A tutorial explaining how to train and generate high-quality anime faces with StyleGAN 1+2 neural networks, and tips/scripts for effective StyleGAN use.
- Examples
- Background
- FAQ
- Training Requirements
- Data Preparation
- Training
- Sampling
- Models
- Transfer Learning
- Reversing StyleGAN To Control & Modify Images
- StyleGAN 2
- Future Work
- BigGAN
- See Also
- External Links
- Appendix
Generative neural networks, such as GANs, have struggled for years to generate decent-quality anime faces, despite their great success with photographic imagery such as real human faces. The task has now been effectively solved, for anime faces as well as many other domains, by the development of a new generative adversarial network, StyleGAN, whose source code was released in February 2019.
I show off my StyleGAN 1+2 CC-0-licensed anime faces & videos, provide downloads for the final models & anime portrait face dataset, provide the ‘missing manual’ & explain how I trained them based on Danbooru2017/2018 with source code for the data preprocessing, document installation & configuration & training tricks.
For application, I document various scripts for generating images & videos, briefly describe the website “This Waifu Does Not Exist” I set up as a public demo & its followup This Anime Does Not Exist.ai (TADNE) (see also Artbreeder), discuss how the trained models can be used for transfer learning such as generating high-quality faces of anime characters with small datasets (eg. Holo or Asuka Souryuu Langley), and touch on more advanced StyleGAN applications like encoders & controllable generation.
The anime face graveyard gives samples of my failures with earlier GANs for anime face generation, and I provide samples & model from a relatively large-scale BigGAN training run suggesting that BigGAN may be the next step forward to generating full-scale anime images.
A minute of reading could save an hour of debugging!
StyleGAN2 Is Obsolete
As of 2022, StyleGAN2 is obsolete for anime generation. Alternatives include (newest-first): Pony Diffusion v6 XL, niji · journey, Holara, Waifu-Diffusion, NovelAI, Waifu Labs, or Crypko.
When Ian Goodfellow’s first GAN paper came out in 2014, with its blurry 64px grayscale faces, I said to myself, “given the rate at which GPUs & NN architectures improve, in a few years, we’ll probably be able to throw a few GPUs at some anime collection like Danbooru and the results will be hilarious.” There is something intrinsically amusing about trying to make computers draw anime, and it would be much more fun than working with yet more celebrity headshots or ImageNet samples; further, anime/illustrations/drawings are so different from the exclusively-photographic datasets always (over)used in contemporary ML research that I was curious how it would work on anime—better, worse, faster, or different failure modes? Even more amusing—if random images become doable, then text → images would not be far behind.
So when GANs hit 128px color images on ImageNet, and could do somewhat passable CelebA face samples around 2015, along with my char-RNN experiments, I began experimenting with Soumith Chintala’s implementation of DCGAN, restricting myself to faces of single anime characters where I could easily scrape up ~5–10k faces. (I did a lot of Asuka Souryuu Langley from Neon Genesis Evangelion because she has a color-centric design which made it easy to tell if a GAN run was making any progress: blonde-red hair, blue eyes, and red hair ornaments.)
It did not work. Despite many runs on my laptop & a borrowed desktop, DCGAN never got remotely near to the level of the CelebA face samples, typically topping out at reddish blobs before diverging or outright crashing.1 Thinking perhaps the problem was too-small datasets & I needed to train on all the faces, I began creating the Danbooru2017 version of “Danbooru2018: A Large-Scale Crowdsourced & Tagged Anime Illustration Dataset”. Armed with a large dataset, I subsequently began working through particularly promising members of the GAN zoo, emphasizing SOTA & open implementations.
Among others, I have tried StackGAN/StackGAN++ & Pixel*NN* (failed to get running)2, WGAN-GP, Glow, GAN-QP, MSG-GAN, SAGAN, IntroVAE VGAN, PokeGAN, BigGAN3, ProGAN, & StyleGAN. These architectures vary widely in their design & core algorithms and which of the many stabilization tricks (2019) they use, but they were more similar in their results: dismal.
Glow & BigGAN had promising results reported on CelebA & ImageNet respectively, but unfortunately their training requirements were out of the question.4 (As interesting as SPIRAL and CAN are, no source was released and I couldn’t even attempt them.)
