Analysis of whether bitter melon reduces blood glucose in one self-experiment and utility of further self-experimentation
I re-analyze a bitter-melon/blood-glucose self-experiment, finding a small effect of increasing blood glucose after correcting for temporal trends & daily variation, giving both frequentist & Bayesian analyses. I then analyze the self-experiment from a subjective Bayesian decision-theoretic perspective, cursorily estimating the costs of diabetes & benefits of intervention in order to estimate Value Of Information for the self-experiment and the benefit of further self-experimenting; I find that the expected value of more data (EVSI) is negative and further self-experimenting would not be optimal compared to trying out other anti-diabetes interventions.
Bitter melon is an Asian fruit which may reduce blood glucose levels (the studies apparently conflict and one might be a priori dubious of it1). In June 2015, Paul LaFontaine ran a self-experiment on 900mg of bitter melon extract taken with breakfast (Vitamin World brand: 100x450mg, $25), randomized daily for 20 days, measuring blood glucose levels with a normal fingerprick test kit before breakfast, at 10AM, and 3PM; the 20-day randomization was followed by a single block of 13 days with bitter melon use. There was no placebo-control or blinding.
LaFontaine reports that his t-tests indicates no statistically-significant effects, and point-values indicating bitter melon harmfully increases blood glucose.
Visualizing
The first thing to note is that taking his data, converting to long format, and plotting it:
melon <- read.csv(stdin(), header=TRUE)
Date,Exercise,Bitter.Melon,Read.wake,Read.10am,Read.3pm
2015-08-09,1,1,105,140,99
2015-08-10,1,1,100,106,88
2015-08-11,1,0,99,111,91
2015-08-12,1,0,103,100,86
2015-08-13,1,1,91,101,87
2015-08-14,1,0,92,114,92
2015-08-15,1,1,112,116,103
2015-08-16,0,0,108,105,93
2015-08-17,1,1,115,113,114
2015-08-18,0,1,115,118,109
2015-08-19,1,1,105,109,105
2015-08-20,1,0,111,116,98
2015-08-21,1,0,109,111,103
2015-08-22,1,1,102,120,NA
2015-08-23,1,1,108,93,134
2015-08-24,1,1,115,101,113
2015-08-25,1,0,114,117,105
2015-08-26,1,0,114,108,113
2015-08-27,0,1,118,114,121
2015-08-28,0,0,104,NA,100
2015-08-29,1,0,105,NA,100
2015-08-30,0,0,NA,NA,105
2015-08-31,0,1,113,NA,118
2015-09-01,0,1,112,120,NA
2015-09-02,0,1,128,113,NA
2015-09-03,0,1,112,115,NA
2015-09-04,0,1,104,112,86
2015-09-05,0,1,98,NA,NA
2015-09-06,1,1,96,NA,NA
2015-09-07,1,1,117,NA,119
2015-09-08,0,1,102,119,NA
2015-09-09,0,1,114,NA,94
2015-09-10,0,1,119,118,NA
2015-09-11,0,1,NA,NA,126
2015-09-12,1,1,122,126,NA
library(reshape)
