Roman', serif; font-size: 4em;left: -0.5em;
opacity: .2; position: absolute; top: .25em }</style>",
+ "blockquoteFormatting_class2": ""
hunk ./static/template/default.html 165
- name: 's115',
- "ulFontSize_class1": "<style>ul { list-style-type: square; }; html { font-size: 115%; }</style>",
- "ulFontSize_class2": ""
- },
- {
- name: 's120',
- "ulFontSize_class1": "<style>ul { list-style-type: square; }; html { font-size: 120%; }</style>",
- "ulFontSize_class2": ""
- },
- {
- name: 'c100',
- "ulFontSize_class1": "<style>ul { list-style-type: circle; }; html { font-size: 100%; }</style>",
- "ulFontSize_class2": ""
- },
- {
- name: 'c105',
- "ulFontSize_class1": "<style>ul { list-style-type: circle; }; html { font-size: 105%; }</style>",
- "ulFontSize_class2": ""
- },
- {
- name: 'c110',
- "ulFontSize_class1": "<style>ul { list-style-type: circle; }; html { font-size: 110%; }</style>",
- "ulFontSize_class2": ""
- },
- {
- name: 'c115',
- "ulFontSize_class1": "<style>ul { list-style-type: circle; }; html { font-size: 115%; }</style>",
- "ulFontSize_class2": ""
- },
- {
- name: 'c120',
- "ulFontSize_class1": "<style>ul { list-style-type: circle; }; html { font-size: 120%; }</style>",
- "ulFontSize_class2": ""
- },
- {
- name: 'd100',
- "ulFontSize_class1": "<style>ul { list-style-type: disc; }; html { font-size: 100%; }</style>",
- "ulFontSize_class2": ""
- },
- {
- name: 'd105',
- "ulFontSize_class1": "<style>ul { list-style-type: disc; }; html { font-size: 105%; }</style>",
- "ulFontSize_class2": ""
- },
- {
- name: 'd110',
- "ulFontSize_class1": "<style>ul { list-style-type: disc; }; html { font-size: 110%; }</style>",
- "ulFontSize_class2": ""
- },
- {
- name: 'd115',
- "ulFontSize_class1": "<style>ul { list-style-type: disc; }; html { font-size: 115%; }</style>",
- "ulFontSize_class2": ""
- },
- {
- name: 'd120',
- "ulFontSize_class1": "<style>ul { list-style-type: disc; }; html { font-size: 120%; }</style>",
- "ulFontSize_class2": ""
+ name: 'oroz',
+ "blockquoteFormatting_class1": "<style></style>",
+ "blockquoteFormatting_class2": ""
... ]]
Data
Readability Quote |
Blockquote highlighting |
n |
Conversion Rate |
---|---|---|---|
no |
yes |
11,663 |
20.04% |
yes |
yes |
11,514 |
19.86% |
no |
no |
11,464 |
19.21% |
yes |
no |
10,669 |
18.51% |
45,310 |
19.42% |
I discovered during this experiment that I could graph the conversion rate of each condition separately:
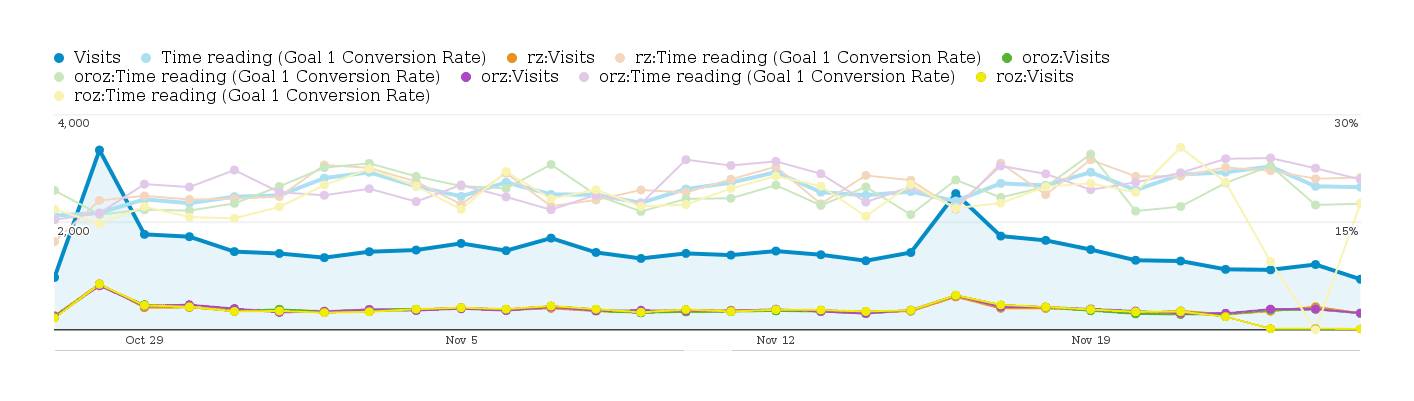
What I like about this graph is how it demonstrates some basic statistical points:
-
the more traffic, the smaller sampling error is and the closer the 4 conditions are to their true values as they cluster together. This illustrates how even what seems like a large difference based on a large amount of data, may still be - unintuitively - dominated by sampling error
-
day to day, any condition can be on top; no matter which one proves superior and which version is the worst, we can spot days where the worst version looks better than the best version. This illustrates how insidious selection biases or choice of datapoints can be: we can easily lie and show black is white, if we can just manage to cherrypick a little bit.
-
the underlying traffic does not itself appear to be completely stable or consistent. There are a lot of movements which look like the underlying visitors may be changing in composition slightly and responding slightly. This harks back to the paper’s warning that for some tests, no answer was possible as the responses of visitors kept changing which version was performing best.
Analysis
rates <- read.csv(stdin(),header=TRUE)
Readability,Zebra,Type,N,Rate
FALSE,FALSE,new,7191,0.1837
TRUE,TRUE,new,7182,0.1910
FALSE,TRUE,new,7112,0.1800
TRUE,FALSE,new,6508,0.1804
FALSE,TRUE,old,4652,0.2236
TRUE,FALSE,old,4452,0.1995
TRUE,TRUE,old,4412,0.2201
FALSE,FALSE,old,4374,0.2046
rates$Successes <- rates$N * rates$Rate
rates$Successes <- round(rates$Successes,0)
rates$Failures <-rates$N - rates$Successes
g <- glm(cbind(Successes,Failures) ~ Readability * Zebra + Type, data=rates, family="binomial"); summary(g)
# ...Coefficients:
# Estimate Std. Error z value Pr(>|z|)
# (Intercept) -1.5095 0.0255 -59.09 <2e-16
# ReadabilityTRUE -0.0277 0.0340 -0.81 0.42
# ZebraTRUE 0.0327 0.0331 0.99 0.32
# ReadabilityTRUE:ZebraTRUE 0.0609 0.0472 1.29 0.20
# Typeold 0.1788 0.0239 7.47 8e-14
summary(step(g))
# ...Coefficients:
# Estimate Std. Error z value Pr(>|z|)
# (Intercept) -1.5227 0.0197 -77.20 < 2e-16
# ZebraTRUE 0.0627 0.0236 2.66 0.0079
# Typeold 0.1782 0.0239 7.45 9.7e-14
The top-performing variant is the status quo (no Readability-style quote, zebra-striped blocks). So we keep it.
Font Size & ToC Background
It was pointed out to me that in my previous font-size test, the clear linear trend may have implied that larger fonts than 100% were bad, but that I was making an unjustified leap in implicitly assuming that 100% was best: if bigger is worse, then mightn’t the optimal font size be something smaller than 100%, like 95%?
And while the blockquote background coloring is a good idea, per the previous test, what about the other place on Gwern.net where I use a light background shading: the Table of Contents? Perhaps it would be better with the same background shading as the blockquotes, or no shading?
Finally, because I am tired of just 2 factors, I throw in a third factor to make it really multifactorial. I picked the number-sizing from the existing list of suggestions.
Each factor has 3 variants, giving 27 conditions:
.num { font-size: 85%; }
.num { font-size: 95%; }
.num { font-size: 100%; }
html { font-size: 85%; }
html { font-size: 95%; }
html { font-size: 100%; }
div#TOC { background: #fff; }
div#TOC { background: #eee; }
div#TOC { background-color: rgb(245, 245, 245); }
Implementation
hunk ./static/template/default.html 30
- <div class="blockquoteFormatting_class1"></div>
+ <div class="tocFormatting_class1"></div>
hunk ./static/template/default.html 150
- blockquoteFormatting: [
+ tocFormatting: [
hunk ./static/template/default.html 152
- name: 'rz',
- "blockquoteFormatting_class1": "<style>blockquote:before { display: block; font-size: 200%; color: #ccc; content: open-quote; height: 0px; margin-left: -0.55em; position:relative; }; blockquote blockquote, blockquote blockquote blockquote blockquote, blockquote blockquote blockquote blockquote blockquote blockquote { background-color: rgb(235, 235, 235); }</style>",
- "blockquoteFormatting_class2": ""
+ name: '88f',
+ "tocFormatting_class1": "<style>.num { font-size: 85%; }; html { font-size: 85%; }; div#TOC { background: #fff; };</style>",
+ "tocFormatting_class2": ""
hunk ./static/template/default.html 157
- name: 'orz',
- "blockquoteFormatting_class1": "<style>blockquote, blockquote blockquote blockquote, blockquote blockquote blockquote blockquote blockquote { z-index: -2; background-color: rgb(245, 245, 245); }; blockquote blockquote, blockquote blockquote blockquote blockquote, blockquote blockquote blockquote blockquote blockquote blockquote { background-color: rgb(235, 235, 235); }</style>",
- "blockquoteFormatting_class2": ""
+ name: '88e',
+ "tocFormatting_class1": "<style>.num { font-size: 85%; }; html { font-size: 85%; }; div#TOC { background: #eee; }</style>",
+ "tocFormatting_class2": ""
hunk ./static/template/default.html 162
- name: 'oroz',
- "blockquoteFormatting_class1": "<style></style>",
- "blockquoteFormatting_class2": ""
+ name: '88r',
+ "tocFormatting_class1": "<style>.num { font-size: 85%; }; html { font-size: 85%; }; div#TOC { background-color: rgb(245, 245, 245); }</style>",
+ "tocFormatting_class2": ""
+ },
+ {
+ name: '89f',
+ "tocFormatting_class1": "<style>.num { font-size: 85%; }; html { font-size: 95%; }; div#TOC { background: #fff; }</style>",
+ "tocFormatting_class2": ""
+ },
+ {
+ name: '89e',
+ "tocFormatting_class1": "<style>.num { font-size: 85%; }; html { font-size: 95%; }; div#TOC { background: #eee; }</style>",
+ "tocFormatting_class2": ""
+ },
+ {
+ name: '89f',
+ "tocFormatting_class1": "<style>.num { font-size: 85%; }; html { font-size: 95%; }; div#TOC { background-color: rgb(245, 245, 245); }</style>",
+ "tocFormatting_class2": ""
+ },
+ {
+ name: '81f',
+ "tocFormatting_class1": "<style>.num { font-size: 85%; }; html { font-size: 100%; }; div#TOC { background: #fff; }</style>",
+ "tocFormatting_class2": ""
+ },
+ {
+ name: '81e',
+ "tocFormatting_class1": "<style>.num { font-size: 85%; }; html { font-size: 100%; }; div#TOC { background: #eee; }</style>",
+ "tocFormatting_class2": ""
+ },
+ {
+ name: '81r',
+ "tocFormatting_class1": "<style>.num { font-size: 85%; }; html { font-size: 100%; }; div#TOC { background-color: rgb(245, 245, 245); }</style>",
+ "tocFormatting_class2": ""
+ },
+ {
+ name: '98f',
+ "tocFormatting_class1": "<style>.num { font-size: 95%; }; html { font-size: 85%; }; div#TOC { background: #fff; };</style>",
+ "tocFormatting_class2": ""
+ },
+ {
+ name: '98e',
+ "tocFormatting_class1": "<style>.num { font-size: 95%; }; html { font-size: 85%; }; div#TOC { background: #eee; }</style>",
+ "tocFormatting_class2": ""
+ },
+ {
+ name: '98r',
+ "tocFormatting_class1": "<style>.num { font-size: 95%; }; html { font-size: 85%; }; div#TOC { background-color: rgb(245, 245, 245); }</style>",
+ "tocFormatting_class2": ""
+ },
+ {
+ name: '99f',
+ "tocFormatting_class1": "<style>.num { font-size: 95%; }; html { font-size: 95%; }; div#TOC { background: #fff; }</style>",
+ "tocFormatting_class2": ""
+ },
+ {
+ name: '99e',
+ "tocFormatting_class1": "<style>.num { font-size: 95%; }; html { font-size: 95%; }; div#TOC { background: #eee; }</style>",
+ "tocFormatting_class2": ""
+ },
+ {
+ name: '99f',
+ "tocFormatting_class1": "<style>.num { font-size: 95%; }; html { font-size: 95%; }; div#TOC { background-color: rgb(245, 245, 245); }</style>",
+ "tocFormatting_class2": ""
+ },
+ {
+ name: '91f',
+ "tocFormatting_class1": "<style>.num { font-size: 95%; }; html { font-size: 100%; }; div#TOC { background: #fff; }</style>",
+ "tocFormatting_class2": ""
+ },
+ {
+ name: '91e',
+ "tocFormatting_class1": "<style>.num { font-size: 95%; }; html { font-size: 100%; }; div#TOC { background: #eee; }</style>",
+ "tocFormatting_class2": ""
+ },
+ {
+ name: '91r',
+ "tocFormatting_class1": "<style>.num { font-size: 95%; }; html { font-size: 100%; }; div#TOC { background-color: rgb(245, 245, 245); }</style>",
+ "tocFormatting_class2": ""
+ },
+ {
+ name: '18f',
+ "tocFormatting_class1": "<style>.num { font-size: 100%; }; html { font-size: 85%; }; div#TOC { background: #fff; };</style>",
+ "tocFormatting_class2": ""
+ },
+ {
+ name: '18e',
+ "tocFormatting_class1": "<style>.num { font-size: 100%; }; html { font-size: 85%; }; div#TOC { background: #eee; }</style>",
+ "tocFormatting_class2": ""
+ },
+ {
+ name: '18r',
+ "tocFormatting_class1": "<style>.num { font-size: 100%; }; html { font-size: 85%; }; div#TOC { background-color: rgb(245, 245, 245); }</style>",
+ "tocFormatting_class2": ""
+ },
+ {
+ name: '19f',
+ "tocFormatting_class1": "<style>.num { font-size: 100%; }; html { font-size: 95%; }; div#TOC { background: #fff; }</style>",
+ "tocFormatting_class2": ""
+ },
+ {
+ name: '19e',
+ "tocFormatting_class1": "<style>.num { font-size: 100%; }; html { font-size: 95%; }; div#TOC { background: #eee; }</style>",
+ "tocFormatting_class2": ""
+ },
+ {
+ name: '19f',
+ "tocFormatting_class1": "<style>.num { font-size: 100%; }; html { font-size: 95%; }; div#TOC { background-color: rgb(245, 245, 245); }</style>",
+ "tocFormatting_class2": ""
+ },
+ {
+ name: '11f',
+ "tocFormatting_class1": "<style>.num { font-size: 100%; }; html { font-size: 100%; }; div#TOC { background: #fff; }</style>",
+ "tocFormatting_class2": ""
+ },
+ {
+ name: '11e',
+ "tocFormatting_class1": "<style>.num { font-size: 100%; }; html { font-size: 100%; }; div#TOC { background: #eee; }</style>",
+ "tocFormatting_class2": ""
+ },
+ {
+ name: '11r',
+ "tocFormatting_class1": "<style>.num { font-size: 100%; }; html { font-size: 100%; }; div#TOC { background-color: rgb(245, 245, 245); }</style>",
+ "tocFormatting_class2": ""
... ]]
Analysis
rates <- read.csv(stdin(),header=TRUE)
NumSize,FontSize,TocBg,Type,N,Rate
1,9,e,new,3060,0.1513
8,9,e,new,2978,0.1605
9,1,r,new,2965,0.1548
8,8,f,new,2941,0.1629
1,9,f,new,2933,0.1558
9,9,r,new,2932,0.1576
8,9,f,new,2906,0.1473
1,9,r,new,2901,0.1482
9,9,f,new,2901,0.1420
8,8,r,new,2885,0.1567
1,8,e,new,2876,0.1412
8,1,r,new,2869,0.1593
9,8,f,new,2846,0.1472
1,1,e,new,2844,0.1551
1,8,f,new,2841,0.1457
9,8,e,new,2834,0.1478
8,1,f,new,2833,0.1521
1,8,r,new,2818,0.1544
8,8,e,new,2818,0.1678
8,1,e,new,2810,0.1605
1,1,r,new,2806,0.1775
9,8,r,new,2801,0.1682
9,1,e,new,2799,0.1422
8,9,r,new,2764,0.1548
9,9,e,new,2753,0.1478
1,1,f,new,2750,0.1611
9,1,f,new,2700,0.1537
8,8,r,old,1551,0.2521
9,8,e,old,1519,0.2146
9,8,f,old,1505,0.2153
1,8,e,old,1489,0.2317
1,1,e,old,1475,0.2339
8,1,f,old,1416,0.2112
1,9,r,old,1390,0.2245
8,9,e,old,1388,0.2464
9,9,r,old,1379,0.2466
8,9,r,old,1374,0.1907
1,9,f,old,1361,0.2337
8,8,f,old,1348,0.2322
1,9,e,old,1347,0.2279
1,8,f,old,1340,0.2470
9,1,r,old,1336,0.2605
8,1,r,old,1326,0.2119
8,8,e,old,1321,0.2286
9,1,f,old,1318,0.2398
1,1,r,old,1293,0.2111
1,8,r,old,1293,0.2073
9,9,f,old,1261,0.2411
8,9,f,old,1254,0.2113
9,9,e,old,1240,0.2435
1,1,f,old,1232,0.2240
8,1,e,old,1229,0.2587
9,1,e,old,1182,0.2335
9,8,r,old,1032,0.2403
rates[rates$NumSize==1,]$NumSize <- 100
rates[rates$NumSize==9,]$NumSize <- 95
rates[rates$NumSize==8,]$NumSize <- 85
rates[rates$FontSize==1,]$FontSize <- 100
rates[rates$FontSize==9,]$FontSize <- 95
rates[rates$FontSize==8,]$FontSize <- 85
rates$Successes <- rates$N * rates$Rate
rates$Successes <- round(rates$Successes,0)
rates$Failures <-rates$N - rates$Successes
g <- glm(cbind(Successes,Failures) ~ NumSize * FontSize * TocBg + Type, data=rates, family="binomial"); summary(g)
# ...Coefficients:
# Estimate Std. Error z value Pr(>|z|)
# (Intercept) 0.124770 3.020334 0.04 0.97
# NumSize -0.022262 0.032293 -0.69 0.49
# FontSize -0.012775 0.032283 -0.40 0.69
# TocBgf 4.042812 4.287006 0.94 0.35
# TocBgr 5.356794 4.250778 1.26 0.21
# NumSize:FontSize 0.000166 0.000345 0.48 0.63
# NumSize:TocBgf -0.040645 0.045855 -0.89 0.38
# NumSize:TocBgr -0.054164 0.045501 -1.19 0.23
# FontSize:TocBgf -0.052406 0.045854 -1.14 0.25
# FontSize:TocBgr -0.065503 0.045482 -1.44 0.15
# NumSize:FontSize:TocBgf 0.000531 0.000490 1.08 0.28
# NumSize:FontSize:TocBgr 0.000669 0.000487 1.37 0.17
# Typeold 0.492688 0.015978 30.84 <2e-16
summary(step(g))
# ...Coefficients:
# Estimate Std. Error z value Pr(>|z|)
# (Intercept) 3.808438 1.750144 2.18 0.0295
# NumSize -0.059730 0.018731 -3.19 0.0014
# FontSize -0.052262 0.018640 -2.80 0.0051
# TocBgf -0.844664 0.285387 -2.96 0.0031
# TocBgr -0.747451 0.283304 -2.64 0.0083
# NumSize:FontSize 0.000568 0.000199 2.85 0.0044
# NumSize:TocBgf 0.008853 0.003052 2.90 0.0037
# NumSize:TocBgr 0.008139 0.003030 2.69 0.0072
# Typeold 0.492598 0.015975 30.83 <2e-16
The two size tweaks turn out to be unambiguously negative compared to the status quo (with an almost negligible interaction term probably reflecting reader preference for consistency in sizes of letters and numbers - as one gets smaller, the other does better if it’s smaller too). The Table of Contents backgrounds also survive (thanks to the new vs old visitor type covariate adding power): there were 3 background types, e
/f
/r
[gb], and f
/r
turn out to have negative coefficients, implying that e
is best - but e
is also the status quo, so no change is recommended.