While some remarkable tools like PaintsTransfer/style2paints were created, and there were the occasional semi-successful anime face GANs like IllustrationGAN, the most notable attempt at anime face generation was Make Girls.moe (et al 2017 ). MGM could, interestingly, do in-browser 256px anime face generation using tiny GANs, but that is a dead end. MGM accomplished that much by making the problem easier: they added some light supervision in the form of a crude tag embedding5, and then simplifying the problem drastically to n = 42k faces cropped from professional video game character artwork, which I regarded as not an acceptable solution—the faces were small & boring, and it was unclear if this data-cleaning approach could scale to anime faces in general, much less anime images in general. They are recognizably anime faces but the resolution is low and the quality is not great:
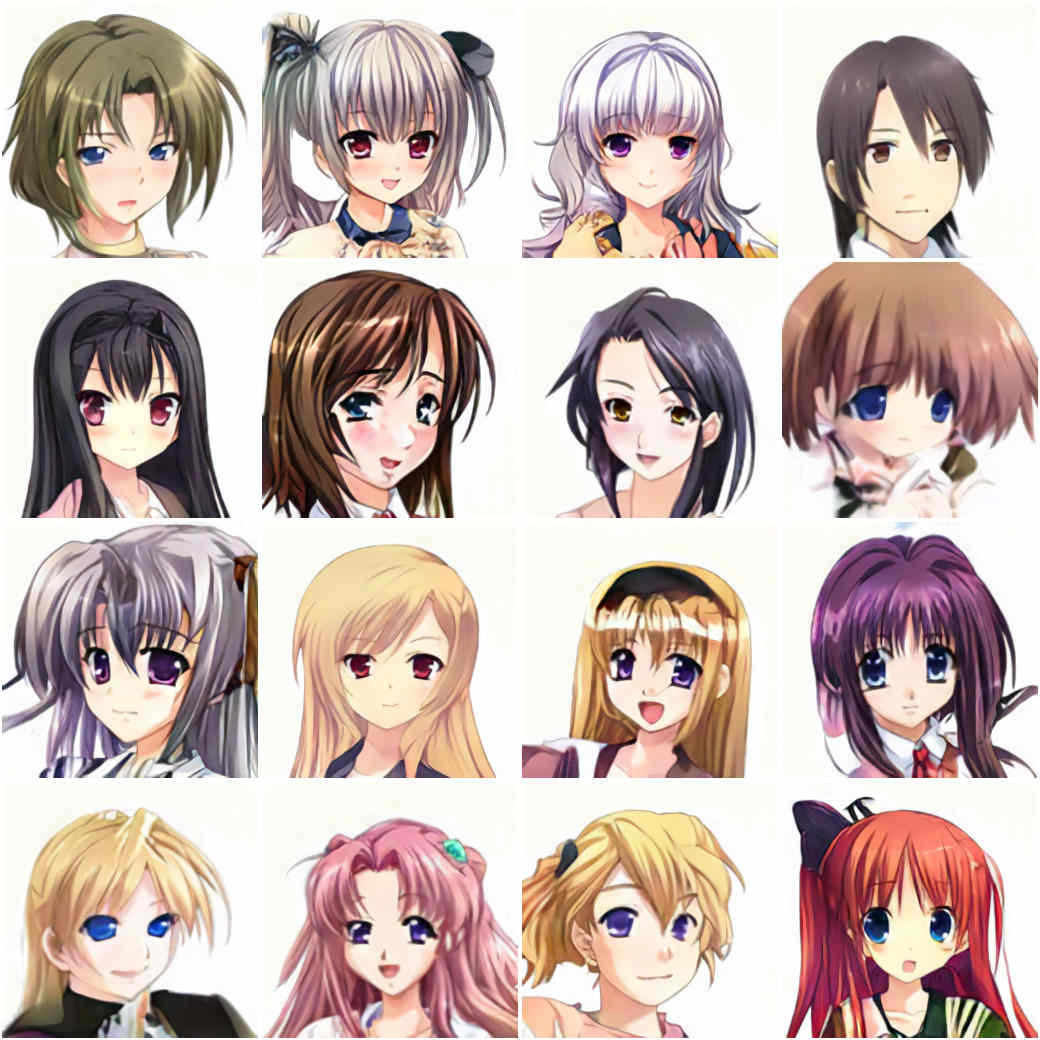
Typically, a GAN would diverge after a day or two of training, or it would collapse to producing a limited range of faces (or a single face), or if it was stable, simply converge to a low level of quality with a lot of fuzziness; perhaps the most typical failure mode was heterochromia (which is common in anime but not that common)—mismatched eye colors (each color individually plausible), from the Generator apparently being unable to coordinate with itself to pick consistently. With more recent architectures like VGAN or SAGAN, which carefully weaken the Discriminator or which add extremely-powerful components like self-attention layers, I could reach fuzzy 128px faces.
Given the miserable failure of all the prior NNs I had tried, I had begun to seriously wonder if there was something about non-photographs which made them intrinsically unable to be easily modeled by convolutional neural networks (the common ingredient to them all). Did convolutions render it unable to generate sharp lines or flat regions of color? Did regular GANs work only because photographs were made almost entirely of blurry textures?
But BigGAN demonstrated that a large cutting-edge GAN architecture could scale, given enough training, to all of ImageNet at even 512px. And ProGAN demonstrated that regular CNNs could learn to generate sharp clear anime images with only somewhat infeasible amounts of training. ProGAN (source; video), while expensive and requiring >6 GPU-weeks6, did work and was even powerful enough to overfit single-character face datasets; I didn’t have enough GPU time to train on unrestricted face datasets, much less anime images in general, but merely getting this far was exciting. Because, a common sequence in DL/DRL (unlike many areas of AI) is that a problem seems intractable for long periods, until someone modifies a scalable architecture slightly, produces somewhat-credible (not necessarily human or even near-human) results, and then throws a ton of compute/data at it and, since the architecture scales, it rapidly exceeds SOTA and approaches human levels (and potentially exceeds human-level). Now I just needed a faster GAN architecture which I could train a much bigger model with on a much bigger dataset.