melon2 <- reshape(melon, varying=c("Read.wake", "Read.10am", "Read.3pm"), timevar="Date", direction=long)
melon2 <- melon2[order(melon2$Date),]
library(ggplot2)
qplot(Date, Read, color=as.logical(Bitter.Melon), data=melon2) +
geom_smooth(aes(group=1)) + geom_point(size=I(4)) + theme(legend.position = "none")
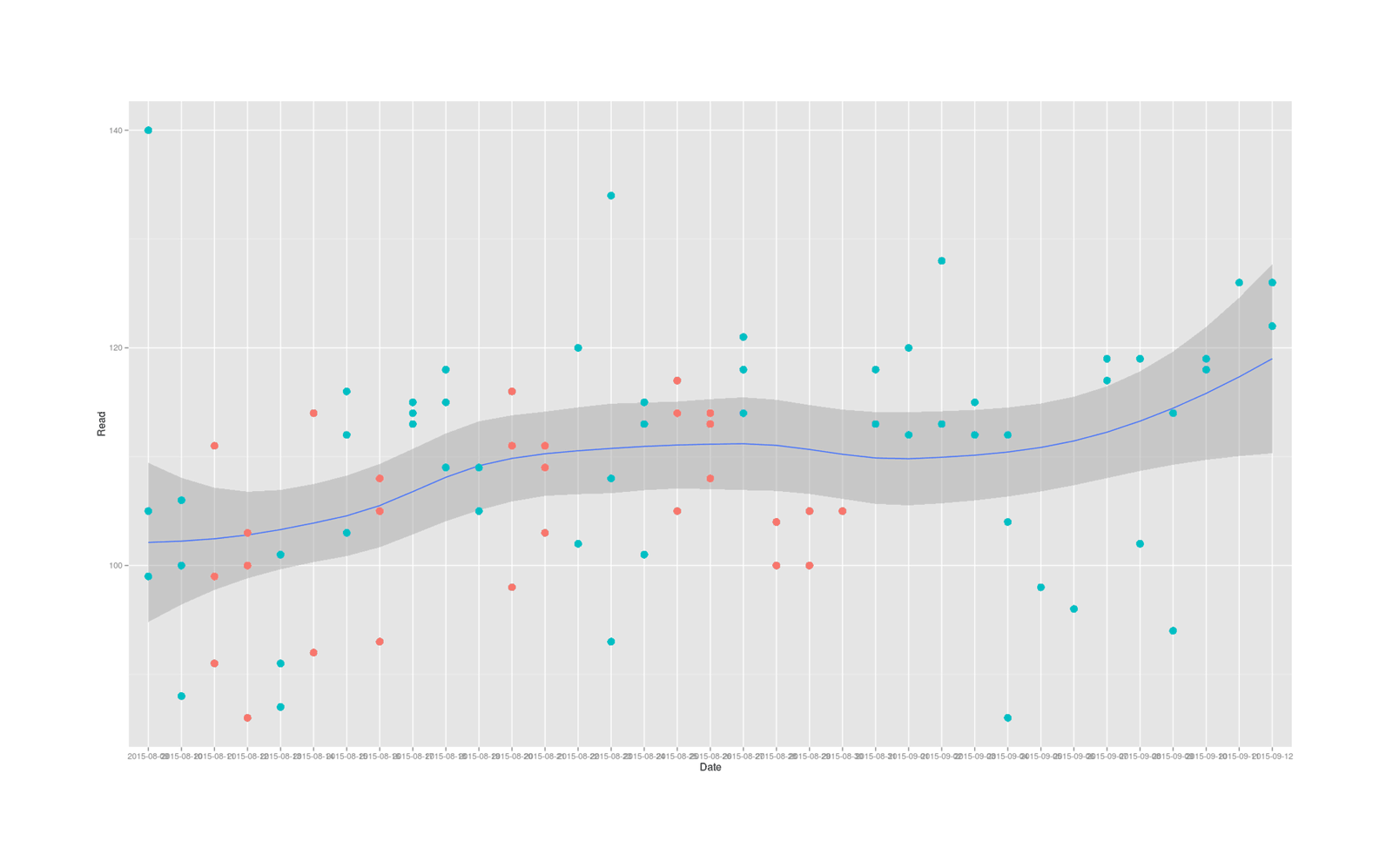
Has an unmistakable time trend of the blood glucose levels increasing almost linearly over time.
Analysis
So while LaFontaine is certainly correct that bitter melon does not statistically-significantly lower total daily blood glucose levels, and the point-estimates are disturbing large:
with(melon, t.test((Read.wake+Read.10am+Read.3pm) ~ Bitter.Melon))
# Welch Two Sample t-test
#
# data: (Read.wake + Read.10am + Read.3pm) by Bitter.Melon
# t = -1.0975011, df = 16.927915, p-value = 0.2877892
# alternative hypothesis: true difference in means is not equal to 0
# 95% confidence interval:
# -30.459040732 9.618131641
# sample estimates:
# mean in group 0 mean in group 1
# 314.1250000 324.5454545
with(melon, wilcox.test((Read.wake+Read.10am+Read.3pm) ~ Bitter.Melon, conf.int=TRUE))
# Wilcoxon rank sum test with continuity correction
#
# data: (Read.wake + Read.10am + Read.3pm) by Bitter.Melon
# W = 29.5, p-value = 0.2472612
# alternative hypothesis: true location shift is not equal to 0
# 95% confidence interval:
# -35.999976618 7.000064149
# sample estimates:
# difference in location
# -11.2627309
Neither the t-test nor U test can yield valid results because the i.i.d. assumption is violated (datapoints come from different distributions depending on what time they were collected), and since bitter melon is backloaded in the final 13 days where blood glucose is higher than ever (for unknown reasons), that alone could drive the estimates of harm. So the temporal trend needs to be modeled somehow; one way, without going to full-blown time-series models, is to regress on the index of the date
Another thing to notice in the plot is that blood glucose tests are both highly variable within a day, and highly variable between days as well. The variability between days implies that a multilevel model here would be good. The variability within days, on the other hand, implies that the different measurement times should be treated as covariates themselves, but also that we should remember that home blood glucose tests are not infinitely precise but the manufacturers claim an accuracy of something like ±5ng/ml (and in using my own blood glucose test strips, I find they can be much more noisy than that), so modeling the measurement-error would be worthwhile.