Multifactorial Roundup
At this point it seems worth asking whether running multifactorials has been worthwhile. The analysis is a bit more difficult, and the more factors there are, the harder to interpret. I’m also not too keen on encoding the combinatorial explosion into a big JS array for ABalytics. In my tests so far, have there been many interactions? A quick tally of the glm()
/step()
results:
-
Text & background color:
-
original: 2 main, 1 two-way interaction
-
survived: 2 main, 1 two-way interaction
-
-
List symbol and font-size:
-
original: 3 main, 2 two-way interactions
-
survived: 1 main
-
-
Blockquote formatting:
-
original: 2 main, 1 two-way
-
survived: 1 main
-
-
Font size & ToC background:
-
original: 4 mains, 5 two-ways, 2 three-ways
-
survived: 3 mains, 2 two-way
-
So of the 11 main effects, 9 two-ways, & 2 three-ways, there were confirmed in the reduced models: 7 mains, 3 two-ways (22%), & 0 three-ways (0%). And of the 2 interactions, only the black/white interaction was important (and even there, if I had regressed instead cbind(Successes, Failures) ~ Black + White
, black & white would still have positive coefficients, they just would not be statistically-significant, and so I would likely have made the same choice as I did with the interaction data available).
This is not a resounding endorsement so far.
Section Header Capitalization
3x3:
-
h1, h2, h3, h4, h5 { text-transform: uppercase; }
-
h1, h2, h3, h4, h5 { text-transform: none; }
-
h1, h2, h3, h4, h5 { text-transform: capitalize; }
-
div#header h1 { text-transform: uppercase; }
-
div#header h1 { text-transform: none; }
-
div#header h1 { text-transform: capitalize; }
--- a/static/template/default.html
+++ b/static/template/default.html
@@ -27,7 +27,7 @@
<body>
- <div class="tocFormatting_class1"></div>
+ <div class="headerCaps_class1"></div>
<div id="main">
<div id="sidebar">
@@ -152,141 +152,51 @@
_gaq.push(['_setAccount', 'UA-18912926-1']);
ABalytics.init({
- tocFormatting: [
+ headerCaps: [
{
- name: '88f',
- "tocFormatting_class1": "<style>.num { font-size: 85%; }; html { font-size: 85%; }; div#TOC { background: #fff; };</style>",
- "tocFormatting_class2": ""
+ name: 'uu',
+ "headerCaps_class1": "<style>h1, h2, h3, h4, h5 { text-transform: uppercase; }; div#header h1 { text-transform: uppercase; }</style>",
+ "headerCaps_class2": ""
},
{
- name: '88e',
- "tocFormatting_class1": "<style>.num { font-size: 85%; }; html { font-size: 85%; }; div#TOC { background: #eee; }</style>",
- "tocFormatting_class2": ""
+ name: 'un',
+ "headerCaps_class1": "<style>div#header h1 { text-transform: uppercase; }; div#header h1 { text-transform: none; }</style>",
+ "headerCaps_class2": ""
},
{
- name: '88r',
- "tocFormatting_class1": "<style>.num { font-size: 85%; }; html { font-size: 85%; }; div#TOC { background-color: rgb(245, 245, 245); }</style>",
- "tocFormatting_class2": ""
+ name: 'uc',
+ "headerCaps_class1": "<style>div#header h1 { text-transform: uppercase; }; div#header h1 { text-transform: capitalize; }</style>",
+ "headerCaps_class2": ""
},
{
- name: '89f',
- "tocFormatting_class1": "<style>.num { font-size: 85%; }; html { font-size: 95%; }; div#TOC { background: #fff; }</style>",
- "tocFormatting_class2": ""
+ name: 'nu',
+ "headerCaps_class1": "<style>h1, h2, h3, h4, h5 { text-transform: none; }; div#header h1 { text-transform: uppercase; }</style>",
+ "headerCaps_class2": ""
},
{
- name: '89e',
- "tocFormatting_class1": "<style>.num { font-size: 85%; }; html { font-size: 95%; }; div#TOC { background: #eee; }</style>",
- "tocFormatting_class2": ""
+ name: 'nn',
+ "headerCaps_class1": "<style>h1, h2, h3, h4, h5 { text-transform: none; }; div#header h1 { text-transform: none; }</style>",
+ "headerCaps_class2": ""
},
{
- name: '89r',
- "tocFormatting_class1": "<style>.num { font-size: 85%; }; html { font-size: 95%; }; div#TOC { background-color: rgb(245, 245, 245); }</style>",
- "tocFormatting_class2": ""
+ name: 'nc',
+ "headerCaps_class1": "<style>h1, h2, h3, h4, h5 { text-transform: none; }; div#header h1 { text-transform: capitalize; }</style>",
+ "headerCaps_class2": ""
},
{
- name: '81f',
- "tocFormatting_class1": "<style>.num { font-size: 85%; }; html { font-size: 100%; }; div#TOC { background: #fff; }</style>",
- "tocFormatting_class2": ""
+ name: 'cu',
+ "headerCaps_class1": "<style>h1, h2, h3, h4, h5 { text-transform: capitalize; }; div#header h1 { text-transform: uppercase; }</style>",
+ "headerCaps_class2": ""
},
{
- name: '81e',
- "tocFormatting_class1": "<style>.num { font-size: 85%; }; html { font-size: 100%; }; div#TOC { background: #eee; }</style>",
- "tocFormatting_class2": ""
+ name: 'cn',
+ "headerCaps_class1": "<style>h1, h2, h3, h4, h5 { text-transform: capitalize; }; div#header h1 { text-transform: none; }</style>",
+ "headerCaps_class2": ""
},
{
- name: '81r',
- "tocFormatting_class1": "<style>.num { font-size: 85%; }; html { font-size: 100%; }; div#TOC { background-color: rgb(245, 245, 245); }</style>",
- "tocFormatting_class2": ""
- },
- {
- name: '98f',
- "tocFormatting_class1": "<style>.num { font-size: 95%; }; html { font-size: 85%; }; div#TOC { background: #fff; };</style>",
- "tocFormatting_class2": ""
- },
- {
- name: '98e',
- "tocFormatting_class1": "<style>.num { font-size: 95%; }; html { font-size: 85%; }; div#TOC { background: #eee; }</style>",
- "tocFormatting_class2": ""
- },
- {
- name: '98r',
- "tocFormatting_class1": "<style>.num { font-size: 95%; }; html { font-size: 85%; }; div#TOC { background-color: rgb(245, 245, 245); }</style>",
- "tocFormatting_class2": ""
- },
- {
- name: '99f',
- "tocFormatting_class1": "<style>.num { font-size: 95%; }; html { font-size: 95%; }; div#TOC { background: #fff; }</style>",
- "tocFormatting_class2": ""
- },
- {
- name: '99e',
- "tocFormatting_class1": "<style>.num { font-size: 95%; }; html { font-size: 95%; }; div#TOC { background: #eee; }</style>",
- "tocFormatting_class2": ""
- },
- {
- name: '99r',
- "tocFormatting_class1": "<style>.num { font-size: 95%; }; html { font-size: 95%; }; div#TOC { background-color: rgb(245, 245, 245); }</style>",
- "tocFormatting_class2": ""
- },
- {
- name: '91f',
- "tocFormatting_class1": "<style>.num { font-size: 95%; }; html { font-size: 100%; }; div#TOC { background: #fff; }</style>",
- "tocFormatting_class2": ""
- },
- {
- name: '91e',
- "tocFormatting_class1": "<style>.num { font-size: 95%; }; html { font-size: 100%; }; div#TOC { background: #eee; }</style>",
- "tocFormatting_class2": ""
- },
- {
- name: '91r',
- "tocFormatting_class1": "<style>.num { font-size: 95%; }; html { font-size: 100%; }; div#TOC { background-color: rgb(245, 245, 245); }</style>",
- "tocFormatting_class2": ""
- },
- {
- name: '18f',
- "tocFormatting_class1": "<style>.num { font-size: 100%; }; html { font-size: 85%; }; div#TOC { background: #fff; };</style>",
- "tocFormatting_class2": ""
- {
- name: '18e',
- "tocFormatting_class1": "<style>.num { font-size: 100%; }; html { font-size: 85%; }; div#TOC { background: #eee; }</style>",
- "tocFormatting_class2": ""
- },
- {
- name: '18r',
- "tocFormatting_class1": "<style>.num { font-size: 100%; }; html { font-size: 85%; }; div#TOC { background-color: rgb(245, 245, 245); }</style>",
- "tocFormatting_class2": ""
- },
- {
- name: '19f',
- "tocFormatting_class1": "<style>.num { font-size: 100%; }; html { font-size: 95%; }; div#TOC { background: #fff; }</style>",
- "tocFormatting_class2": ""
- },
- {
- name: '19e',
- "tocFormatting_class1": "<style>.num { font-size: 100%; }; html { font-size: 95%; }; div#TOC { background: #eee; }</style>",
- "tocFormatting_class2": ""
- },
- {
- name: '19r',
- "tocFormatting_class1": "<style>.num { font-size: 100%; }; html { font-size: 95%; }; div#TOC { background-color: rgb(245, 245, 245); }</style>",
- "tocFormatting_class2": ""
- },
- {
- name: '11f',
- "tocFormatting_class1": "<style>.num { font-size: 100%; }; html { font-size: 100%; }; div#TOC { background: #fff; }</style>",
- "tocFormatting_class2": ""
- },
- {
- name: '11e',
- "tocFormatting_class1": "<style>.num { font-size: 100%; }; html { font-size: 100%; }; div#TOC { background: #eee; }</style>",
- "tocFormatting_class2": ""
- },
- {
- name: '11r',
- "tocFormatting_class1": "<style>.num { font-size: 100%; }; html { font-size: 100%; }; div#TOC { background-color: rgb(245, 245, 245); }</style>",
- "tocFormatting_class2": ""
+ name: 'cc',
+ "headerCaps_class1": "<style>h1, h2, h3, h4, h5 { text-transform: capitalize; }; div#header h1 { text-transform: capitalize; }</style>",
+ "headerCaps_class2": ""
}
],
}, _gaq);
...)}
rates <- read.csv(stdin(),header=TRUE)
Sections,Title,Old,N,Rate
c,u,FALSE,2362, 0.1808
c,n,FALSE,2356,0.1855
c,c,FALSE,2342,0.2003
u,u,FALSE,2341,0.1965
u,c,FALSE,2333,0.1989
n,u,FALSE,2329,0.1928
n,c,FALSE,2323,0.1941
n,n,FALSE,2321,0.1978
u,n,FALSE,2315,0.1965
c,c,TRUE,1370,0.2190
n,u,TRUE,1302,0.2558
u,u,TRUE,1271,0.2919
c,n,TRUE,1258,0.2377
u,c,TRUE,1228,0.2272
n,c,TRUE,1211,0.2337
n,n,TRUE,1200,0.2400
c,u,TRUE,1135,0.2396
u,n,TRUE,1028,0.2442
rates$Successes <- rates$N * rates$Rate
rates$Successes <- round(rates$Successes,0)
rates$Failures <-rates$N - rates$Successes
g <- glm(cbind(Successes,Failures) ~ Sections * Title + Old, data=rates, family="binomial"); summary(g)
# ...Coefficients:
# (Intercept) -1.4552 0.0422 -34.50 <2e-16
# Sectionsn 0.0111 0.0581 0.19 0.848
# Sectionsu 0.0163 0.0579 0.28 0.779
# Titlen -0.0153 0.0579 -0.26 0.791
# Titleu -0.0318 0.0587 -0.54 0.588
# OldTRUE 0.2909 0.0283 10.29 <2e-16
# Sectionsn:Titlen 0.0429 0.0824 0.52 0.603
# Sectionsu:Titlen 0.0419 0.0829 0.51 0.613
# Sectionsn:Titleu 0.0732 0.0825 0.89 0.375
# Sectionsu:Titleu 0.1553 0.0820 1.89 0.058
summary(step(g))
# ...Coefficients:
# Estimate Std. Error z value Pr(>|z|)
# (Intercept) -1.4710 0.0263 -55.95 <2e-16
# Sectionsn 0.0497 0.0337 1.47 0.140
# Sectionsu 0.0833 0.0337 2.47 0.013
# OldTRUE 0.2920 0.0283 10.33 <2e-16
Uppercase and ‘none’ beat ‘capitalize’ in both page titles & section headers (interaction does not survive). So I toss in a CSS declaration to uppercase section headers as well as the status quo of the title.
ToC Formatting
After the page title, the next thing a reader will generally see on my pages in the table of contents. It’s been tweaked over the years (particularly by suggestions from Hacker News) but still has some untested aspects, particularly the first two parts of div#TOC
:
float: left;
width: 25%;
I’d like to test left vs right, and 15,20,25,30,35%, so that’s a 2x5 design. Usual implementation:
diff --git a/static/template/default.html b/static/template/default.html
index 83c6f9c..11c4ada 100644
--- a/static/template/default.html
+++ b/static/template/default.html
@@ -27,7 +27,7 @@
<body>
- <div class="headerCaps_class1"></div>
+ <div class="tocAlign_class1"></div>
<div id="main">
<div id="sidebar">
@@ -152,51 +152,56 @@
_gaq.push(['_setAccount', 'UA-18912926-1']);
ABalytics.init({
- headerCaps: [
+ tocAlign: [
{
- name: 'uu',
- "headerCaps_class1": "<style>h1, h2, h3, h4, h5 { text-transform: uppercase; }; div#header h1 { text-transform: uppercase; }</style>",
- "headerCaps_class2": ""
+ name: 'l15',
+ "tocAlign_class1": "<style>div#TOC { float: left; width: 15%; }</style>",
+ "tocAlign_class2": ""
},
{
- name: 'un',
- "headerCaps_class1": "<style>div#header h1 { text-transform: uppercase; }; div#header h1 { text-transform: none; }</style>",
- "headerCaps_class2": ""
+ name: 'l20',
+ "tocAlign_class1": "<style>div#TOC { float: left; width: 20%; }</style>",
+ "tocAlign_class2": ""
},
{
- name: 'uc',
- "headerCaps_class1": "<style>div#header h1 { text-transform: uppercase; }; div#header h1 { text-transform: capitalize; }</style>",
- "headerCaps_class2": ""
+ name: 'l25',
+ "tocAlign_class1": "<style>div#TOC { float: left; width: 25%; }</style>",
+ "tocAlign_class2": ""
},
{
- name: 'nu',
- "headerCaps_class1": "<style>h1, h2, h3, h4, h5 { text-transform: none; }; div#header h1 { text-transform: uppercase; }</style>",
- "headerCaps_class2": ""
+ name: 'l30',
+ "tocAlign_class1": "<style>div#TOC { float: left; width: 30%; }</style>",
+ "tocAlign_class2": ""
},
{
- name: 'nn',
- "headerCaps_class1": "<style>h1, h2, h3, h4, h5 { text-transform: none; }; div#header h1 { text-transform: none; }</style>",
- "headerCaps_class2": ""
+ name: 'l35',
+ "tocAlign_class1": "<style>div#TOC { float: left; width: 35%; }</style>",
+ "tocAlign_class2": ""
},
{
- name: 'nc',
- "headerCaps_class1": "<style>h1, h2, h3, h4, h5 { text-transform: none; }; div#header h1 { text-transform: capitalize; }</style>",
- "headerCaps_class2": ""
+ name: 'r15',
+ "tocAlign_class1": "<style>div#TOC { float: right; width: 15%; }</style>",
+ "tocAlign_class2": ""
},
{
- name: 'cu',
- "headerCaps_class1": "<style>h1, h2, h3, h4, h5 { text-transform: capitalize; }; div#header h1 { text-transform: uppercase; }</style>",
- "headerCaps_class2": ""
+ name: 'r20',
+ "tocAlign_class1": "<style>div#TOC { float: right; width: 20%; }</style>",
+ "tocAlign_class2": ""
},
{
- name: 'cn',
- "headerCaps_class1": "<style>h1, h2, h3, h4, h5 { text-transform: capitalize; }; div#header h1 { text-transform: none; }</style>",
- "headerCaps_class2": ""
+ name: 'r25',
+ "tocAlign_class1": "<style>div#TOC { float: right; width: 25%; }</style>",
+ "tocAlign_class2": ""
},
{
- name: 'cc',
- "headerCaps_class1": "<style>h1, h2, h3, h4, h5 { text-transform: capitalize; }; div#header h1 { text-transform: capitalize; }</style>",
- "headerCaps_class2": ""
+ name: 'r30',
+ "tocAlign_class1": "<style>div#TOC { float: right; width: 30%; }</style>",
+ "tocAlign_class2": ""
+ },
+ {
+ name: 'r35',
+ "tocAlign_class1": "<style>div#TOC { float: right; width: 35%; }</style>",
+ "tocAlign_class2": ""
}
],
}, _gaq));
I decided to end this test early on 2014-03-10 because I wanted to move onto the BeeLine Reader test, so it’s underpowered & the results aren’t as clear as usual:
rates <- read.csv(stdin(),header=TRUE)
Alignment,Width,Old,N,Rate
r,25,FALSE,1040,0.1673
r,30,FALSE,1026,0.1891
l,20,FALSE,1023,0.1896
l,25,FALSE,1022,0.1800
l,35,FALSE,1022,0.1820
l,30,FALSE,1016,0.1781
l,15,FALSE,1010,0.1851
r,15,FALSE,991,0.1554
r,20,FALSE,989,0.1881
r,35,FALSE,969,0.1672
l,30,TRUE,584,0.2414
l,25,TRUE,553,0.2224
l,20,TRUE,520,0.3096
r,15,TRUE,512,0.2539
l,35,TRUE,496,0.2520
r,25,TRUE,494,0.2105
l,15,TRUE,482,0.2282
r,35,TRUE,480,0.2417
r,20,TRUE,460,0.2326
r,30,TRUE,455,0.2549
rates$Successes <- rates$N * rates$Rate
rates$Successes <- round(rates$Successes,0)
rates$Failures <-rates$N - rates$Successes
g <- glm(cbind(Successes,Failures) ~ Alignment * Width + Old, data=rates, family="binomial"); summary(g)
# Coefficients:
# Estimate Std. Error z value Pr(>|z|)
# (Intercept) -1.43309 0.10583 -13.54 <2e-16
# Alignmentr -0.17726 0.15065 -1.18 0.24
# Width -0.00253 0.00403 -0.63 0.53
# OldTRUE 0.40092 0.04184 9.58 <2e-16
# Alignmentr:Width 0.00450 0.00580 0.78 0.44
So, as I expected, putting the ToC on the right performed worse; the larger ToC widths don’t seem to be better but it’s unclear what’s going on there. A visual inspection of the Width data (library(ggplot2); qplot(Width,Rate,color=Alignment,data=rates)
) suggests that 20% width was the best variant, so might as well go with that.
BeeLine Reader Text Highlighting
BLR is a JS library for highlighting textual paragraphs with pairs of half-lines to make reading easier. I run a randomized experiment on several differently-colored versions to see if default site-wide usage of BLR will improve time-on-page for Gwern.net readers, indicating easier reading of the long-form textual content. Most versions perform worse than the control of no-highlighting; the best version performs slightly better but the improvement is not statistically-significant.
BeeLine Reader (BLR) is an interesting new browser plugin which launched around October 2013; I learned of it from the Hacker News discussion. The idea is that part of the difficulty in reading text is that when one finishes a line and saccades left to the continuation of the next line, the uncertainty of where it is adds a bit of stress, so one can make reading easier by adding some sort of guide to the next line; in this case, each matching pair of half-lines is colored differently, so if you are on a red half-line, when you saccade left, you look for a line also colored red, then you switch to blue in the middle of that line, and so on. A colorful variant on boustrophedon writing. I found the default BLR coloring garish & distracting, but I couldn’t see any reason that a subtle gray variant would not help: the idea seems plausible. And very long text pages (like mine) are where BLR should shine most.
I asked if there were a JavaScript version I could use in an A/B test; the initial JS implementation was not fast enough, but by 2014-03-10 it was good enough. BLR has several themes, including “gray”; I decided to test the variants no BLR, “dark”, “blues”, & expanded the gray selection to include grays #222222
/#333333
/#444444
/#555555
/#666666
/#777777
(gray
-6
; they vary in how blatant the highlighting is) for a total of 9 equally-randomized variants.
Since I’m particularly interested in these results, and I think many other people will find the results interesting, I will run this test extra-long: a minimum of 2 months. I’m only interested in the best variant, not estimating each variant exactly (what do I care if the ugly dark
is 15% rather than 14%? I just want to know it’s worse than the control) so conceptually I want something like a sequential analysis or adaptive clinical trial or multi-armed bandit where bad variants get dropped over time; unfortunately, I haven’t studied them yet (and MABs would be hard to implement on a static site), so I’ll just ad hoc drop the worst variant every week or two. (Maybe next experiment I’ll do a formal adaptive trial.)