A first stab at controlling for the temporal effect reduces the bitter melon estimate a bit to 9.7:
summary(lm(I(Read.wake + Read.10am + Read.3pm) ~ Bitter.Melon + Exercise + as.integer(Date), data=melon))
# ...Coefficients:
# Estimate Std. Error t value Pr(>|t|)
# (Intercept) 300.0368557 18.3528242 16.34827 5.7259e-11
# Bitter.Melon 9.7182611 10.0285767 0.96906 0.34788
# Exercise 2.7759120 13.3097375 0.20856 0.83760
# as.integer(Date) 1.1515280 0.8078333 1.42545 0.17450
#
# Residual standard error: 21.23433 on 15 degrees of freedom
# (16 observations deleted due to missingness)
# Multiple R-squared: 0.1810341, Adjusted R-squared: 0.01724088
# F-statistic: 1.10526 on 3 and 15 DF, p-value: 0.3777754
Switching to long format lets us immediately fit a multilevel model with random effects for days and also treating time of day as a covariate, where the bitter melon estimate has now dropped by a third, to 3.7:
library(lme4)
mlm <- lmer(Read ~ Exercise + Bitter.Melon + Measurement + as.integer(Date) + (1|Date), data=melon2)
summary(mlm)
# ...Random effects:
# Groups Name Variance Std.Dev.
# Date (Intercept) 13.44398 3.666603
# Residual 79.75841 8.930756
# Number of obs: 85, groups: Date, 35
#
# Fixed effects:
# Estimate Std. Error t value
# (Intercept) 104.5433728 4.2378163 24.66916
# Exercise 0.8480363 2.8328254 0.29936
# Bitter.Melon 3.7739611 2.6027090 1.45001
# as.integer(Date) 0.3386202 0.1422834 2.37990
# Measurement3pm -8.4730381 2.5321712 -3.34616
# Measurementwake -4.9616823 2.3702767 -2.09329
#
# Correlation of Fixed Effects:
# (Intr) Exercs Bttr.M as.(D) Msrmn3
# Exercise -0.726
# Bitter.Meln -0.355 0.095
# as.ntgr(Dt) -0.649 0.495 -0.209
# Measrmnt3pm -0.337 0.017 0.093 -0.012
# Measurmntwk -0.273 -0.012 0.022 -0.086 0.527
confint(mlm)
# 2.5 % 97.5 %
# .sig01 0.00000000000 6.2022516958
# .sigma 7.30173759853 10.6754722267
# (Intercept) 96.53558709879 112.5507720395
# Exercise -4.51695529051 6.2003858378
# Bitter.Melon -1.15069394879 8.6949759136
# as.integer(Date) 0.06908453319 0.6074697878
# Measurement3pm -13.39921098464 -3.5485825386
# Measurementwake -9.49214591713 -0.4055767680
Blood glucose measurements definitely differ by time of day, and days do cluster, so this model works much better than the linear model or t-test did. Critically, we see that the bitter melon estimate is still much smaller than what we had from before, having dropped to a quarter of the effect size; this shows that the violation of assumptions was driving much of the apparent harm.
As far as measurement error goes, that can be modeled by linear measurement error models/error-in-variable models/Deming regression/total least squares/orthogonal regression, but these usually seem to assume that you have multiple measurements of the same datapoint (if LaFontaine had measured 3 times immediately in the morning for each day, instead of once at 3 different times of day) from which the size of the error can be estimated, while here we just have prior information.
JAGS
So that motivates a switch to Bayesian modeling using JAGS. Here we have a multilevel (for days) measurement-error (blood glucose levels treated as a latent variable) model with fixed-effects for exercise, bitter melon, date index, and 3 times of day (manually turned into dummy variables since unlike lm
and lmer
, JAGS doesn’t automatically expand a factor into multiple dummy variables):
library(runjags)
model1 <- "model {
delta.between.group ~ dunif(0, 30)
tau.between.group <- pow(sigma.between.group, -2)
sigma.between.group ~ dunif(0, 10)
for (j in 1:m) {
b3_1[j] ~ dnorm(delta.between.group, tau.between.group)
}
for (i in 1:n) {
Blood.noise[i] ~ dnorm(0, tau.Blood.noise)
Blood.hat[i] <- a + b1*Blood.noise[i] + b4*Exercise[i] + b2*Bitter.Melon[i] +
b3_1[Date[i]] + b3_2*Date[i] + b5*Morning[i] + b6*am10[i] + b7*pm3[i]
Blood[i] ~ dnorm(Blood.hat[i], tau)
}
a ~ dnorm(0, .001)
b1 ~ dnorm(0, .001)
b2 ~ dnorm(0, .001)
b3_2 ~ dnorm(0, .001)
b4 ~ dnorm(0, .001)
b5 ~ dnorm(0, .001)
b6 ~ dnorm(0, .001)
b7 ~ dnorm(0, .001)
sigma ~ dunif(0, 20)
tau <- pow(sigma, -2)
# SD of LaFontaine's blood glucose measurements is ~9.2,
# manufacturers claim within ~5 accuracy, so use that as the
# prior for the accuracy of blood glucose measurements:
tau.Blood.noise <- 1 / pow((5/9.2), 2)
}"
j1 <- with(melon2, run.jags(model1, data=list(n=nrow(melon2), Blood=Read, Bitter.Melon=Bitter.Melon,
Date=as.integer(Date), m=length(levels(Date)), Exercise=Exercise,
Morning=as.integer(Measurement=="wake"), am10=as.integer(Measurement=="10am"),
pm3=as.integer(Measurement=="3pm")),
monitor=c("b1", "b2", "b3_2", "b4", "b5", "b6", "b7"), sample=500000))
j1
# JAGS model summary statistics from 1000000 samples (chains = 2; adapt+burnin = 5000):
#
# Lower95 Median Upper95 Mean SD Mode MCerr MC%ofSD SSeff AC.500 psrf
# b1 -17.787 0.42309 17.967 0.33449 11.746 -- 0.27033 2.3 1888 0.17493 1.0003
# b2 -1.172 3.9699 9.3653 3.9906 2.6803 -- 0.026348 1 10348 0.017809 1
# b3_2 0.076985 0.36628 0.65914 0.36598 0.14869 -- 0.0015911 1.1 8733 0.017328 1.0002
# b4 -4.3368 1.3828 7.1201 1.3751 2.9205 -- 0.031235 1.1 8742 0.015053 1.0006
# b5 -10.561 19.604 53.525 19.768 16.298 -- 0.52169 3.2 976 0.39992 1.0038
# b6 -7.4183 24.57 56.427 24.724 16.304 -- 0.52847 3.2 952 0.39991 1.0042
# b7 -14.914 16.107 49.277 16.305 16.324 -- 0.53595 3.3 928 0.40045 1.0039
So here the mean estimate for bitter melon, b2
, is 3.9 with negative values still possible. (Curiously, b4
, whether LaFontaine exercised the morning of that day, is close to zero, though you would expect exercise to reduce blood glucose levels. Exercise may take time to kick in, so I wonder if I should have been treating it as a lagged variable and including a variable for having exercised the day before?)
We can also see how the posterior distribution of the bitter melon parameter evolves with the data:
library(animation)
saveGIF(
for(n in 1:nrow(melon2)){
newData <- melon2[1:n,]
j <- with(newData, run.jags(model1, data=list(n=nrow(newData), Blood=Read, Bitter.Melon=Bitter.Melon,
Date=as.integer(Date), m=length(levels(Date)), Exercise=Exercise,
Morning=as.integer(Measurement=="wake"),
am10=as.integer(Measurement=="10am"), pm3=as.integer(Measurement=="3pm")),
monitor=c("b2"), sample=6000, silent.jags=TRUE, summarise=FALSE))
coeff <- as.mcmc.list(j, vars="b2")
p <- qplot(as.vector(coeff[[1]]), binwidth=1) +
coord_cartesian(xlim = c(-11, 11)) +
ylab("Posterior density") + xlab("Effect on blood glucose (ng/ml)") + ggtitle(n) +
theme(axis.text.y=element_blank())
print(p)
},
interval = 0.5, ani.width = 800, ani.height=800,
movie.name = "/home/gwern/wiki/images/nootropics/2015-lafontaine-bittermelon-samplebysample.gif")
Simulated data: posterior estimates evolving sample by sample
We can see by eye that by the final measurements, the probability that bitter melon’s effect size is negative (reduces blood glucose) has become small because so little of the posterior distribution falls below zero. The mean of the bitter melon winds up not changing our estimate noticeably, but going fully Bayesian does have some nice side-effects like giving us something far more interpretable than a non-statistically-significant p or t-value—the posterior probability bitter melon reduces blood glucose levels, which in this case is:
coeff <- as.mcmc.list(j1, vars="b2")
sum(coeff[[1]]<0) / length(coeff[[1]])
# [1] 0.067156
So the posterior probability that bitter melon lowers blood glucose in this self-experiment is 7%.