Setup
The usual implementation using ABalytics doesn’t work because it uses a innerHTML
call to substitute the various fragments, and while HTML & CSS get interpreted fine, JavaScript does not; the offered solutions were sufficiently baroque I wound up implementing a custom subset of ABalytics hardwired for BLR inside the Analytics script:
<script id="googleAnalytics" type="text/javascript">
var _gaq = _gaq || [];
_gaq.push(['_setAccount', 'UA-18912926-1']);
+ // A/B test: heavily based on ABalytics
+ function readCookie (name) {
+ var nameEQ = name + "=";
+ var ca = document.cookie.split(';');
+ for(var i=0;i < ca.length;i++) {
+ var c = ca[i];
+ while (c.charAt(0)==' ') c = c.substring(1,c.length);
+ if (c.indexOf(nameEQ) == 0) return c.substring(nameEQ.length,c.length);
+ }
+ return null;
+ }
+
+ if (typeof(start_slot) == 'undefined') start_slot = 1;
+ var experiment = "blr3";
+ var variant_names = ["none", "dark", "blues", "gray1", "gray2", "gray3", "gray4", "gray5", "gray6"];
+
+ var variant_id = this.readCookie("ABalytics_"+experiment);
+ if (!variant_id || !variant_names[variant_id]) {
+ var variant_id = Math.floor(Math.random()*variant_names.length);
+ document.cookie = "ABalytics_"+experiment+"="+variant_id+"; path=/";
+ }
+ function beelinefy (COLOR) {
+ if (COLOR != "none") {
+ var elements=document.querySelectorAll("#content");
+ for(var i=0;i < elements.length;i++) {
+ var beeline=new BeeLineReader(elements[i], { theme: COLOR, skipBackgroundColor: true, skipTags: ['math', 'svg', 'h1', 'h2', 'h3', 'h4'] });
+ beeline.color();
+ }
+ }
+ }
+ beelinefy(variant_names[variant_id]);
+ _gaq.push(['_setCustomVar',
+ start_slot,
+ experiment, // The name of the custom variable = name of the experiment
+ variant_names[variant_id], // The value of the custom variable = variant shown
+ 2 // Sets the scope to session-level
+ ]);
_gaq.push(['_trackPageview']);
The themes are defined in beeline.min.js
as:
r.THEMES={
dark: ["#000000","#970000","#000000","#00057F","#FBFBFB"],
blues:["#000000","#0000FF","#000000","#840DD2","#FBFBFB"],
gray1:["#000000","#222222","#000000","#222222","#FBFBFB"],
gray2:["#000000","#333333","#000000","#333333","#FBFBFB"],
gray3:["#000000","#444444","#000000","#444444","#FBFBFB"],
gray4:["#000000","#555555","#000000","#555555","#FBFBFB"],
gray5:["#000000","#666666","#000000","#666666","#FBFBFB"],
gray6:["#000000","#777777","#000000","#777777","#FBFBFB"]
}
(Why “bl3”? I don’t know JS, so it took some time; things I learned along the line included always leaving whitespace around a <
operator, and that the “none” argument passed into beeline.setOptions
causes a problem which some browsers will ignore and continue recording A/B data after but most browsers will not; this broke the original test. Then I discovered that BLR by default broke all the MathML/MathJax, causing nasty-looking errors over pages with math expressions; this broke the second test, and I had to get a fixed version.)
Data
On 31 March, with total n having reached 15652 visits, I deleted the worst-performing variant: gray4
, which at 19.21% was substantially underperforming the best-performing variant’s 22.38%, and wasting traffic. On 6 April, two Hacker News submissions having doubled visits to 36533, I deleted the next-worst variant, gray5
(14.66% vs control of 16.25%; p = 0.038). On 9 April, the almost as inferior gray6
(15.67% vs 16.26%) was deleted. On 17 April, dark
(16.00% vs 16.94%) was deleted. On 30 April, I deleted gray2
(17.56% vs 18.07%). 11 May, blues
was gone (18.11% vs 18.53%), and on 31 May, I deleted gray3
(18.04% vs 18.24%).
Due to caching, the deletions didn’t necessarily drop data collection instantly to zero. Traffic was also heterogeneous: Hacker News traffic is much less likely to spend much time on page than the usual traffic.
The conversion data, with new vs returning visitor, segmented by period, and ordered by when a variant was deleted:
Variant |
Old |
Total: n (%) |
10–31 March |
1–6 April |
7–9 April |
10–17 April |
18–30 April |
1–11 May |
12–31 May |
1–8 June |
---|---|---|---|---|---|---|---|---|---|---|
|
FALSE |
17648 (16.01%) |
1189 (19.26%) 3 |
607 (13.97%) |
460 (17.39%) |
1182 (16.58%) |
3444 (17.04%) |
2397 (14.39%) |
3997 (17.39%) |
2563 (16.35%) |
|
TRUE |
8009 (23.65%) |
578 (24.91%) 1 |
236 (22.09%) |
226 (20.35%) |
570 (23.86%) |
1364 (27.05%) |
1108 (23.83%) |
2142 (22.46%) |
1363 (23.84%) |
|
FALSE |
17579 (16.28%) |
1177 (19.71%) 3 |
471 (14.06%) |
475 (13.47%) |
1200 (17.33%) |
3567 (17.49%) |
2365 (13.57%) |
3896 (18.17%) |
2605 (17.24%) |
|
TRUE |
7694 (23.85%) |
515 (28.35%) 1 |
183 (23.58%) |
262 (21.37%) |
518 (21.43%) |
1412 (26.56%) |
1090 (24.86%) |
2032 (22.69%) |
1197 (23.56%) |
|
FALSE |
14871 (15.81%) |
1192 (18.29%) 3 |
527 (14.15%) |
446 (15.47%) |
1160 (15.43%) |
3481 (17.98%) |
2478 (14.65%) |
3776 (16.26%) |
3 (33.33%) |
|
TRUE |
6631 (23.06%) |
600 (24.83%) 1 |
264 (21.52%) |
266 (18.05%) |
638 (21.79%) |
1447 (25.22%) |
1053 (24.60%) |
1912 (23.17%) |
51 (5.88%) |
|
FALSE |
10844 (15.34%) |
1157 (18.93%) 3 |
470 (14.35%) |
449 (16.04%) |
1214 (15.57%) |
3346 (17.54%) |
2362 (13.46%) |
3 (0.00%) |
|
|
TRUE |
4544 (23.04%) |
618 (27.18%) 1 |
256 (23.81%) |
296 (20.27%) |
584 (22.09%) |
1308 (24.46%) |
1052 (22.15%) |
48 (12.50%) |
|
|
FALSE |
8646 (15.51%) |
1220 (20.33%) 3 |
649 (13.81%) |
416 (15.14%) |
1144 (15.03%) |
3433 (17.54%) |
4 (0.00%) |
||
|
TRUE |
3366 (22.82%) |
585 (22.74%) 1 |
271 (21.79%) |
230 (16.52%) |
514 (21.60%) |
1298 (25.42%) |
44 (27.27%) |
6 (0.00%) |
3 (0.00%) |
|
FALSE |
5240 (14.05%) |
1224 (20.59%) 3 |
644 (13.83%) |
420 (13.81%) |
1175 (14.81%) |
1 (0.00%) |
|||
|
TRUE |
2161 (20.59%) |
618 (21.52%) 1 |
242 (20.85%) |
276 (21.74%) |
574 (20.56%) |
64 (10.94%) |
1 (0.00%) |
2 (0.00%) |
2 (50.00%) |
|
FALSE |
4022 (13.30%) |
1153 (19.51%) 3 |
610 (12.88%) |
409 (17.11%) |
1 (0.00%) |
2 (0.00%) |
3 (0.00%) |
||
|
TRUE |
1727 (20.61%) |
654 (23.70%) 1 |
358 (22.02%) |
259 (18.92%) |
95 (7.37%) |
11 (9.09%) |
1 (0.00%) |
||
|
FALSE |
3245 (12.20%) |
1175 (16.68%) 3 |
242 (12.21%) |
3 (0.00%) |
|||||
|
TRUE |
1180 (21.53%) |
559 (25.94%) 1 |
130 (21.77%) |
34 (17.65%) |
16 (12.50%) |
||||
|
FALSE |
1176 (18.54%) |
1174 (18.57%) 1 |
174 (18.57%) |
2 (0.00%) |
|||||
|
TRUE |
673 (19.91%) |
650 (20.31%) 6 |
69 (20.03%) |
1 (0.00%) |
1 (0.00%) |
2 (0.00%) |
|||
137438 (18.27%) |
Graphed:
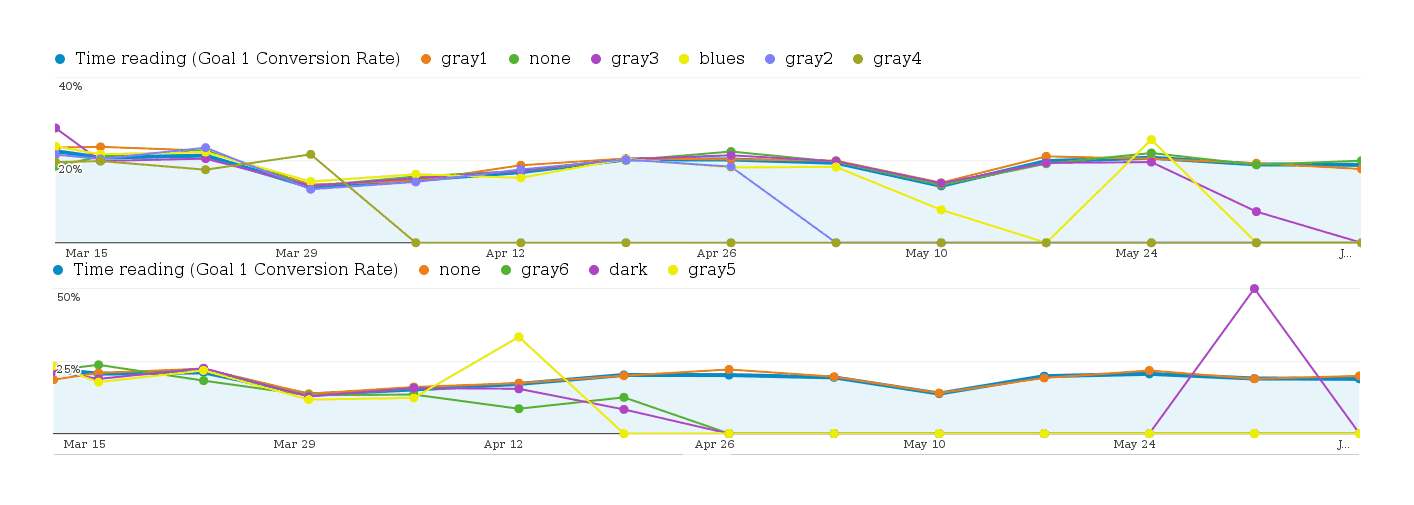
I also received a number of complaints while running the BLR test (principally due to the dark
and blues
variants, but also apparently triggered by some of the less popular gray variants; the number of complaints dropped off considerably by halfway through):
-
2 in emails
-
2 on IRC unsolicited; when I later asked, there were 2 complaints of slowness loading pages & after reflowing
-
2 on Reddit
-
3 mentions in Gwern.net comments
-
4 through my anonymous feedback form
-
6 complaints on Hacker News
-
total: 19
Analysis
The BLR people say that there may be cross-browser differences, so I thought about throwing in browser as a covariate too (an unordered factor of Chrome & Firefox, and maybe I’ll bin everything else as an ‘other’ browser); it seems I may have to use the GA API to extract conversion rates split by variant, visitor status, and browser. This turned out to be enough work that I decided to not bother.
As usual, a logistic regression on the various BLR themes with new vs returning visitors (Old
) as a covariate. Because of the heterogeneity in traffic (and because I bothered breaking out the data by time period this time for the table), I also include each block as a factor. Finally, because I expected the 6 gray variants to perform similarly, I try out a multilevel model nesting the grays together.
The results are not impressive: only 2 gray variants out of the 8 variants have a positive estimate, and neither is statistically-significant; the best variant was gray1
(“#222222” & “#FBFBFB”), at an estimated increase from 19.52% to 20.04% conversion rate. More surprising, the nesting turns out to not matter at all, and in fact the worst variant was gray. (The best-fitting multilevel model ignore the variants entirely, although it did not fit better than the regular logistic model incorporating all of the time periods, Old
, and variants.)
# Pivot table view on custom variable:
# ("Secondary dimension: User Type"; "Pivot by: Custom Variable (Value 01); Pivot metrics: Sessions | Time reading (Goal 1 Conversion Rate)")
# then hand-edited to add Color and Date variables
rates <- read.csv("https://gwern.net/doc/traffic/2014-06-08-abtesting-blr.csv")
rates$Successes <- rates$N * rates$Rate
rates$Successes <- round(rates$Successes,0)
rates$Failures <-rates$N - rates$Successes
# specify the control group is 'none'
rates$Variant <- relevel(rates$Variant, ref="none")
rates$Color <- relevel(rates$Color, ref="none")
# normal:
g0 <- glm(cbind(Successes,Failures) ~ Old + Variant + Date, data=rates, family=binomial); summary(g0)
# ...Coefficients:
# Estimate Std. Error z value Pr(>|z|)
# (Intercept) -1.633959 0.027712 -58.96 < 2e-16
# OldTRUE 0.465491 0.014559 31.97 < 2e-16
# Date10-17 April -0.021047 0.037563 -0.56 0.5753
# Date10-31 March 0.150498 0.035017 4.30 1.7e-05
# Date1-11 May -0.107965 0.035133 -3.07 0.0021
# Date12-31 May 0.009534 0.032448 0.29 0.7689
# Date1-6 April -0.138053 0.031809 -4.34 1.4e-05
# Date18-30 April 0.095898 0.031817 3.01 0.0026
# Date7-9 April -0.129704 0.047314 -2.74 0.0061
#
# Variantgray5 -0.114487 0.040429 -2.83 0.0046
# Variantdark -0.060299 0.033912 -1.78 0.0754
# Variantgray2 -0.027338 0.028518 -0.96 0.3378
# Variantblues -0.012120 0.026330 -0.46 0.6453
# Variantgray3 -0.005484 0.023441 -0.23 0.8150
# Variantgray4 -0.003556 0.047273 -0.08 0.9400
# Variantgray6 0.000536 0.036308 0.01 0.9882
# Variantgray1 0.026765 0.021757 1.23 0.2186
library(lme4)
g1 <- glmer(cbind(Successes,Failures) ~ Old + (1|Color/Variant) + (1|Date), data=rates, family=binomial)
g2 <- glmer(cbind(Successes,Failures) ~ Old + (1|Color) + (1|Date), data=rates, family=binomial)
g3 <- glmer(cbind(Successes,Failures) ~ Old + (1|Date), data=rates, family=binomial)
g4 <- glmer(cbind(Successes,Failures) ~ Old + (1|Variant), data=rates, family=binomial)
g5 <- glmer(cbind(Successes,Failures) ~ Old + (1|Color), data=rates, family=binomial)
AIC(g0, g1, g2, g3, g4, g5)
# df AIC
# g0 17 1035
# g1 5 1059
# g2 4 1058
# g3 13 1041
# g4 3 1252
# g5 3 1264
Conclusion
An unlikely +0.5% to reading rates isn’t enough for me to want to add a dependency another JS library, so I will be removing BLR. I’m not surprised by this result, since most tests don’t show an improvement, BLR coloring test is pretty unusual for a website, and users wouldn’t have any understanding of what it is or ability to opt out of it; using BLR by default doesn’t work, but the browser extension might be useful since the user expects the coloring & can choose their preferred color scheme.
I was surprised that the gray variants could perform so wildly different, from slightly better than the control to horribly worse, considering that they didn’t strike me as looking that different when I was previewing them locally. I also didn’t expect blues
to last as long as it did, and thought I would be deleting it as soon as dark
. This makes me wonder: are there color themes only subtly different from the ones I tried which might work unpredictably well? Since BLR by default offers only a few themes, I think BLR should try out as many color themes as possible to locate good ones they’ve missed.
Some limitations to this experiment:
-
no way for users to disable BLR or change color themes
-
did not include web browser type as a covariate, which might have shown that particular combinations of browser & theme substantially outperformed the control (then BLR could have improved their code for the bad browsers or a browser check done before highlighting any text)
-
did not use formal adaptive trial methodology, so the p-values have no particular interpretation
Floating Footnotes
One of the site features I like the most is how the endnotes pop-out/float when the mouse hovers over the link, so the reader doesn’t have to jump to the endnotes and back, jarring their concentration and breaking their train of thought. I got the JS from Luka Mathis back in 2010. But sometimes the mouse hovers by accident, and with big footnotes, the popped-up footnote can cover the screen and be unreadable. I’ve wondered if it’s as cool as I think it is, or whether it might be damaging. So now that I’ve hacked up an ABalytics clone which can handle JS in order to run the BLR experiment, I might as well run an A/B test to verify that the floating footnotes are not badly damaging conversions. (I’m not demanding the floating footnotes increase conversions by 1% or anything, just that the floating isn’t coming at too steep a price.)
Implementation
diff --git a/static/js/footnotes.js b/static/js/footnotes.js
index 69088fa..e08d63c 100644
--- a/static/js/footnotes.js
+++ b/static/js/footnotes.js
@@ -1,7 +1,3 @@
-$(document).ready(function() {
- Footnotes.setup();
-});
-
diff --git a/static/template/default.html b/static/template/default.html
index 4395130..8c97954 100644
--- a/static/template/default.html
+++ b/static/template/default.html
@@ -133,6 +133,9 @@
<script type="text/javascript" src="//ajax.googleapis.com/ajax/libs/jquery/1.7.1/jquery.min.js"></script>
+ <script type="text/javascript" src="/static/js/footnotes.js"></script>
+
<script id="googleAnalytics" type="text/javascript">
var _gaq = _gaq || [];
@@ -151,14 +154,23 @@
if (typeof(start_slot) == 'undefined') start_slot = 1;
- var experiment = "blr3";
- var variant_names = ["none", "gray1"];
+ var experiment = "floating_footnotes";
+ var variant_names = ["none", "float"];
var variant_id = this.readCookie("ABalytics_"+experiment);
if (!variant_id || !variant_names[variant_id]) {
var variant_id = Math.floor(Math.random()*variant_names.length);
document.cookie = "ABalytics_"+experiment+"="+variant_id+"; path=/";
}
+ // enable the floating footnotes
+ function footnotefy (VARIANT) {
+ if (VARIANT != "none") {
+ $$(document).ready(function() {
+ Footnotes.setup();
+ });
+ }
+ }
+ footnotefy(variant_names[variant_id]);
_gaq.push(['_setCustomVar',
start_slot,
experiment, // The name of the custom variable = name of the experiment
...)]
@@ -196,9 +208,6 @@
<script type="text/javascript" src="http://cdn.mathjax.org/mathjax/latest/MathJax.js?config=TeX-AMS-MML_HTMLorMML"></script>
- <script type="text/javascript" src="/static/js/footnotes.js"></script>
-
<script type="text/javascript" src="/static/js/tablesorter.js"></script>
<script type="text/javascript" id="tablesorter">
Data
2014-06-08–2014-07-12:
Variant |
Old |
n |
Conversion |
---|---|---|---|
none |
FALSE |
10342 |
17.00% |
float |
FALSE |
10039 |
17.42% |
none |
TRUE |
4767 |
22.24% |
float |
TRUE |
4876 |
22.40% |
none |
15109 |
18.65% |
|
float |
14915 |
19.05% |
|
30024 |
18.85% |
Analysis
rates <- read.csv(stdin(),header=TRUE)
Footnote,Old,N,Rate
none,FALSE,10342,0.1700
float,FALSE,10039,0.1742
none,TRUE,4767,0.2224
float,TRUE,4876,0.2240
rates$Successes <- rates$N * rates$Rate
rates$Successes <- round(rates$Successes,0)
rates$Failures <-rates$N - rates$Successes
rates$Footnote <- relevel(rates$Footnote, ref="none")
g <- glm(cbind(Successes,Failures) ~ Footnote + Old, data=rates, family="binomial"); summary(g)
# ...Coefficients:
# Estimate Std. Error z value Pr(>|z|)
# (Intercept) -1.5820 0.0237 -66.87 <2e-16
# Footnotefloat 0.0222 0.0296 0.75 0.45
# OldTRUE 0.3234 0.0307 10.53 <2e-16
confint(g)
# 2.5 % 97.5 %
# (Intercept) -1.62856 -1.53582
# Footnotefloat -0.03574 0.08018
# OldTRUE 0.26316 0.38352
As I had hoped, floating footnotes seems to do no harm, and the point-estimate is positive. The 95% CI, while not excluding zero, does exclude values worse than -0.035, which satisfies me: if floating footnotes are doing any harm, it’s a small harm.
Indented Paragraphs
An anonymous feedback suggested a site design tweak:
Could you format your pages so that the texts are all aligned at the left? It looks unprofessional when the lines of text break at different areas. Could you make the site like a LaTeX article? The formatting is the only thing preventing you from looking really professional.
I wasn’t sure what he meant, since the text is left-aligned, and I can’t ask for clarification (anonymous means anonymous).
Looking at a random page, my best guess is that he’s bothered by the indentation at the start of successive paragraphs: in a sequence of paragraphs, the first paragraph is not indented (because it can’t be visually confused) but the successive paragraphs are indented by 1.5em in order to make reading easier. The CSS is:
p { margin-top: -0.2em;
margin-bottom: 0 }
p + p {
text-indent: 1.5em;
margin-top: 0 }
I liked this, but I suppose for lots of small paragraphs, it lends a ragged appearance to the page. So might as well test a few variants of text-indent
to see what works best: 0em, 0.1, 0.5, 1.0, 1.5, and 2.0.
In retrospect years later, after learning more about typography and revamping Gwern.net CSS a number of times, I think Anonymous was actually talking about text justification: HTML/Gwern.net is by default “flush left, ragged right”, with large whitespace gaps left where words of different lengths get moved to the next line but not broken/hyphenated or stretched to fill the line. Some people do not like text justification, describing ragged right as easier to read, but most typographers endorse it, it was historically the norm for professionally-set print, still carries connotations of class, and I think the appearance fits in with my overall site esthetic. I eventually enabled text justification on Gwern.net in February 2019 (although I was irritated by the discovery that the standard CSS method of doing so does not work in the Chrome browser due to a long-standing failure to implement hyphenation support).
Implementation
Since we’re back to testing CSS, we can use the old ABalytics approach without having to do JS coding:
--- a/static/template/default.html
+++ b/static/template/default.html
@@ -19,6 +19,9 @@
</head>
<body>
+ <div class="indent_class1"></div>
+
<div id="main">
<div id="sidebar">
<div id="logo"><img alt="Logo: a Gothic/Fraktur blackletter capital G/𝕲" height="36" src="/image/logo/logo.png" width="32" /></div>
@@ -136,10 +139,48 @@
<script type="text/javascript" src="/static/js/footnotes.js"></script>
+ <script type="text/javascript" src="/static/js/abalytics.js"></script>
+ <script type="text/javascript">
+ window.onload = function() {
+ ABalytics.applyHtml();
+ };
+ </script>
+
<script id="googleAnalytics" type="text/javascript">
var _gaq = _gaq || [];
_gaq.push(['_setAccount', 'UA-18912926-1']);
+
+ ABalytics.init({
+ indent: [
+ {
+ name: "none",
+ "indent_class1": "<style>p + p { text-indent: 0.0em; margin-top: 0 }</style>"
+ },
+ {
+ name: "indent0.1",
+ "indent_class1": "<style>p + p { text-indent: 0.1em; margin-top: 0 }</style>"
+ },
+ {
+ name: "indent0.5",
+ "indent_class1": "<style>p + p { text-indent: 0.5em; margin-top: 0 }</style>"
+ },
+ {
+ name: "indent1.0",
+ "indent_class1": "<style>p + p { text-indent: 1.0em; margin-top: 0 }</style>"
+ },
+ {
+ name: "indent1.5",
+ "indent_class1": "<style>p + p { text-indent: 1.5em; margin-top: 0 }</style>"
+ },
+ {
+ name: "indent2.0",
+ "indent_class1": "<style>p + p { text-indent: 2.0em; margin-top: 0 }</style>"
+ }
+ ],
+ }, _gaq);
+
_gaq.push(['_trackPageview']);
(function() { // })
Data
2014-07-27, since the 95% CIs for the best and worst indent variants no longer overlapped, I deleted the worst variant (0.1
). 2014-08-23, the 2.0em and 0.0em variants no longer overlapped, and I deleted the latter.
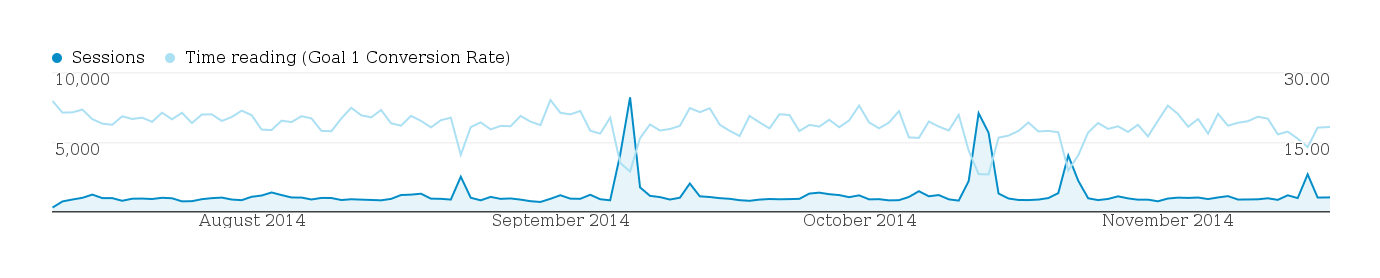
The conversion data, with new vs returning visitor, segmented by period, and ordered by when a variant was deleted:
Variant |
Old |
Total: n (%) |
12-27 July |
28 July-23 August |
24 August-19 November |
---|---|---|---|---|---|
|
FALSE |
1552 (18.11%) |
1551 (18.12%) |
1552 (18.11%) |
|
|
TRUE |
707 (21.64%) |
673 (21.69%) |
706 (21.67%) |
6 (0.00%) |
|
FALSE |
5419 (16.70%) |
1621 (17.27%) |
5419 (16.70%) |
3179 (16.55%) |
|
TRUE |
2742 (23.23%) |
749 (27.77%) |
2684 (23.62%) |
1637 (21.01%) |
|
FALSE |
26357 (15.09%) |
1562 (18.89%) |
5560 (17.86%) |
24147 (14.74%) |
|
TRUE |
10965 (21.35%) |
728 (23.63%) |
2430 (23.13%) |
9939 (21.06%) |
|
FALSE |
25987 (14.86%) |
1663 (19.42%) |
5615 (17.68%) |
23689 (14.39%) |
|
TRUE |
11288 (21.14%) |
817 (25.46%) |
2498 (24.38%) |
10159 (20.74%) |
|
FALSE |
26045 (14.54%) |
1619 (16.80%) |
5496 (16.67%) |
23830 (14.26%) |
|
TRUE |
11255 (21.60%) |
694 (26.95%) |
2647 (24.25%) |
10250 (21.00%) |
|
FALSE |
26198 (14.96%) |
1659 (18.75%) |
5624 (18.31%) |
23900 (14.59%) |
|
TRUE |
11125 (21.17%) |
781 (25.99%) |
2596 (24.27%) |
10010 (20.74%) |
159634 (16.93%) |
14117 (20.44%) |
42827 (19.49%) |
140746 (16.45%) |
Analysis
A simple analysis of the totals would indicate that 0.1em is the best setting - which is odd since it was the worst-performing and first variant to be deleted, so how could it be the best? The graph of traffic suggests that, like before, the final totals are confounded by time-varying changes in conversion rates plus dropping variants; that is, 0.1em probably only looks good because after it was dropped, a bunch of Hacker News traffic hit and happened to convert at lower rates, making the surviving variants look bad. One might hope that all of that effect would be captured by the Old covariate as HN traffic gets recorded as new visitors, but that would be too much to hope for. So instead, I add a dummy variable for each of the 3 separate time-periods which will absorb some of this heterogeneity and make clearer the effect of the indentation choices.
rates <- read.csv(stdin(),header=TRUE)
Indent,Old,Month,N,Rate
0.1,FALSE,July,1551,0.1812
0.1,TRUE,July,673,0.2169
0,FALSE,July,1621,0.1727
0,TRUE,July,749,0.2777
0.5,FALSE,July,1562,0.1889
0.5,TRUE,July,728,0.2363
1.0,FALSE,July,1663,0.1942
1.0,TRUE,July,817,0.2546
1.5,FALSE,July,1619,0.1680
1.5,TRUE,July,694,0.2695
2.0,FALSE,July,1659,0.1875
2.0,TRUE,July,781,0.2599
0.1,FALSE,August,1552,0.1811
0.1,TRUE,August,706,0.2167
0,FALSE,August,5419,0.1670
0,TRUE,August,2684,0.2362
0.5,FALSE,August,5560,0.1786
0.5,TRUE,August,2430,0.2313
1.0,FALSE,August,5615,0.1768
1.0,TRUE,August,2498,0.2438
1.5,FALSE,August,5496,0.1667
1.5,TRUE,August,2647,0.2425
2.0,FALSE,August,5624,0.1831
2.0,TRUE,August,2596,0.2427
0.1,FALSE,November,0,0.000
0.1,TRUE,November,6,0.000
0,FALSE,November,3179,0.1655
0,TRUE,November,1637,0.2101
0.5,FALSE,November,24147,0.1474
0.5,TRUE,November,9939,0.2106
1.0,FALSE,November,23689,0.1439
1.0,TRUE,November,10159,0.2074
1.5,FALSE,November,23830,0.1426
1.5,TRUE,November,10250,0.2100
2.0,FALSE,November,23900,0.1459
2.0,TRUE,November,10010,0.2074
rates$Successes <- rates$N * rates$Rate
rates$Successes <- round(rates$Successes,0)
rates$Failures <-rates$N - rates$Successes
g <- glm(cbind(Successes,Failures) ~ as.factor(Indent) + Old + Month, data=rates, family="binomial"); summary(g)
# ...Coefficients:
# Estimate Std. Error z value Pr(>|z|)
# (Intercept) -1.55640959 0.02238959 -69.51487 < 2.22e-16
# as.factor(Indent)0.1 -0.05726851 0.04400363 -1.30145 0.1931046
# as.factor(Indent)0.5 0.00249949 0.02503877 0.09982 0.9204833
# as.factor(Indent)1 -0.00877850 0.02502047 -0.35085 0.7256988
# as.factor(Indent)1.5 -0.02435198 0.02505726 -0.97185 0.3311235
# as.factor(Indent)2 0.00271475 0.02498665 0.10865 0.9134817
# OldTRUE 0.42448061 0.01238799 34.26549 < 2.22e-16
# MonthJuly 0.06606325 0.02459961 2.68554 0.0072413
# MonthNovember -0.20156678 0.01483356 -13.58857 < 2.22e-16
#
# (Dispersion parameter for binomial family taken to be 1)
#
# Null deviance: 1496.6865 on 34 degrees of freedom
# Residual deviance: 41.1407 on 26 degrees of freedom
# AIC: 331.8303
There’s definitely temporal heterogeneity, given the statistical-significance of the time-period dummies, so that is good to know. But the estimated effects for each indentation variant is derisorily small (despite having spent n = 159634), suggesting readers don’t care at all. Since I have no opinion on the matter, I suppose I’ll go with the highest point-estimate, 2em.
CSE
A CSE is a Google search query but one specialized in various ways - somewhat like offering an user a form field which redirects to a Google search query like QUERY site:gwern.net/doc/
, but more powerful since you can specify thousands of URLs to blacklist and whitelist and have limited patterns. I have two: one is specialized for searching for anime/manga news sites and makes writing Wikipedia articles much easier (since you can search for a particular anime title and the results will be mostly news and reviews which you can use in a WP article, rather than images, songs, memes, Amazon and commercial sites, blogs, etc); and the second is specialized to search Gwern.net, my Reddit, LessWrong, PredictionBook, Good Reads and some other sites, to make it easier to find something I may’ve written. The second I created to put in the sidebar and serve as a website search function. (I threw in the other sites because why not?)
Google provides HTML & JS for integrating a CSE somewhere, so creating & installing it was straightforward, and it went live 2013-05-24.
The problem is that the CSE search input takes up space in the sidebar, and is more JS to run on each page load and loads at least one other JS file as well. So on 2015-07-17, I took a look to evaluate whether it was worth keeping.
There had been 8974 searches since I installed it 785 days previously or ~11.4 searches per day; at least 119 were searches for “e”, which I assume were user mistakes where they didn’t intend to search and probably annoyed them. (The next most popular searches are “Graeber”/26, “chunking”/22, and “nootropics”/10, with CSE refusing to provide any further queries due to low volume. This suggests a long tail of search queries - but also that they’re not very important since it’s easy to find the DNB FAQ & my nootropics page, and it can hardly be useful if the top search is an error.)
To put these 8855 searches in perspective, in that same exact time period, there were 891,790 unique users with 2,010,829 page views. So only 0.44% of page-views involve an use of the CSE, or a ratio of 1:227 Is it net-beneficial to make 227 page-views incur the JS run & loading for the sake of 1 CSE search?
This might seem like a time to A/B test the presence/absence of the CSE div. (I can’t simply hide it using CSS like usual because it will still affect page loads.) Except consider the power issues: if that 1 CSE search converts, then to be profitable, it needs to damage the 227 other page-views conversion rate by <1/227. Or to put it the other way, the current conversion rate is ~17% of page-views and CSE search represents 0.44% of page-views, so if the CSE makes that one page-view 100% guaranteed to convert and otherwise converts normally, then over 1000 page-views, we have 0.17 × 995 + 1.0 × 5 = 174 vs 0.17 × 995 + 0.17 × 5 = 170, or 17.4% vs 17.0%.
power.prop.test(p1=0.174, p2=0.170, power=0.80, sig.level=0.05)
# Two-sample comparison of proportions power calculation
# n = 139724.5781
Even with the most optimistic possible assumptions (perfect conversion, no negative effect), it takes 279,449 page-views to get decent power. This is ridiculous from a cost-benefit perspective, and worse given that my priors are against it due to the extra JS & CSS it entails.
So I simply removed it. It was a bit of an experiment, and <8.9k searches does not seem worth it.
Deep Reinforcement Learning
A/B testing variants one at a time is fine as far as it goes, but it has several drawbacks that have become apparent:
-
fixed trials, compared to sequential or adaptive trial approaches, waste data/page-views. Looking back, it’s clear that many of these trials didn’t need to run so long.
-
they are costly to set up, both because of the details of a static site doing A/B tests but also because it requires me to define each change, code it up, collect, and analyze the results all by hand.
-
they are not amenable to testing complicated models or relationships, since factorial designs suffer combinatorial explosion.
-
they will test only the interventions the experimenter thinks of, which may be a tiny handful of possibilities out of a wide space of possible interventions (this is related to the cost: I won’t test anything that isn’t interesting, controversial, or potentially valuable, because it’s far too much of a hassle to implement/collect/analyze)
The topic of sequential trials leads naturally to multi-armed bandits (MAB), which can be seen as a generalization of regular experimenting which naturally reallocate samples across branches as the posterior probabilities change in a way which minimizes how many page-views go to bad variants. It’s hard to see how to implement MABs as a static site, so this would probably motivate a shift to a dynamic site, at least to the extent that the server will tweak the served static content based on the current MAB.
MABs work for the current use case of specifying a small number of variants (eg. <20) and finding the best one. Depending on implementation detail, they could also make it easy to run factorial trials checking for interactions among those variants, resolving another objection.
They’re still expensive to set up since one still has to come up with concrete variants to pit against each other, but if it’s now a dynamic server, it can at least handle the analysis automatically.
MABs themselves are a special case of reinforcement learning (RL), which is a family of approaches to exploring complicated systems to maximize a reward at (hopefully) minimum data cost. Optimizing a website fits naturally into a RL mold: all the possible CSS and HTML variants are a very complicated system, which we are trying to explore as cheaply as possible while maximizing the reward of visitors spending more time reading each page.
To solve the expressivity problem, one could try to equip the RLer with a lot of power over the CSS: parse it into an AST, so instead of specifying by hand ‘100%’ vs ‘105%’ in a CSS declaration like div#sidebar-news a { font-size: 105%; }
, the RLer sees a node in the AST like (font-size [Real ~ dnorm(100,20)])
and tries out numbers around 100% to see what yields higher conversion rates. Of course, this yields an enormous number of possibilities and my website traffic is not equally enormous. Informative priors on each node would help if one was using a Bayesian MAB to do the optimization, but a Bayesian model might be too weak to detect many effects. (You can’t easily put in interactions between every node of the AST, after all.)
In a challenging problem like this, deep neural networks come to mind, yielding a deep reinforcement learner (Q-learning) - such a system made a splash in 2013-2015 in learning to play dozens of Atari games (DQN). The deep network handles interpretation of the input, and the RLer handles policy and optimization.
So the loop would go something like this:
-
a web browser requests a page
-
the server asks the RL for CSS to include
-
the RL generates a best guess at optimal CSS, taking the CSS AST skeleton and returning the defaults, with some fields/parameters randomized for exploration purposes (possibly selected by Bayesian optimization to maximize information gain)
-
the CSS is transcluded into the HTML page, and sent to the web browser
-
JS analytics in the HTML page report back how long the user spent on that page and details like their country, web browser, etc, which predict time on page (explaining variance, making it easier to see effects)
-
this time-on-page constitutes the reward which is fed into the RL and updates
-
return to waiting for a request
Learning can be sped up by data augmentation or local training: the developer can browse pages locally and based on whether they look horrible or not, insert pseudo-data. (If one variant looks bad, it can be immediately heavily penalized by adding, say, 100 page-views of that variant with low rewards.) Once previews have stabilized on not-too-terrible-looking, it can be run on live users; the developer’s preferences may introduce some bias compared to the general Internet population, but the developer won’t be too different and this will kill off many of the worst variants. As well, historical information can be inserted as pseudo-data: if the current CSS file has 17% conversion over 1 million page views, one can simulate 1m page views to that CSS variant’s considerable credit.
Parsing CSS into an AST seems difficult, and it is still limited in that it will only ever tweak existing CSS fields.
How to offer more power and expressivity to the RLer without giving it so much freedom that it will hang itself with gibberish CSS before ever finding working CSS, never mind improvements?
A powerful AI tool which could generate CSS on its own are the recurrent neural networks: NNs which generate some output which gets fed back in until a long sequence has been emitted. (They usually also have some special support for storing ‘memories’ over multiple recursive applications, using LSTM.) RNNs are famous for mimicking text and other sequential material; in one demo, Karpathy’s “The Unreasonable Effectiveness of Recurrent Neural Networks”, he trained a RNN on a Wikipedia dump in XML format and a LaTeX math book (both replicating the syntax quite well) and more relevantly, 474MB of C source code & headers where the RNN does a credible job of emitting pseudo-C code which looks convincing and is even mostly syntactically-correct in balancing parentheses & brackets, which more familiar Markov-chain approaches would have trouble managing. (Of course, the pseudo-C doesn’t do anything but that RNN was never asked to make it do something, either.) In another RNN paper, the authors trained it on Python source code and it was able to ‘execute’ very simple Python programs and predict the output; this is perhaps not too surprising given the earlier “Neural Turing Machines” and solving the Traveling Salesman Problem (“Pointer Networks”). So RNNs are powerful and have already shown promise in learning how to write simple programs.
This suggests the use of an RNN inside an RLer for generating CSS files. Train the RNN on a few hundred megabytes of CSS files (there are millions online, no shortage there), which teaches the RNN about the full range of possible CSS expressions, then plug it into step 3 of the above website optimization algorithm and begin training it to emit useful CSS. For additional learning, the output can be judged using an oracle (a CSS validator like the W3C CSS Validation Service/w3c-markup-validator
package, or possibly CSSTidy), and the error or reward based on how many validation errors there are. The pretraining provides extremely strong priors about what CSS should look like so syntactically valid CSS will be mostly used without the constraint of operating on a rigid AST, the RL begins optimizing particular steps, and providing the original CSS with a high reward prevents it from straying too far from a known good design.
Can we go further? Perhaps. In the Atari RL paper, the NN was specifically a convolutional neural network (CNN), used almost universally in image classification tastes; the CNN was in charge of understanding the pixel output so it could be manipulated by the RL. The RNN would have considerable understanding of CSS on a textual level, but it wouldn’t be easily able to understand how one CSS declaration changes the appearance of the webpage. A CNN, on the other hand, can look at a page+CSS as rendered by a web browser, and ‘see’ what it looks like; possibly it could learn that ‘messy’ layouts are bad, that fonts shouldn’t be made ‘too big’, that blocks shouldn’t overlap, etc. The RNN generates CSS, the CSS is rendered in a web browser, the rendering is looked at by a CNN… and then what? I’m not sure how to make use of a generative approach here. Something to think about.