Cost-Benefit
As well, LaFontaine is concerned to optimize his health and financial expenditures while not spending too much effort testing out an intervention:
As a result of this analysis I will no longer take Bitter Melon and save myself the money…I try to balance the strength of the statistics with pragmatic “no go” decisions on supplements and other mechanisms.
With the posterior distribution from the Bayesian model, we could examine this question directly: what is the current value of bitter melon and is the current experiment sufficient to rule out bitter melon use, or rule out collecting additional data?
To do a cost-benefit, we assign costs to the use of bitter melon, risk & cost of developing diabetes, estimate how much a reduction in blood glucose reduces diabetes risk, and then we can work with the posterior distribution to estimate
Cost-benefit:
-
cost of bitter melon use: some browsing suggests that a good buy would be 120x600mg at $14; 600mg total is a recommended dose for extract, so this is 120 days’ worth at $0.12/day or $44/year or, for indefinite consumption discounted at 5% annually ()
-
cost of diabetes: while not familiar with the literature, it’s clear that diabetes is extremely expensive in every way: substantial ongoing costs to monitor blood glucose (the cheapest possible test strips are still like $0.17, which at 3+ times a day adds up), there are serious side-effects like blindness, increased rates of other diseases like cancer (themselves expensive), life-expectancy reductions etc Just the medical expenditures could easily be $124,600 (NPV) if developed at age 40. (I learned after finishing that LaFontaine is older than that so a better figure would have been $53-91,000. Another good source of information would be Global Burden of Disease.) So avoiding it is important.
-
How much does a reduction in blood glucose reduce the risk of diabetes, and how much is any given reduction in risk itself worth compared to the annual cost of bitter melon? My strategy here is to look at RCTs of how much drugs reduce blood glucose, how much drugs reduce diabetes rates, and assume that the drugs are exerting this effect through the blood glucose reduction and define the reduction in diabetes risk per ng/ml accordingly. Here too I am not familiar with the large literature, so what I did was I looked through one of the more recent meta-analyses; that and other meta-analyses didn’t include reductions in blood glucose or any estimate of the kind I wanted, unfortunately, so I then looked for the largest single study included. It found that in their sample, the fasting blood glucose went from 5.8mmol/L on placebo to 5.4mmol/L in their intervention (104.5 vs 97.3), and this was associated with the intervention group having 60% of the risk as the control group2. (We could also try looking at existing decision-theory treatments of diabetes interventions like et al 2010 .)
If the male American lifetime risk for diabetes is 0.328, and the cost of diabetes is at least $124,600, then the expected loss is ; if an intervention lowering by 7.2ng/ml is done and thus then risk is reduced by 60% to 40% of what it was, then the expected loss is or a reduction in loss of $16,347.52. Assuming linear response, then each ng/ml reduction of 1 was worth .
So for example, we might ask for the probability bitter melon reduces blood glucose by >=1 ng/ml:
sum(coeff[[1]]<(-1)) / length(coeff[[1]])
# [1] 0.030978
3.1% is not much, but the expected value of >=1ng/ml is >$70.33 () ie. it’s worth more than 1 year of bitter melon would cost—although still not more than the lifetime cost of bitter melon. On the other hand, the lowest value of bitter melon’s effect with any substantial probability is -5, which if it happened to be true, would be worth quite a bit: $10,449 ().
What is our loss function over the bitter-melon effect-size posterior distribution? In the scenario where we take bitter melon: If the effect is <0 or negative, and it reduces blood-sugar, then the loss is -2270 times the effect-size plus the cost of lifetime bitter melon ($901 from before). If bitter-melon actually increases blood glucose (>0), then likewise—the increased blood glucose does harm to our health and we still pay for bitter melon extracts.
On the other hand, if we don’t take bitter melon, then our loss is 0 since we don’t change our blood glucose and we don’t pay anything more for bitter melon.
mean(coeff[[1]]*2270 + 901)
# [1] 9925.345678
In this case, since the posterior estimate for bitter melon is skewed so heavily towards increasing blood-glucose, the expected loss is very dismal even beyond the cost of buying bitter melon; and since 0 is less than $9925, based on the results of this self-experiment, we would prefer to not use bitter melon in the future.
Of course, we have other options,like collecting more information. Is it worthwhile to experiment more on bitter melon?
EVPI/EVSI
The upper bound on the value of more information is the Expected Value of Perfect Information (EVPI): our expected gain if an oracle told us the exact effect of bitter melon; but remember, we gain only if we switch actions based on new information, otherwise the information was just trivia.