-
Reinforcement Learning: An Introduction, 1998/2017
-
“Reinforcement Learning with Recurrent Neural Networks”, 2008
-
DQN: “Human-level control through deep reinforcement learning”, source code, simple Pong version; parallelizing it
-
“Self-improving reactive agents based on reinforcement learning, planning and teaching”, 1992
-
“Reinforcement learning: A survey”, by Kaelbling, L.P., Littman, M.L., and Moore, A.W., in the Journal of Artificial Intelligence Research, 4:237–285, 1996.
-
“Learning and sequential decision making”, et al 1990
-
“Deep Reinforcement Learning”, 2015 lecture
-
Dynamic Programming, 1957
-
“Chapter 10; Sequence Modeling: Recurrent and Recursive Nets”, Deep Learning (Bengio et al draft)
Recurrent Q-learning:
-
1992 “Memory approaches to reinforcement learning in non-Markovian domains”
-
Meeden, 1993 “Emergent control and planning in an autonomous vehicle”
-
1991b “Reinforcement learning in Markovian and non-Markovian environments”
-
http://nikhilbuduma.com/2015/01/11/a-deep-dive-into-recurrent-neural-networks/
Training A Neural Net To Generate CSS
It would be nifty if I could set up a NN to generate and optimize the CSS on Gwern.net so I don’t have to learn CSS & devise tests myself; as a first step towards this, I wanted to see how well a recurrent neural network (RNN) could generate CSS after being trained on CSS. (If it can’t do a good job mimicking the ‘average’ syntax/appearance of CSS based on a large CSS corpus, then it’s unlikely it can learn more useful things like generating usable CSS given a particular HTML file, or the ultimate goal - learn to generate optimal CSS given HTML files and user reactions.)
char-rnn
Fortunately, Karpathy has already written an easy-to-use tool char-rnn
which has already been shown to work well on XML/LaTeX/C. (I was particularly amused by the LaTeX/math textbook, which yielded a compiling and even good-looking document after Karpathy fixed some errors in it; if the RNN had been trained against compile errors/warnings as well, perhaps it would not have needed any fixing at all…?)
char-rnn
relies on the Torch NN framework & NVIDIA’s CUDA GPU framework (Ubuntu installation guide/download).
Torch is fairly easy to install (cheat sheet):
cd ~/src/
curl -s https://raw.githubusercontent.com/torch/ezinstall/master/install-deps | bash
git clone https://github.com/torch/distro.git ./torch --recursive
cd ./torch; ./install.sh
export PATH=$HOME/src/torch/install/bin:$PATH
## fire up the REPL to check:
th
Then char-rnn
is likewise easy to get running and try out a simple example:
luarocks install nngraph
luarocks install optim
# luarocks install cutorch && luarocks install cunn ## 'cutorch' & 'cunn' need working CUDA
git clone 'https://github.com/karpathy/char-rnn.git'
cd ./char-rnn/
th train.lua -data_dir data/tinyshakespeare/ -gpuid 0 -rnn_size 512 -num_layers 2 -dropout 0.5
# package cunn not found!
# package cutorch not found!
# If cutorch and cunn are installed, your CUDA toolkit may be improperly configured.
# Check your CUDA toolkit installation, rebuild cutorch and cunn, and try again.
# Falling back on CPU mode
# loading data files...
# cutting off end of data so that the batches/sequences divide evenly
# reshaping tensor...
# data load done. Number of data batches in train: 423, val: 23, test: 0
# vocab size: 65
# creating an lstm with 2 layers
# number of parameters in the model: 3320385
# cloning rnn
# cloning criterion
# 1/21150 (epoch 0.002), train_loss = 4.19087871, grad/param norm = 2.1744e-01, time/batch = 4.98s
# 2/21150 (epoch 0.005), train_loss = 4.99026574, grad/param norm = 1.8453e+00, time/batch = 3.13s
# 3/21150 (epoch 0.007), train_loss = 4.29807770, grad/param norm = 5.6664e-01, time/batch = 4.30s
# 4/21150 (epoch 0.009), train_loss = 3.78911860, grad/param norm = 3.1319e-01, time/batch = 3.87s
# ...
Unfortunately, even on my i7 CPU, training is quite slow: ~3s a batch on the Tiny Shakespeare example. The important parameter is train_loss
here1; after some experimenting, I found that >3=output is total garbage, 1-2=lousy, and with <1=good, with <0.8=very good.
With Tiny Shakespeare, the loss drops quickly at first, getting <4 within seconds and into the 2s within 20 minutes, but then the 1s take a long time to surpass, and <1 even longer (hours of waiting).
GPU vs CPU
This is a toy dataset and suggests that for a real dataset I’d be waiting weeks or months. GPU acceleration is critical. I spent several days trying to get Nvidia’s CUDA to work, even signing up as a developer & using the unreleased version 7.5 preview of CUDA, but it seems that when they say Ubuntu 14.04 and not 15.04 (the latter is what I have installed), they are quite serious: everything I tried yielded bloodcurdling ATA hard drive errors (!) upon boot followed by a hard freeze the instant X began to run.2 This made me unhappy since my old laptop began dying in late July 2015 and I had purchased my Acer Aspire V17 Nitro Black Edition VN7-791G-792A laptop with the express goal of using its NVIDIA GeForce GTX 960M for deep learning. But at the moment I am out of ideas for how to get CUDA working aside from either reinstalling to downgrade to Ubuntu 14.04 or simply waiting for version 8 of CUDA which will hopefully support the latest Ubuntu. (Debian is not an option because on Debian Stretch, I could not even get the GPU driver to work, much less CUDA.)31
Frustrated, I finally gave up and went the easy way: Torch provides an Amazon OS image preconfigured with Torch, CUDA, and other relevant libraries for deep learning.
EC2
The Torch AMI can be immediately launched if you have an AWS account. (I assume you have signed up, have a valid credit card, IP permission accesses set to allow you to connect to your VM at all, and a SSH public key set up so you can log in.) The two GPU instances seem to have the same number and kind of GPUs (1 Nvidia4) and differ mostly in RAM & CPUs, neither of which are the bottleneck here, so I picked the smaller/cheaper “g2.2xlarge” type. (“Cheaper” here is relative; “g2.2xlarge” still costs $0.84$0.652015/hr and when I looked at spot that day, ~$0.27$0.212015.)
Once started, you can SSH using your registered public key like any other EC2 instance. The default username for this image is “ubuntu”, so:
ssh -i /home/gwern/.ssh/EST.pem ubuntu@ec2-54-164-237-156.compute-1.amazonaws.com
Once in, we set up the $PATH
to find the Torch installation like before (I’m not sure why Torch’s image doesn’t already have this done) and grab a copy of char-rnn
to run Tiny Shakespeare:
export PATH=$HOME/torch/install/bin:$PATH
git clone 'https://github.com/karpathy/char-rnn'
# etc
Per-batch, this yields a 20x speedup on Tiny Shakespeare compared to my laptop’s CPU, running each batch in ~0.2s.
Now we can begin working on what we care about.
CSS
First, to generate a decent sized CSS corpus; between all the HTML documentation installed by Ubuntu and my own WWW crawls, I have something like 1GB of CSS hanging around my drive. Let’s grab 20MB of it (enough to not take forever to train on, but not so little as to be trivial):
cd ~/src/char-rnn/
mkdir ./data/css/
find / -type f -name "*.css" -exec cat {} \; | head --bytes=20MB >> ./data/css/input.txt
## https://www.dropbox.com/s/mvqo8vg5gr9wp21/rnn-css-20mb.txt.xz
wc --chars ./data/css/input.txt
# 19,999,924 ./data/input.txt
scp -i ~/.ssh/EST.pem -C data/css/input.txt ubuntu@ec2-54-164-237-156.compute-1.amazonaws.com:/home/ubuntu/char-rnn/data/css/
With 19.999M characters, our RNN can afford only <20M parameters; how big can I go with -rnn_size
and -num_layers
? (Which as they sound like, specify the size of each layer and how many layers.) The full set of char-rnn
training options:
# -data_dir data directory. Should contain the file input.txt with input data [data/tinyshakespeare]
# -rnn_size size of LSTM internal state [128]
# -num_layers number of layers in the LSTM [2]
# -model LSTM, GRU or RNN [LSTM]
# -learning_rate learning rate [0.002]
# -learning_rate_decay learning rate decay [0.97]
# -learning_rate_decay_after in number of epochs, when to start decaying the learning rate [10]
# -decay_rate decay rate for RMSprop [0.95]
# -dropout dropout for regularization, used after each RNN hidden layer. 0 = no dropout [0]
# -seq_length number of timesteps to unroll for [50]
# -batch_size number of sequences to train on in parallel [50]
# -max_epochs number of full passes through the training data [50]
# -grad_clip clip gradients at this value [5]
# -train_frac fraction of data that goes into train set [0.95]
# -val_frac fraction of data that goes into validation set [0.05]
# -init_from initialize network parameters from checkpoint at this path []
# -seed torch manual random number generator seed [123]
# -print_every how many steps/minibatches between printing out the loss [1]
# -eval_val_every every how many iterations should we evaluate on validation data? [1000]
# -checkpoint_dir output directory where checkpoints get written [cv]
# -savefile filename to autosave the checkpoint to. Will be inside checkpoint_dir/ [lstm]
# -gpuid which GPU to use. -1 = use CPU [0]
# -opencl use OpenCL (instead of CUDA) [0]
Large RNN
Some playing around suggests that the upper limit is 950 neurons and 3 layers, yielding a total of 18,652,422 parameters. (I originally went with 4 layers, but with that many layers, RNNs seem to train very slowly.) Some other settings to give an idea of how parameter count increases:
-
512/4: 8,012,032
-
950/3: 18,652,422
-
1000/3: 20,634,122
-
1024/3: 21,620,858
-
1024/4: 30,703,872
-
1024/5: 39,100,672
-
1024/6: 47,497,472
-
1800/4: 93,081,856
-
2048/4: 120,127,744
-
2048/5: 153,698,560
-
2048/6: 187,269,376
If we really wanted to stress the EC2 image’s hardware, we could go as large as this:
th train.lua -data_dir data/css/ -rnn_size 1306 -num_layers 4 -dropout 0.5 -eval_val_every 1
This turns out to not be a good idea since it will take forever to train - eg. after ~70m of training, still at train-loss of 3.7! I suspect some of the hyperparameters may be important - the level of dropout doesn’t seem to matter much but more than 3 layers seems to be unnecessary and slow if there are a lot of neurons to store state (perhaps because RNNs are said to ‘unroll’ computations over each character/time-step instead of being forced to do all their computation in a single deep network with >4 layers?) - but with the EC2 clock ticking and my own impatience, there’s no time to try a few dozen random sets of hyperparameters to see which achieves best validation scores.
Undeterred, I decided to upload all the CSS (using the sort-key
trick to reduce the archive size):
find / -type f -name "*.css" | rev | sort | rev | tar c --to-stdout --no-recursion --files-from - | xz -9 --stdout > ~/src/char-rnn/data/css/all.tar.xz
cd ~/src/char-rnn/ && scp -C data/css/all.tar.xz ubuntu@ec2-54-164-237-156.compute-1.amazonaws.com:/home/ubuntu/char-rnn/data/css/
unxz all.tar.xz
## non-ASCII input seems to cause problems, so delete anything not ASCII:
## https://disqus.com/home/discussion/karpathyblog/the_unreasonable_effectiveness_of_recurrent_neural_networks_66/#comment-2042588381
## https://github.com/karpathy/char-rnn/issues/51
tar xfJ data/css/all.tar.xz --to-stdout | iconv -c -tascii > data/css/input.txt
wc --char all.css
# 1,126,949,128 all.css
Unsurprisingly, this did not solve the problem, and with 1GB of data, even 1 pass over the data (1 epoch) would take weeks, likely. Additional problems included -val_frac
’s default 50 and -eval_val_every
’s default 1000: 0.05 of 1GB is 50MB, which means every time char-rnn
checked on the validation set, it took ages; and since it only wrote a checkpoint out every 1000 iterations, hours would pass in between checkpoints. 1MB or 0.001 is a more feasible validation data size; and checking every 100 iterations strikes a reasonable balance between being able to run the latest & greatest and spending as much GPU time on training as possible.
Small RNN
So I backed off to the 20MB sample and a smaller 3-layer RNN, training it overnight, and was startled to see what happened:
th train.lua -print_every 5 -data_dir data/css/ -savefile css -eval_val_every 10000 -val_frac 0.001 -rnn_size 1700 -num_layers 3 -dropout 0.8
# ...
# 20000/21408250 (epoch 0.047), train_loss = 0.84076253, grad/param norm = 3.4693e-03, time/batch = 3.59s
# loss is exploding, aborting.
Specifically, the loss on the validation set had exploded to 333.2351 (!). When I looked at samples from the check-pointed copy, it performed both well and poorly. th sample.lua cv/lm_css_epoch0.05_333.2351.t7
yielded:
...
.nest-comments .mb_comments p,
.pomenefister .views-content .add-post,
.pleuse-product-nav .dropdown-pane .date .intro,
.product-nav .shortlist.tooltip_pane .bread-slide,
.module-category .vote-content td {
font-size: 9px;
text-align: center;
}
.teotspaces-left h2,
.footer .social-icons .mepubititab-left ul {
margin: 0;
}
.mlissbox-box .post-content {
margin-top: 10px;
}
.secondary .tooltip-arrow .scegee-category h6,
.no-list .comment-footer .module-title,
.featured-left-column .lightbox-description h0,
.popup-tomimate .social-rover .comment-arrow {
list-style: none;
}
.tooltip-post .secondary .module-menu-houder .thumbnail {
float: left;
}
.module-content .lightbox-list {
display: inline-block;
float: none;
width: 50%;
position: relative;
background-position: 0 1px;
width: 30px;
margin-top: 5px;
text-align: center;
margin-right: 10px;
}
.logo-events .show-luset .box-content li {
width: 30px;
-webkit-box-shadow: inset 0 2px 4px rgba(0, 0, 0, 0.3);
}
.search-results .section-featured .content-top-section-left {
margin-top: -6px;
}
.secondary .module-mones-grey .down-mod-page {
margin-bottom: 20px;
}
.comment-top-content .meviat-alignright {
width: 350px;
}
.module-contributor h2.comment-hold-homicate.sptbed_postnames{
display: inline-block;
padding-top: -24px;
}
.secondary .module-content .logo div {
float: left;
}
.medium-block-grid-1 > li:hover {
border-bottom: none #5c466d;
}
.row-image-container .medium-topic-browser {
clear: right;
}
.rooc-box-sprite .modal-links .list-group li {
display: inline-block;
float: left;
padding-top: 8px;
}
.vead-video-list {
display: block;
margin-right: 13px;
}
#no-touch .main-tabs-new-content .widget-top-content {
color: #58128c;
display: block;
padding: 8px 0;
border-color: transparent;
border-bottom: 1px solid #fff;
padding: 5px 12px;
text-align: center;
}
Aside from the Unicode junk at the beginning, the output actually looks tremendously like CSS! The brackets are matched, the selectors look like selectors, and the fields are properly typed (pixels go into pixel fields, colors go into color fields, etc). If I validate the non-junk CSS part, the validator remarkably yields only 1 error, at line 52/.module-contributor h2.comment-hold-homicate.sptbed_postnames
where it notes that “Value Error : padding-top -24px negative values are not allowed : -24px”. Considering it didn’t even finish 1 epoch, the mimicking is almost uncanny: it nails the various aspects like RGB color notation (both hex & rgba()
), matching brackets, plausible-sounding identifiers (eg. .scegee-category
), etc. If I were shown this without any corresponding HTML, I would not easily be able to tell it’s all gibberish.
Chastened by the exploding-error problem and the mostly waste of ~26 hours of processing (7:30PM–9:30PM / $20.23$15.62015), I tried a smaller yet RNN (500/2), run from 5PM–11AM (so total bill for all instances, including various playing around, restarting, generating samples, downloading to laptop etc: $33.17$25.582015).
Data URI Problem
One flaw in the RNN I stumbled across but was unable to reproduce was that it seemed to have a problem with data URIs. A data URI is a special kind of URL which is its own content, letting one write small files inline and avoiding needing a separate file; for example, this following CSS fragment would yield a PNG image without the user’s browser making additional network requests or the developer needing to create a tiny file just for an icon or something:
.class {
content: url('data:image/png;base64,iVBORw0KGgoAA \
AANSUhEUgAAABAAAAAQAQMAAAAlPW0iAAAABlBMVEUAAAD///+l2Z/dAAAAM0l \
EQVR4nGP4/5/h/1+G/58ZDrAz3D/McH8yw83NDDeNGe4Ug9C9zwz3gVLMDA/A6 \
P9/AFGGFyjOXZtQAAAAAElFTkSuQmCC')
}
So it’s a standard prefix like data:image/png;base64,
followed by an indefinitely long string of ASCII gibberish, which is a textual base-64 encoding of the underlying binary data. The RNN sometimes starts a data URI and generates the prefix but then gets stuck continually producing hundreds or thousands of characters of ASCII gibberish without ever closing the data URI with a quote & parentheses and getting back to writing regular CSS.
What’s going on there? Since PNG/JPG are compressed image formats, the binary encoding will be near-random and the base-64 encoding likewise near-random. The RNN can easily generate another character once it has started the base-64, but how does it know when to stop? (“I know how to spell banana, I just don’t know when to stop! BA NA NA NA…”) Possibly it has run into the limits of its ‘memory’ and once it has started emitting base-64 and has reached a plausible length of at least a few score characters (few images can be encoded in less), it’s now too far away from the original CSS, and all it can see is base-64; so of course the maximal probability is an additional base-64 character…
This might be fixable by either giving the RNN more neurons in the hope that with more memory it can break out of the base-64 trap, training more (perhaps data URIs are too rare for it to have adequately learned it with the few epochs thus far), backpropagating error further in time/the sequence by increasing the size of the RNN in terms of unrolling (such as increasing -seq_length
from 50); I thought improving the sampling strategy with beam search rather than greedy character-by-character generation would help but it turns out beam search doesn’t fix it and can perform worse, getting trapped in an even deeper local minima of repeating the character “A” endlessly. Or of course one could delete data URIs and other undesirable features from the corpus, in which case those problems will never come up; still, I would prefer the RNN to handle issues on its own and have as little domain knowledge engineered in as possible. I wonder if the data URI issue might be what killed the large RNN at the end? (My other hypothesis is that the sort-key
trick accidentally led to a multi-megabyte set of repetitions of the same common CSS file, which caused the large RNN to overfit, and then once the training reached a new section of normal CSS, the large RNN began making extremely confident predictions of more repetition, which were wrong and would lead to very large losses, possibly triggering the exploding-error killer.)
Progress
This RNN progressed steadily over time, although by the end the performance on the held-out validation dataset seem to have been stagnating when I plot the validation tests:
performance <- dget(textConnection("structure(list(Epoch = c(0.13, 0.26, 0.4, 0.53, 0.66, 0.79, 0.92,
1.06, 1.19, 1.32, 1.45, 1.58, 1.71, 1.85, 1.98, 2.11, 2.24, 2.37,
2.51, 2.64, 2.77, 2.9, 3.03, 3.17, 3.3, 3.43, 3.56, 3.69, 3.82,
3.96, 4.09, 4.22, 4.35, 4.48, 4.62, 4.75, 4.88, 5.01, 5.14, 5.28,
5.41, 5.54, 5.67, 5.8, 5.94, 6.07, 6.2, 6.33, 6.46, 6.59, 6.73,
6.86, 6.99, 7.12, 7.25, 7.39, 7.52, 7.65, 7.78, 7.91, 8.05, 8.18,
8.31, 8.44, 8.57, 8.7, 8.84, 8.97, 9.1, 9.23, 9.36, 9.5, 9.63,
9.76, 9.89, 10.02, 10.16, 10.29, 10.42, 10.55, 10.68, 10.82,
10.95, 11.08, 11.21, 11.34, 11.47, 11.61, 11.74, 11.87, 12, 12.13,
12.27, 12.4, 12.53, 12.66, 12.79, 12.93, 13.06, 13.19, 13.32,
13.45, 13.58, 13.72, 13.85, 13.98, 14.11, 14.24, 14.38, 14.51,
14.64, 14.77, 14.9, 15.04, 15.17, 15.3, 15.43, 15.56, 15.7, 15.83,
15.96, 16.09, 16.22, 16.35, 16.49, 16.62, 16.75, 16.88, 17.01,
17.15, 17.28, 17.41, 17.54, 17.67, 17.81, 17.94, 18.07, 18.2,
18.33, 18.46, 18.6, 18.73, 18.86, 18.99, 19.12, 19.26, 19.39,
19.52, 19.65, 19.78, 19.92, 20.05, 20.18, 20.31, 20.44, 20.58,
20.71, 20.84, 20.97, 21.1, 21.23, 21.37, 21.5, 21.63, 21.76,
21.89, 22.03, 22.16, 22.29, 22.42, 22.55, 22.69, 22.82, 22.95,
23.08, 23.21, 23.34, 23.48, 23.61, 23.74, 23.87, 24, 24.14, 24.27,
24.4, 24.53, 24.66, 24.8, 24.93, 25.06, 25.19, 25.32, 25.46,
25.59, 25.72), Validation.loss = c(1.4991, 1.339, 1.3006, 1.2896,
1.2843, 1.1884, 1.1825, 1.0279, 1.1091, 1.1157, 1.181, 1.1525,
1.1382, 1.0993, 0.9931, 1.0369, 1.0429, 1.071, 1.08, 1.1059,
1.0121, 1.0614, 0.9521, 1.0002, 1.0275, 1.0542, 1.0593, 1.0494,
0.9714, 0.9274, 0.9498, 0.9679, 0.9974, 1.0536, 1.0292, 1.028,
0.9872, 0.8833, 0.9679, 0.962, 0.9937, 1.0054, 1.0173, 0.9486,
0.9015, 0.8815, 0.932, 0.9781, 0.992, 1.0052, 0.981, 0.9269,
0.8523, 0.9251, 0.9228, 0.9838, 0.9807, 1.0066, 0.8873, 0.9604,
0.9155, 0.9242, 0.9259, 0.9656, 0.9892, 0.9715, 0.9742, 0.8606,
0.8482, 0.8879, 0.929, 0.9663, 0.9866, 0.9035, 0.9491, 0.8154,
0.8611, 0.9068, 0.9575, 0.9601, 0.9805, 0.9005, 0.8452, 0.8314,
0.8582, 0.892, 0.9186, 0.9551, 0.9508, 0.9074, 0.7957, 0.8634,
0.8884, 0.8953, 0.9163, 0.9307, 0.8527, 0.8522, 0.812, 0.858,
0.897, 0.9328, 0.9398, 0.9504, 0.8664, 0.821, 0.8441, 0.8832,
0.8891, 0.9422, 0.953, 0.8326, 0.871, 0.8024, 0.8369, 0.8541,
0.895, 0.8892, 0.9275, 0.8378, 0.8172, 0.8078, 0.8353, 0.8602,
0.8863, 0.9176, 0.9335, 0.8561, 0.7952, 0.8423, 0.8833, 0.9052,
0.9202, 0.9354, 0.8477, 0.8271, 0.8187, 0.8714, 0.8714, 0.9089,
0.903, 0.9225, 0.8583, 0.7903, 0.8016, 0.8432, 0.877, 0.8825,
0.9323, 0.8243, 0.8233, 0.7981, 0.8249, 0.826, 0.9109, 0.8875,
0.9265, 0.8239, 0.8026, 0.7934, 0.851, 0.8856, 0.9033, 0.9317,
0.8576, 0.8335, 0.7829, 0.8172, 0.8658, 0.8976, 0.8756, 0.9262,
0.8184, 0.792, 0.7826, 0.8244, 0.861, 0.9144, 0.9244, 0.9106,
0.8327, 0.766, 0.7988, 0.8378, 0.8606, 0.8831, 0.9032, 0.8113,
0.8138, 0.7747, 0.8027, 0.8197, 0.8684, 0.874, 0.912)), .Names = c('Epoch',
'Validation.loss'), class = 'data.frame', row.names = c(NA, -195L
))"))
library(ggplot2)
qplot(Epoch, Validation.loss, data=performance) + stat_smooth()
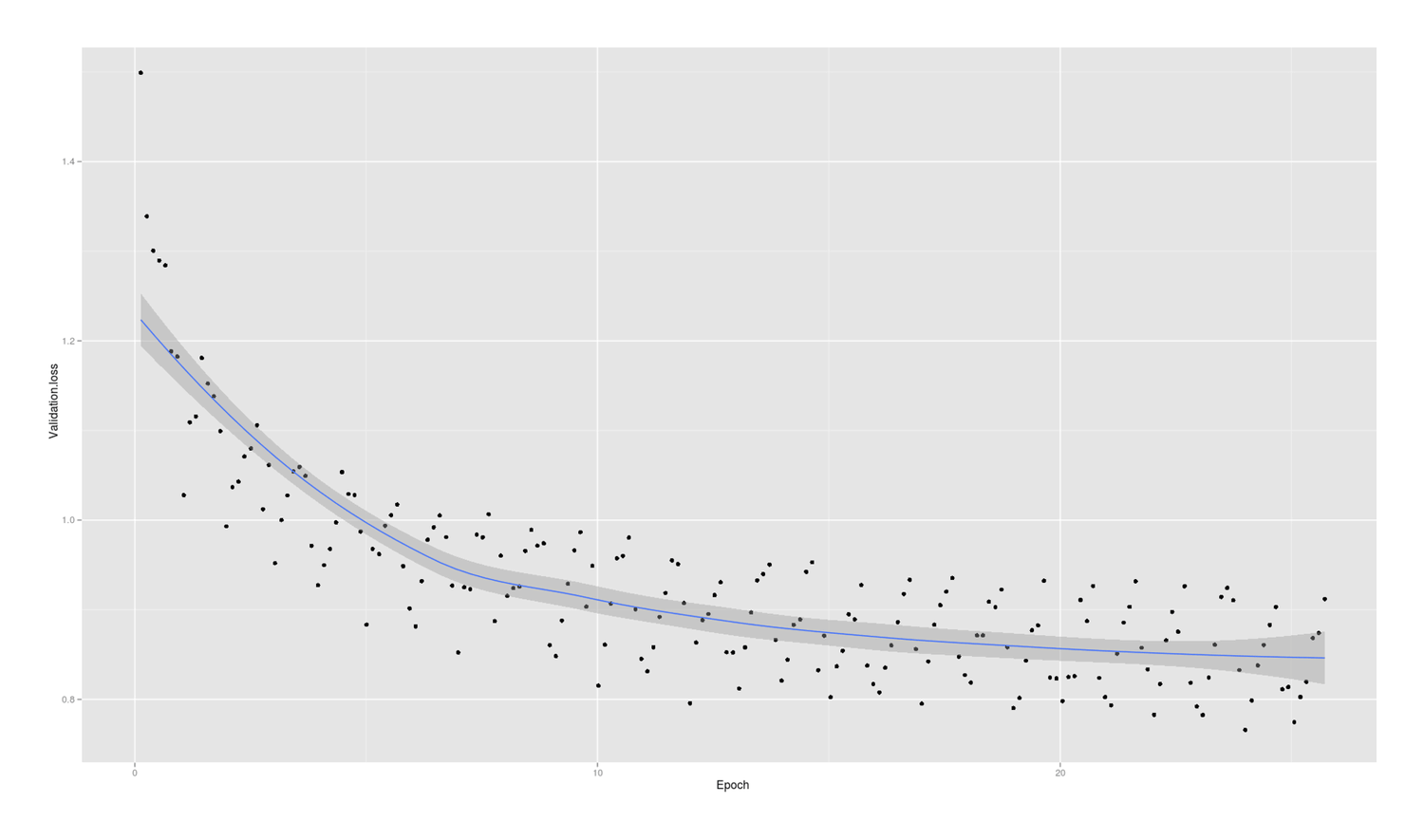
As the loss diminished to ~0.8-0.9, the sampled CSS output became even more realistic. At one point I was impressed to see that the RNN had learned to switch between “minified” and unminified CSS formatting. For example, above the output is unminified, but the RNN at 0.88 sometimes writes minified (following has been line-broken from a single line):
$ th sample.lua cv/lm_css_epoch6.07_0.8815.t7 -primetext 'div#sidebar { margin: 0px; }' -length 2000
div#sidebar { margin: 0px; }
#flashTopgip ul li h3 { clear: both; padding: 0; height:25px;
background:url(/images/exporibox.png) no-repeat 0
0;}.col_description{text-align:left!important;display:block;height:44px;top:-3px;left:68%;width:150px;}.front
.content
h3{display:inline-block;width:100%;position:fixed;position:absolute;left:0;}.date-repeat
#right{list-style:none;}.rtl
#block-agned-header{padding:10px;line-height:14px;width:auto;}#block-column-right{background:#63c;}.block-document_body
#content,.activism-content-box .content,.rtl .archive-wide
.button.input-rawignad{float:left;}.rtl .panel-pane .social-view table .lim
h1,.page-news h3.pane-title{*zoom:2 !important;}.rtl
.arow-right,#right-fields-img{display:none;}div.error{background-color:#ededed;}div.page-term
span.recimsregoor_contemt #aconds,.input-admin-widget-fill div.inner
.form-submit{display:block;margin-right:.1em;}#edit-activism-field-actionpoint{color:#8c0000;background:url(/sites/all/themes/zrw/sprites/hadd.png)
no-repeat 3px 0px;calse:0}.login-form p{margin:4px 25px;}.rtl
.note-ssTitle{margin:0 0 3px 0}ul.secondary
.page,#node-region{background:url(/sites/all/themes/rpg_theme/images/btn/form_subscription_not-page.png)
no-repeat 12px 0 #016 !important;}#network-footer:active{}#rtl
#newsletter-recitients-work_latest .center a{background-position:5px
-154px;}#product-item{margin-bottom:10px;}.node-type-article .home
.field-popup-widget-form{padding:20px 10px 10px 4px;text-align:right;}.rtl
.view-filters,.rtl #comments-albumang_sprite{float:left;}.node-type-nodes
.field-actionpoints-view-filters{padding:19px 28px 8px 0;}.rtl
#multimedia-latest .field-body,.view-content
div.field-view-layout{ulline-color:white;}.view-experts
.views-field-title{padding:4px;text-align:center;}.node-description
.views-exposed-form{overflow:visible;}#content .views-view-grid
tr.format{padding-bottom:10px;background:#030000;}.view-forword-source
.views-exposed-form #edit-submit{margin-right:0;}
This initially does not look impressive, but if we run it through a unminifier:
div#sidebar {
margin: 0px;
}
#flashTopgip ul li h3 {
clear: both;
padding: 0;
height: 25px;
background: url(/images/exporibox.png) no-repeat 0 0;
}
.col_description {
text-align: left!important;
display: block;
height: 44px;
top: -3px;
left: 68%;
width: 150px;
}
.front .content h3 {
display: inline-block;
width: 100%;
position: fixed;
position: absolute;
left: 0;
}
.date-repeat #right {
list-style: none;
}
.rtl #block-agned-header {
padding: 10px;
line-height: 14px;
width: auto;
}
#block-column-right {
background: #63c;
}
.block-document_body #content,
.activism-content-box .content,
.rtl .archive-wide .button.input-rawignad {
float: left;
}
.rtl .panel-pane .social-view table .lim h1,
.page-news h3.pane-title {
*zoom: 2 !important;
}
.rtl .arow-right,
#right-fields-img {
display: none;
}
div.error {
background-color: #ededed;
}
div.page-term span.recimsregoor_contemt #aconds,
.input-admin-widget-fill div.inner .form-submit {
display: block;
margin-right: .1em;
}
#edit-activism-field-actionpoint {
color: #8c0000;
background: url(/sites/all/themes/zrw/sprites/hadd.png) no-repeat 3px 0px;
calse: 0
}
.login-form p {
margin: 4px 25px;
}
.rtl .note-ssTitle {
margin: 0 0 3px 0
}
ul.secondary .page,
#node-region {
background: url(/sites/all/themes/rpg_theme/images/btn/form_subscription_not-page.png) no-repeat 12px 0 #016 !important;
}
#network-footer:active {}#rtl #newsletter-recitients-work_latest .center a {
background-position: 5px -154px;
}
#product-item {
margin-bottom: 10px;
}
.node-type-article .home .field-popup-widget-form {
padding: 20px 10px 10px 4px;
text-align: right;
}
.rtl .view-filters,
.rtl #comments-albumang_sprite {
float: left;
}
.node-type-nodes .field-actionpoints-view-filters {
padding: 19px 28px 8px 0;
}
.rtl #multimedia-latest .field-body,
.view-content div.field-view-layout {
ulline-color: white;
}
.view-experts .views-field-title {
padding: 4px;
text-align: center;
}
.node-description .views-exposed-form {
overflow: visible;
}
#content .views-view-grid tr.format {
padding-bottom: 10px;
background: #030000;
}
.view-forword-source .views-exposed-form #edit-submit {
margin-right: 0;
}
Now it’s readable and we can see the RNN has done an excellent job of still writing CSS while in minified-mode, and around this level of loss, I noticed the RNN had learned to write valid-looking URLs—fragments like background: url(/sites/all/themes/rpg_theme/images/btn/form_subscription_not-page.png)
look exactly like what a human CSS programmer would write. (Unfortunately, this sample has 4 validation errors: 1 from an imbalanced bracket; 1 one parse error on *zoom: 2 !important
due to the asterisk which is an old IE hack & arguably the RNN isn’t wrong; and 2 properties which don’t exist. Also in the RNN’s favor, I should note that lots of CSS in the wild will not have 0 validation errors.)
At 0.88, I also noticed the RNN was now making a valiant attempt to write comments. Bad comments, but still:
/* ubuntu@ip-172-31-30-222:~/char-rnn$ th sample.lua cv/lm_css_epoch6.07_0.8815.t7 -primetext 'div#sidebar { margin: 100px; }' -length 2000 -seed 1
using CUDA on GPU 0...
creating an lstm...
seeding with div#sidebar { margin: 100px; }
-------------------------- */
div#sidebar { margin: 100px; }
viv .yeah-company:first-child, .news-row0 .colsetIcob img,
.content .content-number { background-position: 0 -340px; text-decoration: repeat-x; }
#content .rcper { display:none; display: block;
}
#coftelNotif .topUy { background: url('/assets/css/epwide-datetherator.png'); }
#leftCol span.scord img { background: url(/img/text/about_links.png) no-repeat 0 -1050px; }
div.subkit_snav_created, ul.up_tains li.active { width: 64% !important; }
.hdr_outer {text-align:center; }
active, img {
top: auto;
margin-right: 20px;
margin: 0 !important;
text-align: center;
-webkit-box-shadow: #205575 1px 0 0 rgba(0,0,0,0.6) 1px 0px px;
box-shadow: 0 0 5px rgba(0,0,0,.5);
}
#ywip_section p.tab_promo,
#search_container #slideshow .page_inner #triabel_left {
background: url(drop, sanc-email' }
simple{
box-sizing: border-box;
}
span.naveptivionNav}
a.nav, pre,
html { */
background-color: #8ccedc;
background: #22a82c;
float: left;
color: #451515;
border: 1px solid #701020;
color: #0000ab;
font-family: Arial, sans-serif;
text-align: center;
margin-bottom: 50px;
line-height: 16px;
height: 49px;
padding: 15px 0 0 0;
font-size: 15px;
font-weight: bold;
background-color: #cbd2eb;
}
a.widespacer2,
#jomList, #frq {
margin: 0 0 0 0;
padding: 10px -4px;
background-color: #FFCFCF;
border: 1px solid #CBD7DD;
padding: 0 0 4px 12px;
min-height: 178px;
}
.eventmenu-item, .navtonbar .article ul, .creditOd_Dectls {
border-top: 1px #CCC gradsed 1px solid;
font-size: 0.75em;
}
h2,
div.horingnav img {
font-size: 5px;
}
body {
margin: 0 0 5px 20px;
}
.n-cmenuamopicated,
.teasicOd-view td {
border-top: 4px solid #606c98;
}
/* Rpp-fills*/
.ads{padding: 0 10px;}.statearch-header div.title img{display:table-call(}
fieldset legend span,
blockquote.inner ul {padding:0;}}
...
/* Ableft Title */
/* ======================================================== helper column parting if nofis calendar image Andy "Heading Georgia" */
.right_content {
position: relative;
width: 560px;
height: 94px;
}
Ultimately, the best RNN achieved a loss of 0.7660 before I decided to shut it down because it wasn’t making much further progress.
Samples
It stalwartly continued to try to write comments, approximating slightly English (even though there is not that much English text in those 20MB, only 8.5k lines with /*
in them - it’s CSS, not text). Examples of comments extracted from a large sample of 0.766’s output (grep -F '/*' best.txt
):
*//* COpToMNINW BDFER
/*
.snc .footer li a.diprActy a:hover, #sciam table {/*height: 164px;*//*/* }
body.node-type-xplay-info #newsletter,body.node-type-update
#header{min-width:128px;height:153px;float:left;}#main-content
.newsletternav,#ntype-audio
.block-title{background:url(/sites/www.amnesty.org/modules/civicrm/print-widget.clu))
/*gray details */
/* Grid >> 1px 0 : k0004_0 */
/* corner */
/* ST LETTOTE/ CORCRE TICEm langs 7 us1 Q+S. Sap q i blask */
/*/*/
/* Side /**/
/* Loading Text version Links white to 10ths */
/*-modaty pse */
/**/div#sb-adrom{display:none !important;}
/*
/* `Grid >> Global
/* `Grid >> 16 Columns
/* `Grid >> 16 Columns
/* `Suffix Extra Space >> 16 Columns
/* `Prefix Extra Space >> 12 Columns
/* `Prefix Extra Space >> 12 Columns
/* `Clear Floated Elements
/* `Prefix Extra Space >> 12 Columns
/* `Push Space >> 16 Columns
/* `Suffix Extra Space >> 16 Columns
/* `Suffix Extra Space >> 16 Columns
/* `Suffix Extra Space >> 16 Columns
/* `Prefix Extra Space >> 16 Columns
/* `Suffix Extra Space >> 16 Columns
/* IE7 inline-block hack */
/* T* */
Not too great, but still more than I expected Still, the (unminified) CSS looks good:
div#sidebar { margin: 100px; }
.ep_summary_box_body { float: left; width: 550px; }
.dark_search span { margin-right: 5px; }
h1.highlight_column { text-align: right; display: block; font-size: 18px; }
h3 {
font-weight: bold;
font-size: 12px;
}
col.teas h2 {
clear: both;
width: 100%;
z-index: 190;
action: !important;
}
#full_content .fancybox.no-float {
background-image: url('/static/onion/img/description.png');
max-width: 33px;
height: 40px;
margin-top: 20px;
color: #3D5042;
font-size: 0.75em;
padding-left: 25px !important;
}
.filter-container iframe{
width: 990px;
}
#funcy-oneTom {
margin: 0;
padding: 10px 1%;
line-height: 30px;
}
#utb_documentAlert {
color: #222;
}
#utb_column02 a.button:focus {
display: block;
font-family: Arial, Helvetica, sans-serif;
}
#utb_column02 ul.blogs-listing aundoc1 ul:before,
#utb_column01 a:active,
h1 { font-weight: bold; font-family: line-heetprind, AnimarzPromo, Atial; line-height: 1.4; font-size: 1 9px; }
#utb_column03 ul.fourder { width: 500px; padding: 4px 10px; }
The RNN also seems to have a thing for Amnesty International, regularly spitting out Amnesty URLs likeurl(/sites/www.amnesty.org/modules/civicrm/i/mast2adCbang.png)
(not actually valid URLs).
Once that was done, I generated samples from all the checkpoints:
for NN in cv/*.t7; do th sample.lua $NN -primetext 'div#sidebar { margin: 0px; }' -length 2000 > $NN.txt; done
## https://www.dropbox.com/s/xgstn9na3efxb43/smallrnn-samples.tar.xz
## if we want to watch the CSS evolve as the loss decreased:
for SAMPLE in `ls cv/lm_css*.txt | sort --field-separator="_" --key=4 --numeric-sort --reverse`;
do echo $SAMPLE: && tail -5 $SAMPLE | head -5; done
Evaluation
In under a day of GPU training on 20MB of CSS, a medium-sized RNN (~30M parameters) learned to produce high quality CSS, which passes visual inspection and on some batches yields few CSS syntactic errors. This strikes me as fairly impressive: I did not train a very large RNN, did not train it for very long, did not train it on very much, did no optimization of the many hyper-parameters, and it is doing unsupervised learning in the sense that it doesn’t know how well emitted CSS validates or renders in web browsers - yet the results still look good. I would say this is a positive first step.