If the oracle tells us bitter melon increases blood glucose by +1 or something and so we shouldn’t take it, this is worthless to us since we had already decided to not take it; if it told us that it reduced by -0.4ng/ml, that exactly counterbalances the total cost of $901, so we still wouldn’t change our action; while if the oracle tells us that bitter melon decreases blood glucose by -2, then we have learned something valuable since a reduction of -2 ng/ml is worth $4.5k to us and the intervention only costs $901, for a big win of $3.6k, or even more if it was actually as much as -6. But we know it’s very unlikely a posteriori that any decrease would be as extreme as -6, and stillrather unlikely that it’s as much as -2, so we need to discount these benefit estimates like $3.6k by how probable they are in the first place. And then we want to average over all of them. This is easily done with the posterior: for each sample of the possible effect (which was the parameter b2
), we ignore it if it’s above -0.4 and note that the information was worth $0, while estimating the gain if it is, and then take the average.
mean(sapply(coeff[[1]], function(bg) { if(bg>=(-0.4)) { return(0); } else { return(-bg*2270 - 901); }}))
# [1] 132.6411989
Because we still haven’t totally ruled out that bitter melon reduces blood glucose, which would be extremely valuable if it did, we would be willing to pay up to $132 for certainty, since we might learn that it does reduce blood glucose by a useful amount. $132 also implies that we might want to do more experimenting, since another bottle of bitter melon would not cost much and could probably drive down that remaining 7%, but is also close to zero, so we might not.
We don’t have access to any EVPI oracle, but we can instead try preposterior analysis: simulating future data, re-estimating the optimal decision based on the new posterior, seeing if it changes our decision to not take bitter melon, and estimating how beneficial that change is, then combine the probability & size of benefit to get expected-value and weigh it against the upfront cost of doing more experimenting. This gives us Expected Value of Sample Information (EVSI). Ideally, EVSI is positive at the start of a trial, and as information comes in, our posterior estimates firm up, our decisions become less likely to change, and the value of additional information decreases until EVSI becomes negative; at which point we can then stop collecting data because the cost of collecting it no longer is less than the reduction from bad decisions it might yield.
Let’s say each datapoint costs $2 (and since we’re measuring 3 times a day, each day costs $6) between the bitter melon & hassle. And we already defined all the other data, model, and losses, so we can calculate EVSI.
Calculating EVSI for collecting one more datapoint is easy but it’s also interesting to calculate it historically and get an idea of how EVSI increased or decreased over the trial
data <- melon2
sampleValues <- data.frame(N=NULL, newOptimumLoss=NULL, sampleValue=NULL, sampleValueProfit=NULL)
for (n in seq(from=1, to=(nrow(data)+10))) {
evsis <- replicate(20, {
# if n is more than we collected, bootstrap hypothetical new data; otherwise, just take that prefix
# and pretend we are doing a sequential trial where we have only collected the first n observations thus far
if (n > nrow(data)) { newData <- rbind(data, data[sample(1:nrow(data), n - nrow(data) , replace=TRUE),]) } else { newData <- data[1:n,] }
kEVSI <- with(newData, run.jags(model1, data=list(n=nrow(newData), Blood=Read, Bitter.Melon=Bitter.Melon,
Date=as.integer(Date), m=length(levels(Date)), Exercise=Exercise,
Morning=as.integer(Measurement=="wake"),
am10=as.integer(Measurement=="10am"), pm3=as.integer(Measurement=="3pm")),
monitor=c("b2"), sample=1000, silent.jags=TRUE, summarise=FALSE))
coeff <- as.mcmc.list(kEVSI, vars="b2")
lossNonuse <- 0
lossUse <- mean(sapply(coeff[[1]], function(bg) { return(-bg*2270 + 901); }))
# compare to the previous estimated optimum using n-1 data
if (n==1) { oldOptimum <- 0; } else { oldOptimum <- sampleValues[n-1,]$newOptimumLoss; }
newOptimum <- max(c(lossNonuse, lossUse))
sampleValue <- newOptimum - oldOptimum
sampleCost <- 2
sampleValueProfit <- sampleValue - (n*sampleCost)
return(list(N=n, newOptimumLoss=newOptimum, sampleValue=sampleValue, sampleValueProfit=sampleValueProfit))
}
)
sampleValues <- rbind(sampleValues, data.frame(N=n, newOptimumLoss=mean(unlist(evsis[2,])),
sampleValue=mean(unlist(evsis[3,])), sampleValueProfit=mean(unlist(evsis[4,]))))
}
sampleValues