Lessons learned:
-
GPUs > CPUs
-
char-rnn
, while rough-edged, is excellent for quick prototyping -
NNs are slow:
-
major computation is required for the best results
-
meaningful exploration of NN sizes or other hyperparameters will be challenging when a single run can cost days
-
-
computing large datasets or NNs on Amazon EC2 will entail substantial financial costs; it’s adequate for short runs but bills around $32.42$252015 for two days of playing around are not a long-term solution
-
pretraining an RNN on CSS may be useful for a CSS reinforcement learner
External Links
-
Discussion:
RNN: CSS → HTML
After showing good-looking CSS can be generated from learning on a CSS corpus and mastery of the syntactic rules, the next question is how to incorporate meaning. The generated CSS doesn’t mean anything and will only ‘do’ anything if it happens to have generated CSS modifying a sufficiently universal ID or CSS element (you might call the generated CSS ‘what the average CSS looks like’, although like the ‘average man’, average CSS does not exist in real life). We trained it to generate CSS from CSS. What if we trained it to generate CSS from HTML? Then we could feed in a particular HTML page and, if it has learned to generate meaningfully-connected CSS, then it should write CSS targeted on that HTML page. If a HTML page has a div
named lightbox
, then instead of the previous nonsense like .logo-events .show-luset .box-content li { width: 30px; }
, perhaps it will learn to write instead something meaningful like lightbox li { width: 30px; }
. (Setting that to 30px is not a good idea, but once it has learned to generate CSS for a particular page, then it can learn to generate good CSS for a particular page.)
Creating a Corpus
Before, creating a big CSS corpus was easy: simply find all the CSS files on disk, and cat them together into a single file which char-rnn
could be fed. From a supervised learning perspective, the labels were also the inputs. But to learn to generate CSS from HTML, we need pairs of HTML and CSS: all the CSS for a particular HTML page.
I could try to take the CSS files and work backwards to where the original HTML page may be, but most of them are not easily found and a single HTML page may call several CSS files or vice versa. It seems simpler instead to generate a fresh set of files by taking some large list of URLs, downloading each URL, saving its HTML and then parsing it for CSS links which then get downloaded and combined into a paired CSS file, with that single CSS file hopefully formatted and cleaned up in other ways.
I don’t know of any existing clean corpus of HTML/CSS pairs: existing databases like Common Crawl would provide more data than I need but in the wrong format (split over multiple files as the live website serves it), and I can’t reuse my current archive downloads (how to map all the downloaded CSS back onto their original HTML file and then combine them appropriately?). So I will generate my own.
I would like to crawl a wide variety of sites, particularly domains which are more likely to provide clean and high-quality CSS exercising lots of functionality, so I grab URLs from:
-
exports of my personal Firefox browsing history, URLs linked on Gwern.net, and URLs generated from my past archives
-
exports of the Hacker News submission history
-
the CSS Zen Garden (hundreds of pages with the same HTML but wildly different & carefully hand-written CSS)
Personal
To filter out useless URLs, files with bad extensions, & de-duplicate:
cd ~/css/
rm urls.txt
xzcat ~/doc/backups/urls/*-urls.txt.xz | cut --delimiter=',' --fields=2 | tr --delete "'" >> urls.txt
find ~/www/ -type f | cut -d '/' -f 4- | awk '{print "http://" $0}' >> urls.txt
find ~/wiki/ -name "*.md" -type f -print0 | parallel --null runghc ~/wiki/static/build/link-extractor.hs | grep -F http >> urls.txt
cat ~/.urls.txt >> urls.txt
firefox-urls >> urls.txt
sqlite3 -separator ',' -batch "$(find ~/.mozilla/firefox/ -name 'places.sqlite' | sort | head -1)" "SELECT datetime(visit_date/1000000,'unixepoch') AS visit_date, quote(url), quote(title), visit_count, frecency FROM moz_places, moz_historyvisits WHERE moz_places.id = moz_historyvisits.place_id and visit_date > strftime('\%s','now','-1 year)*1000000 ORDER by visit_date;" >> urls.txt
cat urls.txt | filter-urls | grep -E --invert-match -e '.onion/' -e '.css$' -e '.gif$' -e '.svg$' -e '.jpg$' -e '.png$' -e '.pdf$' -e 'ycombinator.com' -e 'reddit.com' -e 'nytimes.com' -e .woff -e .ttf -e .eot -e '\.css$'| sort | uniq --check-chars=18 | shuf >> tmp; mv tmp urls.txt
wc --lines urls.txt
## 136328 urls.txt
(uniq --check-chars=18
is there as a hack for deduplication: we don’t need to waste time on 1000 URLs all from the same domain, since their CSS will usually all be near-identical; this defines all URLs with the same first 18 characters as being duplicates and so to be removed.)
HN
HN:
wget 'https://archive.org/download/HackerNewsStoriesAndCommentsDump/HNStoriesAll.7z'
cat HNStoriesAll.json | tr ' ' '\n' | tr '"' '\n' | grep -E '^http://' | sort --unique >> hn.txt
cat hn.txt >> urls.txt
CSS Zen Garden
CSS Zen Garden:
nice linkchecker --complete -odot -v --ignore-url=^mailto --no-warnings --timeout=100 --threads=1 'https://www.csszengarden.com' | grep -F http | grep -F -v "label=" | grep -F -v -- "->" | grep -F -v '" [' | grep -F -v "/ " | sed -e "s/href=\"//" -e "s/\",//" | tr -d ' ' | filter-urls | tee css/csszengarden.txt # ]
cat csszengarden.txt | sort -u | filter-urls | grep -E --invert-match -e '.onion/' -e '.css$' -e '.gif$' -e '.svg$' -e '.jpg$' -e '.png$' -e '.pdf$' -e 'ycombinator.com' -e 'reddit.com' -e 'nytimes.com' -e .woff -e .ttf -e .eot -e '\.css$' > tmp
mv tmp csszengarden.txt
cat csszengarden.txt >> urls.txt
Downloading
To describe the download algorithm in pseudocode:
For each URL index i in 1:n:
download the HTML
parse
filter out `<link rel='stylesheet'>`, `<style>`
forall stylesheets,
download & concatenate into a single css
concatenate style into the single css
write html -> ./i.html
write css -> ./i.css
Downloading the HTML part of the URL can be done with wget as usual, but if instructed to --page-requisites
, it will spit CSS files over the disk and the CSS would need to be stitched together into one file. It would also be good if unused parts of the CSS could be ignored, the formatting be cleaned up & consistent across all pages, and while we’re wishing, JS evaluated just in case that makes a difference (since so many sites are unnecessarily dynamic these days). uncss
does all this in a convenient command-line format; the only downside I noticed is that it is inherently much slower, there is an unnecessary two-line header prefixed to the emitted CSS (specifying the URL evaluated) which is easily removed, and uncss
sometimes hangs & so something must be arranged to kill laggard instances so progress can be made. (Originally, I was looking for a tool which would download all the CSS on a page and emit it in a single stream/file rather than write my own tagsoup parser, but when I saw uncss
, I realized that the minimizing/optimizing was better than what I had intended and would be useful - why make the RNN learn CSS which isn’t used by the paired HTML?) Installing:
# Debian/Ubuntu workaround:
sudo ln -s /usr/bin/nodejs /usr/bin/node
# possibly helpful to pull in dependencies:
sudo apt-get install phantomjs
npm install -g path-is-absolute
npm install -g uncss --prefix ~/bin/
npm install -g brace-expansion --prefix ~/bin/
npm install -g uncss --prefix ~/bin/
Then having generated the URL list previously, it is simple to download each HTML/CSS pair:
downloadCSS () {
ID=`echo "$@" | md5sum | cut -f1 -d' '`
echo "$@":"$ID"
if [[ ! -s $IDcss ]]; then
timeout 120s wget --quiet "$@" -O $ID.html &
# `tail +3` gets rid of some uncss boilerplate
timeout 120s nice uncss --timeout 2000 "$@" | tail --lines=+3 >> $IDcss
fi
}
export -f downloadCSS
cat urls.txt | parallel downloadCSS
Screenshots: save as screenshot.js
var system = require('system');
var url = system.args[1];
var filename = system.args[2];
var WebPage = require('webpage');
page = WebPage.create();
page.open(url);
page.onLoadFinished = function() {
page.render(filename);
phantom.exit(); }
downloadScreenshot() {
echo "$@"
ID=`echo "$@" | md5sum | cut -f1 -d' '`
if [[ ! -a $ID.png ]]; then
timeout 120s nice phantomjs screenshot.js "$@" $ID.png && nice optipng -o9 -fix $ID.png
fi
}
export -f downloadScreenshot
cat urls.txt | nice parallel downloadScreenshot
After finish, find and delete duplicates with fdupes
; and delete any stray HTML/CSS:
# delete any empty file indicating CSS or HTML download failed:
find . -type f -size 0 -delete
# delete bit-identical duplicates:
fdupes . --delete --noprompt
# look for extremely similar screenshots, and delete all but the first such image:
nice /usr/bin/findimagedupes --fingerprints=./.fp --threshold=99% *.png | cut --delimiter=' ' --field=2- | xargs rm
# delete any file without a pair (empty or duplicate CSS having been previously deleted, now we clean up orphans):
orphanedFileRemover () {
if [[ ! -a $@.html || ! -a $@css ]];
then ls $@*; rm $@*;
fi; }
export -f orphanedFileRemover
find . -name "*css" -or -name "*.html" | sed -e 's/.html//' -e 'scss//' | sort --unique | parallel orphanedFileRemover
TODO: once the screenshotter has finished one full pass, then you can add image harvesting to enforce clean triplets of HTML/CSS/PNG
This yields a good-sized corpus of clean HTML/CSS pairs:
ls *css | wc --lines; cat *css | wc --char
TODO: yield seems low: 1 in 3? will this be enough even with 136k+ URLs? a lot of the errors seem to be sporadic and page downloads work when retrying them NYT seems to lock up uncss! had to filter it out, too bad, their CSS was nice and complex
Data Augmentation
Data augmentation is a way to increase corpus size by transforming each data point into multiple variants which are different on a low level but semantically are the same. For example, the best move in a particular Go board position is the same whether you rotate it by 45° or 180°; an upside-down or slightly brighter or slightly darker photograph of a fox is still a photograph of a fox, etc. By transforming them, we can make our dataset much larger and also force the NN to learn more about the semantics and not focus all its learning on mimicking surface appearances or making unwarranted assumptions. It seems to help image classification a lot (where the full set of data augmentation techniques used can be quite elaborate), and is a way you can address concerns about an NN not being robust to a particular kind of noise or transformation: you can include that noise/transformation as part of your data augmentation.
HTML and CSS can be transformed in various ways which textually look different but still mean the same thing to a browser: they can be minified, they can be reformatted per a style guide, some optimizations can be done to combine CSS declarations or write them in better ways, CSS files can be permuted (sometimes shuffling the order of declarations will change things by changing which of two overlapping declarations gets used, but apparently it’s rare in practice and CSS developers often write in random order), comments by definition can be deleted without affecting the displayed page,and so on.
TODO: use html5-tidy to clean up the downloaded html too? http://www.htacg.org/tidy-html5/documentation/#part_building keep both the original and clean version: this will be good data augmentation
Data augmentation:
-
raw HTML + uncss
-
tidy-html5 + uncss
-
tidy-html5 + csstidy(uncss)
-
tidy-html5 + minified CSS
-
tidy-html5 + shuffle CSS order as well? CSS is not fully but mostly declarative: https://www.w3.org/TR/2011/REC-CSS2-20110607/cascade.html#cascade
RNN
encoder-decoder? attention models? neural Turing machines?
-
http://devblogs.nvidia.com/parallelforall/introduction-neural-machine-translation-with-gpus/ / http://devblogs.nvidia.com/parallelforall/introduction-neural-machine-translation-gpus-part-2/ / http://devblogs.nvidia.com/parallelforall/introduction-neural-machine-translation-gpus-part-3/ implementation of encoder-decoder with attention in Theano: https://github.com/kyunghyuncho/dl4mt-material/tree/master/session2 “Currently, this code includes three subdirectories; session0, session1 and session2. session0 contains the implementation of the recurrent neural network language model using gated recurrent units, and session1 the implementation of the simple neural machine translation model. In session2, you can find the implementation of the attention-based neural machine translation model we discussed today. I am planning to make a couple more sessions, so stay tuned!”
-
possible example to steal from: https://github.com/nicholas-leonard/dp/blob/master/examples/recurrentlanguagemodel.lua / https://dp.readthedocs.org/en/latest/languagemodeltutorial/index.html#neural-network-language-model simple tutorial: https://dp.readthedocs.org/en/latest/neuralnetworktutorial/index.html (more lowlevel: https://github.com/Element-Research/rnn )
-
char-rnn seems too hardwired in character at a time, bidirectional?
-
pure Python implementation: https://github.com/karpathy/neuraltalk rip out the image stuff…?
-
https://github.com/wojzaremba/lstm
-
https://github.com/Element-Research/rnn#rnn.BiSequencerLM ?
-
http://www6.in.tum.de/pub/Main/Publications/Graves2008c.pdf
-
“Reinforcement Learning Neural Turing Machines” https://arxiv.org/abs/1505.00521
-
“Learning to Execute” https://arxiv.org/abs/1410.4615#google https://github.com/wojciechz/learning_to_execute
-
“Sequence to Sequence Learning with Neural Networks” https://arxiv.org/abs/1409.3215
-
“Generating Sequences With Recurrent Neural Networks” https://arxiv.org/abs/1308.0850
-
“Neural Turing Machines” https://arxiv.org/abs/1410.5401#deepmind
-
“DRAW: A Recurrent Neural Network For Image Generation” https://arxiv.org/abs/1502.04623
-
“Neural Machine Translation by Jointly Learning to Align and Translate” https://arxiv.org/abs/1409.0473 “In this paper, we conjecture that the use of a fixed-length vector is a bottleneck in improving the performance of this basic encoder-decoder architecture, and propose to extend this by allowing a model to automatically (soft-)search for parts of a source sentence that are relevant to predicting a target word, without having to form these parts as a hard segment explicitly. With this new approach, we achieve a translation performance comparable to the existing state-of-the-art phrase-based system on the task of English-to-French translation. Furthermore, qualitative analysis reveals that the (soft-)alignments found by the model agree well with our intuition.”
-
https://github.com/arctic-nmt/nmt
-
https://github.com/joschu/cgt/blob/master/examples/demo_neural_turing_machine.py
-
http://smerity.com/articles/2015/keras_qa.html
-
“Neural Transformation Machine: A New Architecture for Sequence-to-Sequence Learning”, et al 2015 https://arxiv.org/abs/1506.06442
-
“On End-to-End Program Generation from User Intention by Deep Neural Networks”, et al 2015 (almost too limited and simple, though)
Indentation + Left-Justified Text
Appendix
Covariate Impact On Power
Is it important in randomized testing of A/B versions of websites to control for covariates, even powerful ones? A simulation using a website’s data suggests that data is sufficiently large that it is not critical the way it is in many applications.
In December 2013, I was discussing website testing with another site owner, which monetizes traffic by selling a product, while I just optimize for reading time. He argued (deleting identifying details since I will be using their real traffic & conversion numbers throughout):
I think a big part that gets lost out is the quality of traffic. For our [next website version] (still speccing it all out), one of my biggest requirements for A/B testing is that all referring traffic must be bucketed and split-test against them. Buckets themselves are amorphous - they can be visitors of the same resolution, visitors who have bought our guide, etc. But just comparing how we did (and our affiliates did) on sales of our guide (an easy to measure metric - our RPU), traffic matters so much. X sent 5x the traffic that Y did, yet still generated 25% less sales. That would destroy any meaningful A/B testing without splitting up the quality.
I was a little skeptical that this was a major concern much less one worth expensively engineering into a site, and replied:
Eh. You would lose some power by not correcting for the covariates of source, but the randomization would still work and deliver you meaningful results. As long as visitors were being randomized into the A and B variants, and there was no gross imbalance in cells between Y and X, and Y and X visitors didn’t react differently, you’d still get the right results - just you would need more traffic to get the same statistical power. I don’t think 25% difference between X and Y visitors would even cost you that much power…
2013 note that:
…we conditioned on the user level covariates listed in the column labeled by the vector W in Table 1 using several methods to strengthen power; such panel techniques predict and absorb residual variation. Lagged sales are the best predictor and are used wherever possible, reducing variance in the dependent variable by as much as 40%…However, seemingly large improvements in R2 lead to only modest reductions in standard errors. A little math shows that going from R2 = 0 in the univariate regression to R2{|w} = 50% yields a sublinear reduction in standard errors of 29%. Hence, the modeling is as valuable as doubling the sample—a substantial improvement, but one that does not materially change the measurement difficulty. An order-of-magnitude reduction in standard errors would require R2{|w} = 99%, perhaps a “nearly impossible” goal.
In particular, if you lost a lot of power, wouldn’t that imply randomized trials were inefficient or impossible? The point of randomization is that it eliminates the impact of the indefinitely many observed and unobserved variables to let you do causal inference.
Power Simulation
Since this seems like a relatively simple problem, I suspect there is an analytic answer, but I don’t know it. So instead, we can set this up as a simulated power analysis: we generate random data where we force the hypothesis to be true by construction, we run our planned analysis, and we see how often we get a p-value underneath 0.05 (which is the true correct answer, by construction).
Let’s say Y’s visitors convert at 10%, then X’s must convert at 10% * 0.75, as he said, and let’s imagine our A/B test of a blue site-design increases sales by 1%. (So in the better version, Y visitors convert at 11% and X convert at 8.5%.) We generate n⁄4 datapoints from each condition (X/blue, X/not-blue, Y/blue, Y/not-blue), and then we do the usual logistic regression looking for a difference in conversion rate, with and without the info about the source. So we regress Conversion ~ Color, to look at what would happen if we had no idea where visitors came from, and then we regress Conversion ~ Color + Source
. These will spit out p-values on the Color
coefficient which are almost the same, but not quite the same: the regression with the Source
variable is slightly better so it should yield slightly lower p-values for Color
. Then we count up all the times the p-value was below the magical amount for each regression, and we see how many statistically-significant p-values we lost when we threw out Source
. Phew!
So we might like to do this for each sample size to get an idea of how they change. n = 100 may not the same for n = 10,000. And ideally, for each n, we do the random data generation step many times, because it’s a simulation and so any particular run may not be representative. Below, I’ll look at n = 1000, 1100, 1200, 1300, and so on up until n = 10,000. And for each n, I’ll generate 1000 replicates, which should be pretty accurate.
Large N
The whole schmeer in R:
set.seed(666)
yP <- 0.10
xP <- yP * 0.75
blueP <- 0.01
## examine various possible sizes of N
rm(controlledResults, uncontrolledResults)
for (n in seq(1000,10000,by=100)) {
rm(controlled, uncontrolled)
## generate 1000 hypothetical datasets
for (i in 1:1000) {
nn <- n/4
## generate 2x2=4 possible conditions, with different probabilities in each:
d1 <- data.frame(Converted=rbinom(nn, 1, xP + blueP), X=TRUE, Color=TRUE)
d2 <- data.frame(Converted=rbinom(nn, 1, yP + blueP), X=FALSE, Color=TRUE)
d3 <- data.frame(Converted=rbinom(nn, 1, xP + 0), X=TRUE, Color=FALSE)
d4 <- data.frame(Converted=rbinom(nn, 1, yP + 0), X=FALSE, Color=FALSE)
d <- rbind(d1, d2, d3, d4)
## analysis while controlling for X/Y
g1 <- summary(glm(Converted ~ Color + X, data=d, family="binomial"))
## pull out p-value for Color, which we care about; did we reach statistical-significance?
controlled[i] <- 0.05 > g1$coef[11]
## again, but not controlling
g2 <- summary(glm(Converted ~ Color , data=d, family="binomial"))
uncontrolled[i] <- 0.05 > g2$coef[8]
}
controlledResults <- c(controlledResults, (sum(controlled)/1000))
uncontrolledResults <- c(uncontrolledResults, (sum(uncontrolled)/1000))
}
controlledResults
uncontrolledResults
uncontrolledResults / controlledResults
Results:
controlledResults
# [1] 0.081 0.086 0.093 0.113 0.094 0.084 0.112 0.112 0.100 0.111 0.104 0.124 0.146 0.140 0.146 0.110
# [17] 0.125 0.141 0.162 0.138 0.142 0.161 0.170 0.161 0.184 0.182 0.199 0.154 0.202 0.180 0.189 0.202
# [33] 0.186 0.218 0.208 0.193 0.221 0.221 0.233 0.223 0.247 0.226 0.245 0.248 0.212 0.264 0.249 0.241
# [49] 0.255 0.228 0.285 0.271 0.255 0.278 0.279 0.288 0.333 0.307 0.306 0.306 0.306 0.311 0.329 0.294
# [65] 0.318 0.330 0.328 0.356 0.319 0.310 0.334 0.339 0.327 0.366 0.339 0.333 0.374 0.375 0.349 0.369
# [81] 0.366 0.400 0.363 0.384 0.380 0.404 0.365 0.408 0.387 0.422 0.411
uncontrolledResults
# [1] 0.079 0.086 0.093 0.113 0.092 0.084 0.111 0.112 0.099 0.111 0.103 0.124 0.146 0.139 0.146 0.110
# [17] 0.125 0.140 0.161 0.137 0.141 0.160 0.170 0.161 0.184 0.180 0.199 0.154 0.201 0.179 0.188 0.199
# [33] 0.186 0.218 0.206 0.193 0.219 0.221 0.233 0.223 0.245 0.226 0.245 0.248 0.211 0.264 0.248 0.241
# [49] 0.255 0.228 0.284 0.271 0.255 0.278 0.279 0.287 0.333 0.306 0.305 0.303 0.304 0.310 0.328 0.294
# [65] 0.316 0.330 0.328 0.356 0.319 0.310 0.334 0.339 0.326 0.366 0.338 0.331 0.374 0.372 0.348 0.369
# [81] 0.363 0.400 0.363 0.383 0.380 0.404 0.364 0.406 0.387 0.420 0.410
uncontrolledResults / controlledResults
# [1] 0.9753 1.0000 1.0000 1.0000 0.9787 1.0000 0.9911 1.0000 0.9900 1.0000 0.9904 1.0000 1.0000
# [14] 0.9929 1.0000 1.0000 1.0000 0.9929 0.9938 0.9928 0.9930 0.9938 1.0000 1.0000 1.0000 0.9890
# [27] 1.0000 1.0000 0.9950 0.9944 0.9947 0.9851 1.0000 1.0000 0.9904 1.0000 0.9910 1.0000 1.0000
# [40] 1.0000 0.9919 1.0000 1.0000 1.0000 0.9953 1.0000 0.9960 1.0000 1.0000 1.0000 0.9965 1.0000
# [53] 1.0000 1.0000 1.0000 0.9965 1.0000 0.9967 0.9967 0.9902 0.9935 0.9968 0.9970 1.0000 0.9937
# [66] 1.0000 1.0000 1.0000 1.0000 1.0000 1.0000 1.0000 0.9969 1.0000 0.9971 0.9940 1.0000 0.9920
# [79] 0.9971 1.0000 0.9918 1.0000 1.0000 0.9974 1.0000 1.0000 0.9973 0.9951 1.0000 0.9953 0.9976
So at n = 1000 we don’t have decent statistical power to detect our true effect of 1% increase in conversion rate thanks to blue - only 8% of the time will we get our magical p < 0.05 and rejoice in the knowledge that blue is boss. That’s not great, but that’s not what we were asking about.
Small N
Moving on to our original question, we see that the regressions controlling for source had a very similar power as to the regressions which didn’t bother. It looks like you may pay a small price of 2% less statistical power, but probably even less than that because so many of the other entries yielded an estimate of 0% penalty. And the penalty gets smaller as sample size increases and a mere 25% difference in conversion rate washes out as noise.
What if we look at smaller samples? say, n = 12-1012?
...
for (n in seq(12,1012,by=10)) {
... }
controlledResults
# [1] 0.000 0.000 0.000 0.001 0.003 0.009 0.010 0.009 0.024 0.032 0.023 0.027 0.033 0.032 0.045
# [16] 0.043 0.035 0.049 0.048 0.060 0.047 0.043 0.035 0.055 0.051 0.069 0.055 0.057 0.045 0.046
# [31] 0.037 0.049 0.057 0.057 0.050 0.061 0.055 0.054 0.053 0.062 0.076 0.064 0.055 0.057 0.064
# [46] 0.077 0.059 0.062 0.073 0.059 0.053 0.059 0.058 0.062 0.073 0.070 0.060 0.045 0.075 0.067
# [61] 0.077 0.072 0.068 0.069 0.082 0.062 0.072 0.067 0.076 0.069 0.074 0.074 0.062 0.076 0.087
# [76] 0.079 0.073 0.065 0.076 0.087 0.059 0.070 0.079 0.084 0.068 0.077 0.089 0.077 0.081 0.086
# [91] 0.094 0.080 0.080 0.087 0.085 0.087 0.082 0.084 0.073 0.083 0.077
uncontrolledResults
# [1] 0.000 0.000 0.000 0.001 0.002 0.009 0.005 0.007 0.024 0.031 0.023 0.024 0.033 0.032 0.044
# [16] 0.043 0.035 0.048 0.047 0.060 0.047 0.043 0.035 0.055 0.051 0.068 0.054 0.057 0.045 0.045
# [31] 0.037 0.048 0.057 0.057 0.050 0.060 0.055 0.054 0.053 0.062 0.074 0.063 0.055 0.057 0.059
# [46] 0.077 0.058 0.062 0.073 0.059 0.053 0.059 0.057 0.061 0.071 0.068 0.060 0.045 0.074 0.067
# [61] 0.076 0.072 0.068 0.069 0.082 0.062 0.072 0.066 0.076 0.069 0.073 0.073 0.061 0.074 0.085
# [76] 0.079 0.073 0.065 0.076 0.087 0.058 0.066 0.076 0.084 0.067 0.077 0.089 0.077 0.081 0.086
# [91] 0.094 0.080 0.080 0.087 0.085 0.087 0.080 0.081 0.071 0.083 0.076
uncontrolledResults / controlledResults
# [1] NaN NaN NaN 1.0000 0.6667 1.0000 0.5000 0.7778 1.0000 0.9688 1.0000 0.8889 1.0000
# [14] 1.0000 0.9778 1.0000 1.0000 0.9796 0.9792 1.0000 1.0000 1.0000 1.0000 1.0000 1.0000 0.9855
# [27] 0.9818 1.0000 1.0000 0.9783 1.0000 0.9796 1.0000 1.0000 1.0000 0.9836 1.0000 1.0000 1.0000
# [40] 1.0000 0.9737 0.9844 1.0000 1.0000 0.9219 1.0000 0.9831 1.0000 1.0000 1.0000 1.0000 1.0000
# [53] 0.9828 0.9839 0.9726 0.9714 1.0000 1.0000 0.9867 1.0000 0.9870 1.0000 1.0000 1.0000 1.0000
# [66] 1.0000 1.0000 0.9851 1.0000 1.0000 0.9865 0.9865 0.9839 0.9737 0.9770 1.0000 1.0000 1.0000
# [79] 1.0000 1.0000 0.9831 0.9429 0.9620 1.0000 0.9853 1.0000 1.0000 1.0000 1.0000 1.0000 1.0000
# [92] 1.0000 1.0000 1.0000 1.0000 1.0000 0.9756 0.9643 0.9726 1.0000 0.9870
As expected, with tiny samples like 12, 22, or 32, the A/B test has essentially 0% power to detect any difference, and so it doesn’t matter if one controls for source or not. In the n = 42+ range, we start seeing some small penalty, but the fluctuations from a 33% penalty to 0% penalty to 50% to 23% to 0% show that once we start nearing n = 100, the difference barely exists, and the long succession of 1.0000s say that past that, we must be talking a very small power penalty of like 1%.
Larger Differences
So let me pull up some real #s. I will give you source, # of unique visitors to sales page, # of unique visitors to buy page, # of actual buyers. Also note that I am doing it on a per-affiliate basis, and thus disregarding the origin of traffic (more on that later):
Website.com - 3963 - 722 - 293
X - 1232 - 198 - 8
Y - 1284 - 193 - 77
Z - 489 - 175 - 75
So even the origin of traffic was everywhere. X was all website, but pushed via FB. EC was email. Y was Facebook. Ours was 3 - email, Facebook, Twitter. Email converted at 13.72%, Facebook at 8.35%, and Twitter at 1.39%. All had >500 clicks.
So with that in mind, especially seeing how X and Y had the same # of people visit the buy page, but X converted at 10% the rate (and relatively to X, Y converted at 200%), I would wager that re-running your numbers would find that the origin matters.
Those are much bigger conversion differentials than the original 25% estimate, but the loss of power was so minute in the first case that I suspect that the penalty will still be relatively small.
I can fix the power analysis by looking at each traffic source separately and tweaking the random generation appropriately with liberal use of copy-paste. For the website, he said 3x500 but there’s 3963 hits so I’ll assume the remainder is your general organic website traffic. That gives me a total table:
-
Email:
500 * 13.72%
= 67 -
Facebook:
500 * 8.35%
= 42 -
Twitter:
500 * 1.39%
= 7 -
organic:
293-(67+42+7)
= 177;3963 - (3*500)
= 2463;177 / 2463
= 7.186%
Switching to R for convenience:
website <- read.csv(stdin(),header=TRUE)
Source,N,Rate
"X",1232,0.006494
"Y",1284,0.05997
"Z",489,0.1534
"Website email",500,0.1372
"Website Facebook",500,0.0835
"Website Twitter",500,0.0139
"Website organic",2463,0.07186
website$N / sum(website$N)
# [1] 0.17681 0.18427 0.07018 0.07176 0.07176 0.07176 0.35347
Change the power simulation appropriately:
set.seed(666)
blueP <- 0.01
rm(controlledResults, uncontrolledResults)
for (n in seq(1000,10000,by=1000)) {
rm(controlled, uncontrolled)
for (i in 1:1000) {
d1 <- data.frame(Converted=rbinom(n*0.17681, 1, 0.006494 + blueP), Source="X", Color=TRUE)
d2 <- data.frame(Converted=rbinom(n*0.17681, 1, 0.006494 + 0), Source="X", Color=FALSE)
d3 <- data.frame(Converted=rbinom(n*0.18427, 1, 0.05997 + blueP), Source="Y", Color=TRUE)
d4 <- data.frame(Converted=rbinom(n*0.18427, 1, 0.05997 + 0), Source="Y", Color=FALSE)
d5 <- data.frame(Converted=rbinom(n*0.07018, 1, 0.1534 + blueP), Source="Z", Color=TRUE)
d6 <- data.frame(Converted=rbinom(n*0.07018, 1, 0.1534 + 0), Source="Z", Color=FALSE)
d7 <- data.frame(Converted=rbinom(n*0.07176, 1, 0.1372 + blueP), Source="Website email", Color=TRUE)
d8 <- data.frame(Converted=rbinom(n*0.07176, 1, 0.1372 + 0), Source="Website email", Color=FALSE)
d9 <- data.frame(Converted=rbinom(n*0.07176, 1, 0.0835 + blueP), Source="Website Facebook", Color=TRUE)
d10 <- data.frame(Converted=rbinom(n*0.07176, 1, 0.0835 + 0), Source="Website Facebook", Color=FALSE)
d11 <- data.frame(Converted=rbinom(n*0.07176, 1, 0.0139 + blueP), Source="Website Twitter", Color=TRUE)
d12 <- data.frame(Converted=rbinom(n*0.07176, 1, 0.0139 + 0), Source="Website Twitter", Color=FALSE)
d13 <- data.frame(Converted=rbinom(n*0.35347, 1, 0.07186 + blueP), Source="Website organic", Color=TRUE)
d14 <- data.frame(Converted=rbinom(n*0.35347, 1, 0.07186 + 0), Source="Website organic", Color=FALSE)
d <- rbind(d1, d2, d3, d4, d5, d6, d7, d8, d9, d10, d11, d12)
g1 <- summary(glm(Converted ~ Color + Source, data=d, family="binomial"))
controlled[i] <- 0.05 > g1$coef[23]
g2 <- summary(glm(Converted ~ Color , data=d, family="binomial"))
uncontrolled[i] <- 0.05 > g2$coef[8]
}
controlledResults <- c(controlledResults, (sum(controlled)/1000))
uncontrolledResults <- c(uncontrolledResults, (sum(uncontrolled)/1000))
}
controlledResults
uncontrolledResults
uncontrolledResults / controlledResults
An hour or so later:
controlledResults
# [1] 0.105 0.175 0.268 0.299 0.392 0.432 0.536 0.566 0.589 0.631
uncontrolledResults
# [1] 0.093 0.167 0.250 0.285 0.379 0.416 0.520 0.542 0.576 0.618
uncontrolledResults / controlledResults
# [1] 0.8857 0.9543 0.9328 0.9532 0.9668 0.9630 0.9701 0.9576 0.9779 0.9794
In the most extreme case (total n = 1000), where our controlled test’s power is 0.105 or 10.5% (well, what do you expect from that small an A/B test?), our test where we throw away the Source info has a power of 0.093 or 9.3%. So we lost 0.1143 or 11% of the power.
Sample Size Implication
That’s not as bad as I feared when I saw the huge conversion rate differences, but maybe it has a bigger consequence than I guess?
What does this 11% loss translate to in terms of extra sample size?
Well, our original total conversion rate was 6.52%:
sum((website$N * website$Rate)) / sum(website$N)
# [1] 0.0652
We were examining a hypothetical increase by 1% to 7.52%. A regular 2-proportion power calculation (the closest thing to a binomial in the R standard library)
power.prop.test(n = 1000, p1 = 0.0652, p2 = 0.0752)
# Two-sample comparison of proportions power calculation
#
# n = 1000
# p1 = 0.0652
# p2 = 0.0752
# sig.level = 0.05
# power = 0.139
Its 14% estimate is reasonably close to 10.5% given all the simplifications I’m doing here. So, imagine our 0.139 power here was the victim of the 11% loss, and the true power is x = 0.11_x_ + 0.139 where then x = 0.15618. Given the p1 and p2 for our A/B test, how big would n then have to be to reach our true power?
power.prop.test(p1 = 0.0652, p2 = 0.0752, power=0.15618)
# Two-sample comparison of proportions power calculation
#
# n = 1178
So in this worst-case scenario with small sample size and very different true conversion rates, we would need another 178 page-views/visits to make up for completely throwing out the source covariate. This is usually a doable number of extra page-views.
Gwern.net
What are the implications for my own A/B tests, with less extreme “conversion” differences? It might be interesting to imagine a hypothetical where my traffic split between my highest conversion traffic source and my lowest, and see how much extra n I must pay in my testing because I decline to figure out how to record source for tested traffic.
Looking at my traffic for the year 2012-12-26-2013, I see that of the top 10 referral sources, the highest converting source is bulletproofexec.com traffic at 29.95% of the 9461 visits, and the lowest is t.co (Twitter) at 8.35% of 15168. We’ll split traffic 50/50 between these two sources.
set.seed(666)
## model specification:
bulletP <- 0.2995
tcoP <- 0.0835
blueP <- 0.0100
sampleSizes <- seq(100,5000,by=100)
replicates <- 1000
rm(controlledResults, uncontrolledResults)
for (n in sampleSizes) {
rm(controlled, uncontrolled)
# generate _m_ hypothetical datasets
for (i in 1:replicates) {
nn <- n/2
# generate 2x2=4 possible conditions, with different probabilities in each:
d1 <- data.frame(Converted=rbinom(nn, 1, bulletP + blueP), X=TRUE, Color=TRUE)
d2 <- data.frame(Converted=rbinom(nn, 1, tcoP + blueP), X=FALSE, Color=TRUE)
d3 <- data.frame(Converted=rbinom(nn, 1, bulletP + 0), X=TRUE, Color=FALSE)
d4 <- data.frame(Converted=rbinom(nn, 1, tcoP + 0), X=FALSE, Color=FALSE)
d0 <- rbind(d1, d2, d3, d4)
# analysis while controlling for Twitter/Bullet-Proof-Exec
g1 <- summary(glm(Converted ~ Color + X, data=d0, family="binomial"))
controlled[i] <- g1$coef[11] < 0.05
g2 <- summary(glm(Converted ~ Color , data=d0, family="binomial"))
uncontrolled[i] <- g2$coef[8] < 0.05
}
controlledResults <- c(controlledResults, (sum(controlled)/length(controlled)))
uncontrolledResults <- c(uncontrolledResults, (sum(uncontrolled)/length(uncontrolled)))
}
controlledResults
uncontrolledResults
uncontrolledResults / controlledResults
Results:
controlledResults
# [1] 0.057 0.066 0.059 0.065 0.068 0.073 0.073 0.071 0.108 0.089 0.094 0.106 0.091 0.110 0.126 0.112
# [17] 0.123 0.125 0.139 0.117 0.144 0.140 0.145 0.137 0.161 0.165 0.170 0.148 0.146 0.171 0.197 0.171
# [33] 0.189 0.180 0.184 0.188 0.180 0.177 0.210 0.207 0.193 0.229 0.209 0.218 0.226 0.242 0.259 0.229
# [49] 0.254 0.271
uncontrolledResults
# [1] 0.046 0.058 0.046 0.056 0.057 0.066 0.053 0.062 0.095 0.080 0.078 0.090 0.077 0.100 0.099 0.103
# [17] 0.109 0.113 0.118 0.105 0.134 0.130 0.123 0.124 0.142 0.152 0.153 0.133 0.126 0.151 0.168 0.151
# [33] 0.163 0.163 0.168 0.170 0.160 0.162 0.189 0.183 0.170 0.209 0.192 0.198 0.209 0.215 0.233 0.208
# [49] 0.221 0.251
uncontrolledResults / controlledResults
# [1] 0.8070 0.8788 0.7797 0.8615 0.8382 0.9041 0.7260 0.8732 0.8796 0.8989 0.8298 0.8491 0.8462
# [14] 0.9091 0.7857 0.9196 0.8862 0.9040 0.8489 0.8974 0.9306 0.9286 0.8483 0.9051 0.8820 0.9212
# [27] 0.9000 0.8986 0.8630 0.8830 0.8528 0.8830 0.8624 0.9056 0.9130 0.9043 0.8889 0.9153 0.9000
# [40] 0.8841 0.8808 0.9127 0.9187 0.9083 0.9248 0.8884 0.8996 0.9083 0.8701 0.9262
1 - mean(uncontrolledResults / controlledResults)
# [1] 0.1194
So our power loss is not too severe in this worst-case scenario: we lose a mean of 12% of our power, or around half.
We were examining a hypothetical conversion increase by 1% from 19.15% (mean(c(bulletP, tcoP))
) to 20.15%. A regular 2-proportion power calculation (the closest thing to a binomial in the R standard library)
power.prop.test(n = 1000, p1 = 0.1915, p2 = 0.2015)
# Two-sample comparison of proportions power calculation
#
# n = 1000
# p1 = 0.1915
# p2 = 0.2015
# sig.level = 0.05
# power = 0.08116
Its 14% estimate is reasonably close to 10.5% given all the simplifications I’m doing here. So, imagine our 0.08116 power here was the victim of the 12% loss, and the true power is x = 0.12_x_ + 0.08116 where then x = 0.0922273. Given the p1 and p2 for our A/B test, how big would n then have to be to reach our true power?
power.prop.test(p1 = 0.1915, p2 = 0.2015, power=0.0922273)
# Two-sample comparison of proportions power calculation
#
# n = 1265
So this worst-case scenario means I must spend an extra n of 265 or roughly a fifth of a day’s traffic. Since it would probably cost me, on net, far more than a fifth of a day to find an implementation strategy, debug it, and incorporate it into all future analyses, I am happy to continue throwing out the source information & other covariates.
-
The loss here seems to be the average Negative Log Likelihood of each character; so a training loss of 3.78911860 means
exp(-3.78911860) → 0.02
or 2% chance of predicting the next character. This is not better than the base-rate of uniformly guessing each of the 128 ASCII characters, which would yield1/128 → 0.0078125
or 0.7% chance. However, after a few hours to train and getting down to ~0.8, then it’s starting to become quite impressive: 0.8 here translates to a 45% chance - not shabby! At that point, the RNN is starting to become a good natural-language compressor as it’s approaching estimates of the entropy of natural human English and RNNs have gotten close to records like 1.278 bits per character. (Which, after converting to bits per character, implies that for English text similarly complicated as Wikipedia, we shouldn’t expect our RNN to do any better than a training loss of ~0.87 and more realistically 0.9-1.1.)↩︎ -
Several days after I gave up, Nvidia released a 7.5 RC which did claim to support Ubuntu 15.04, but installing it yielded the same lockup. I then installed Ubuntu 14.04 and tried the 14.04 version of that 7.5 RC, and that worked flawlessly for GPU acceleration of both graphics & NNs.↩︎
-
Eventually the Nvidia release caught up with 15.04 and I was able to use the Acer laptop for deep learning. This may not have been a good thing in the long run because the laptop wound up being bricked on 2016-11-26, with what I think was the motherboard dying, when it was just out of warranty, and corrupting the filesystem on the SSD to boot. This is an odd way for a laptop to die, and perhaps the warnings against using laptop GPUs for deep learning were right - the laptop was indeed running
torch-rnn
the night/morning it died.↩︎ -
The EC2 price chart describes it as “High-performance NVIDIA GPUs, each with 1,536 CUDA cores and 4GB of video memory”. These apparently are NVIDIA Quadro K5000 cards, which cost somewhere around $1945.36$15002015. (Price & performance-wise, it seems there are these days a lot of better options now; for example, my GeForce GTX 960M seems to train at similar speed at the EC2 instances do.) At $0.84$0.652015/hr, that’s ~2300 hours or 96 days; at spot, 297 days. Even adding in local electricity cost and the cost of building a desktop PC around the GPUs, it’s clear that breakeven is under a year and that for more than the occasional dabbling, one’s own hardware is key. If nothing else, you won’t feel anxious about the clock ticking on your Amazon bill!↩︎