# N newOptimumLoss sampleValue sampleValueProfit
# 1 1 0.000000000 0.000000000 -2.00000000
# 2 2 0.000000000 0.000000000 -4.00000000
# 3 3 0.000000000 0.000000000 -6.00000000
# 4 4 0.000000000 0.000000000 -8.00000000
# 5 5 0.000000000 0.000000000 -10.00000000
# 6 6 0.000000000 0.000000000 -12.00000000
# 7 7 0.000000000 0.000000000 -14.00000000
# 8 8 0.000000000 0.000000000 -16.00000000
# 9 9 0.000000000 0.000000000 -18.00000000
# 10 10 766.302423286 766.302423286 746.30242329
# 11 11 414.117599555 -352.184823732 -374.18482373
# 12 12 33.173212243 -380.944387312 -404.94438731
# 13 13 453.109658628 419.936446385 393.93644639
# 14 14 519.121851308 66.012192680 38.01219268
# 15 15 129.521213019 -389.600638289 -419.60063829
# 16 16 1220.999692932 1091.478479912 1059.47847991
# 17 17 2065.063702988 844.064010056 810.06401006
# 18 18 1173.637439342 -891.426263646 -927.42626365
# 19 19 0.000000000 -1173.637439342 -1211.63743934
# 20 20 0.000000000 0.000000000 -40.00000000
# 21 21 0.000000000 0.000000000 -42.00000000
# 22 22 0.000000000 0.000000000 -44.00000000
# 23 23 3.830036441 3.830036441 -42.16996356
# 24 24 0.000000000 -3.830036441 -51.83003644
# 25 25 0.000000000 0.000000000 -50.00000000
# 26 26 0.000000000 0.000000000 -52.00000000
# 27 27 0.000000000 0.000000000 -54.00000000
# ...
# 99 99 0.000000000 0.000000000 -198.00000000
# 100 100 0.000000000 0.000000000 -200.00000000
# 101 101 103.108222757 103.108222757 -98.89177724
# 102 102 0.000000000 -103.108222757 -307.10822276
# 103 103 0.000000000 0.000000000 -206.00000000
# 104 104 0.000000000 0.000000000 -208.00000000
# 105 105 0.000000000 0.000000000 -210.00000000
# 106 106 0.000000000 0.000000000 -212.00000000
# 107 107 0.000000000 0.000000000 -214.00000000
# 108 108 0.000000000 0.000000000 -216.00000000
# 109 109 0.000000000 0.000000000 -218.00000000
# 110 110 0.000000000 0.000000000 -220.00000000
# 111 111 0.000000000 0.000000000 -222.00000000
# 112 112 0.000000000 0.000000000 -224.00000000
# 113 113 0.000000000 0.000000000 -226.00000000
# 114 114 0.000000000 0.000000000 -228.00000000
# 115 115 0.000000000 0.000000000 -230.00000000
So it looks like by the 20th measurement or so (corresponding to day #11, halfway through the first randomization), LaFontaine could have been reasonably certain (assuming non-informative priors etc) that the expected gain from further experimentation with bitter melon did not outweigh the cost of additional experimentation. And taking another 10 samples is likewise expected to be a net loss, with none of the bootstrapped datapoints being able to shift the posterior enough to justify taking bitter melon.
Conclusions
The final outcome suggests that LaFontaine should not take bitter melon and (probably) shouldn’t experiment further with it; the high cost of diabetes, though, indicates he should experiment much more with other anti-diabetes interventions (it’s not clear to me whether drugs such as metformin are a good idea prophylactically, but there’s many potential interventions like exercise kind).
There are many caveats to this conclusion:
-
I used a noninformative prior on the effects of bitter melon, which implies that it’s as likely for bitter melon to drive blood glucose increases as decreases; this strikes me as implausible, and if I had tried to meta-analyze the past studies on bitter melon, I would probably have come up with a much stronger prior in favor of bitter melon, in which case the EVSI of sampling would take much longer to go negative and might have reversed the recommendations
-
on the other hand, the existing bitter melon papers also suggest that taking it in tablet form may be ineffective and only the fresh or juice forms work; in which case, the failure to show benefits was a foregone conclusion and LaFontaine shouldn’t’ve bothered with testing something already believed not to work
-
-
the temporal trend in the blood glucose is concerning because it is too steep to represent any kind of long-term trend (LaFontaine would be dead by now if his blood glucose really did increase by 0.36ng/ml a day) but suggests something wacky was going on during his self-experiment (could the test strips have expired and been going bad such that the results are near-meaningless?); this wackiness is a joker in the deck, since whatever is causing it, could itself be neutralizing any benefit from bitter melon or it could diverge from a linear trend in a way that increases the underlying sampling error beyond what is modeled
-
the diabetes cost estimate is too low; I included only the direct medical costs, though the cost of lost QALYs is probably even larger and the estimate not half what it should be. This underestimation would bias benefit estimates downwards and lead to premature ending of experimenting.
-
in the other direction, the conversion from blood glucose reductions to diabetes risk is questionable; some of the anti-diabetes drugs like metformin are already believed to have effects not mediated solely through blood glucose. This overestimation of the effect of blood glucose reductions would bias estimates upwards and lead to too much experimenting.
I suspect problem #3 & #4 mostly cancel out, that problem #1 would have been a problem if LaFontaine had actually conducted a sequential trial based on EVSI but since he over-collected data it doesn’t wind up being an issue (a favorable prior probably would have been canceled out quickly), and #2 is the major reason that the results could be wrong.
Still, an interesting self-experiment to try to analyze.
See Also
-
While bitter melon is traditionally eaten, and many sweet fruits are not dangerous because they are evolved by the plant to be eaten by animals for various reasons, traditional use is far from a guarantee of safety (consider the widespread low-grade cyanide poisoning from one of the most common crops, cassava, whose danger apparently does not outweigh the caloric value) and bitterness in seeds or fruits is a warning sign of potential low-grade toxicity from the wide world of alkaloids (which is likely why humans have bitterness taste receptors and enjoying a bitter food must be learned); apple seeds are bitter and contain cyanide, wild almonds likewise (‘sweet’ or domesticated almonds have been bred for lack of cyanide), nicotine & caffeine & theobromine are safe for humans only because we are slightly different from insects & dogs, and the fruits Starfruit, ackee fruit, beach apples, and pangium edule are all dangerous to humans. Plants ‘want’ their fruit to be eaten but only by the specific pollinators and seed-spreaders they are co-evolved with… Hence, any fruit whose name literally contains ‘bitter’ is suspicious.↩︎
-
Specifically:
…There was no statistical evidence of an interaction between the rosiglitazone and ramipril arms of the DREAM study for the primary outcomes, secondary outcomes, or their components (interaction p>0·11 for all; data not shown). The primary outcome of diabetes or death was seen in [statistically-]significantly fewer individuals in the rosiglitazone group than in the placebo group (hazard ratio [HR] 0·40, 95% CI 0·35–0·46; p < 0·0001; table 2). There was no difference in the number of deaths (0·91, 0·55–1·49; p = 0·7) and a large difference in the frequency of diabetes (0·38, 0·33–0·44; p < 0·0001) between the two groups (table 2). The event curves for the primary outcome diverged by the time of the first assessment (after 1 year of follow-up; figure 2).
…Figure 5 shows the effect of rosiglitazone on fasting and 2-h plasma glucose concentrations. The median fasting plasma glucose concentration was 0·5 mmol/L lower in the rosiglitazone group than in the placebo group (p < 0·0001); the 2-h plasma glucose concentration was 1·6 mmol/L lower (p < 0·0001). Mean systolic and diastolic blood pressure were 1·7 mm Hg and 1·4 mm Hg lower, respectively, in the rosiglitazone group than in the placebo group (p < 0·0001). Furthermore, mean hepatic ALT concentrations during the first year of therapy were 4·2 U/L lower in patients treated with rosiglitazone than those in the placebo group (p < 0·0001). All results are for the final visit apart from the ALT difference, which was at 1 year. Of note, there was no difference in the use of antihypertensive agents in the two groups during the trial. Finally, by the final visit mean bodyweight was increased by 2·2 kg more in the rosiglitazone group than in the placebo group (p < 0·0001). This increase in bodyweight in the rosiglitazone group was associated with a lower waist-to-hip ratio (p < 0·0001) because of an increase in hip circumference of 1·8 cm; there was no effect on waist circumference (figure 6).
…This large, prospective, blinded international clinical trial shows that 8 mg of rosiglitazone daily, together with lifestyle recommendations, substantially reduces the risk of diabetes or death by 60% in individuals at high risk for diabetes. The absolute risk difference between treatment groups of 14·4% means that for every seven people with impaired fasting glucose or impaired glucose tolerance who are prescribed rosiglitazone for 3 years, one will be prevented from developing diabetes. Moreover, rosiglitazone [statistically-]significantly increased the likelihood of regression to normoglycaemia by about 70–80% compared with placebo. The reduction in diabetes reported here is of much the same magnitude as the reduction achieved with lifestyle approaches4,5 and greater than the reductions reported previously with drugs such as metformin4 or acarbose.3