StyleGAN was the final breakthrough in providing ProGAN-level capabilities but fast: by switching to a radically different architecture, it minimized the need for the slow progressive growing (perhaps eliminating it entirely7), and learned efficiently at multiple levels of resolution, with bonuses in providing much more control of the generated images with its “style transfer” metaphor.
Examples
First, some demonstrations of what is possible with StyleGAN on anime faces:
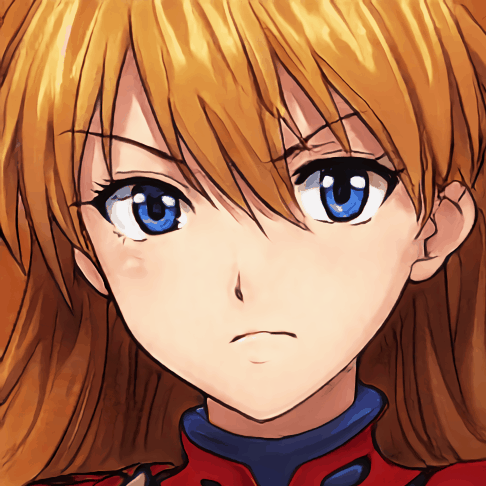
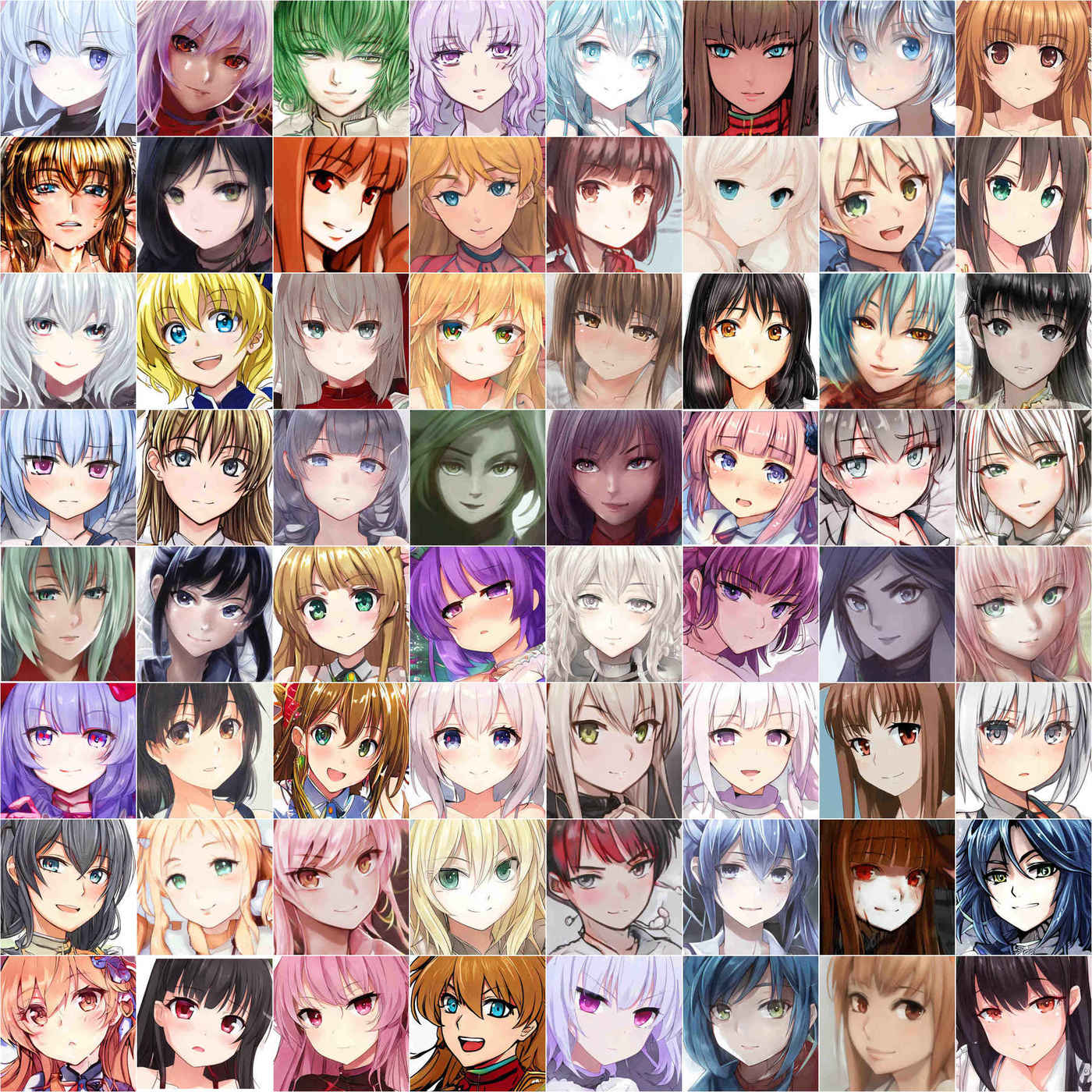
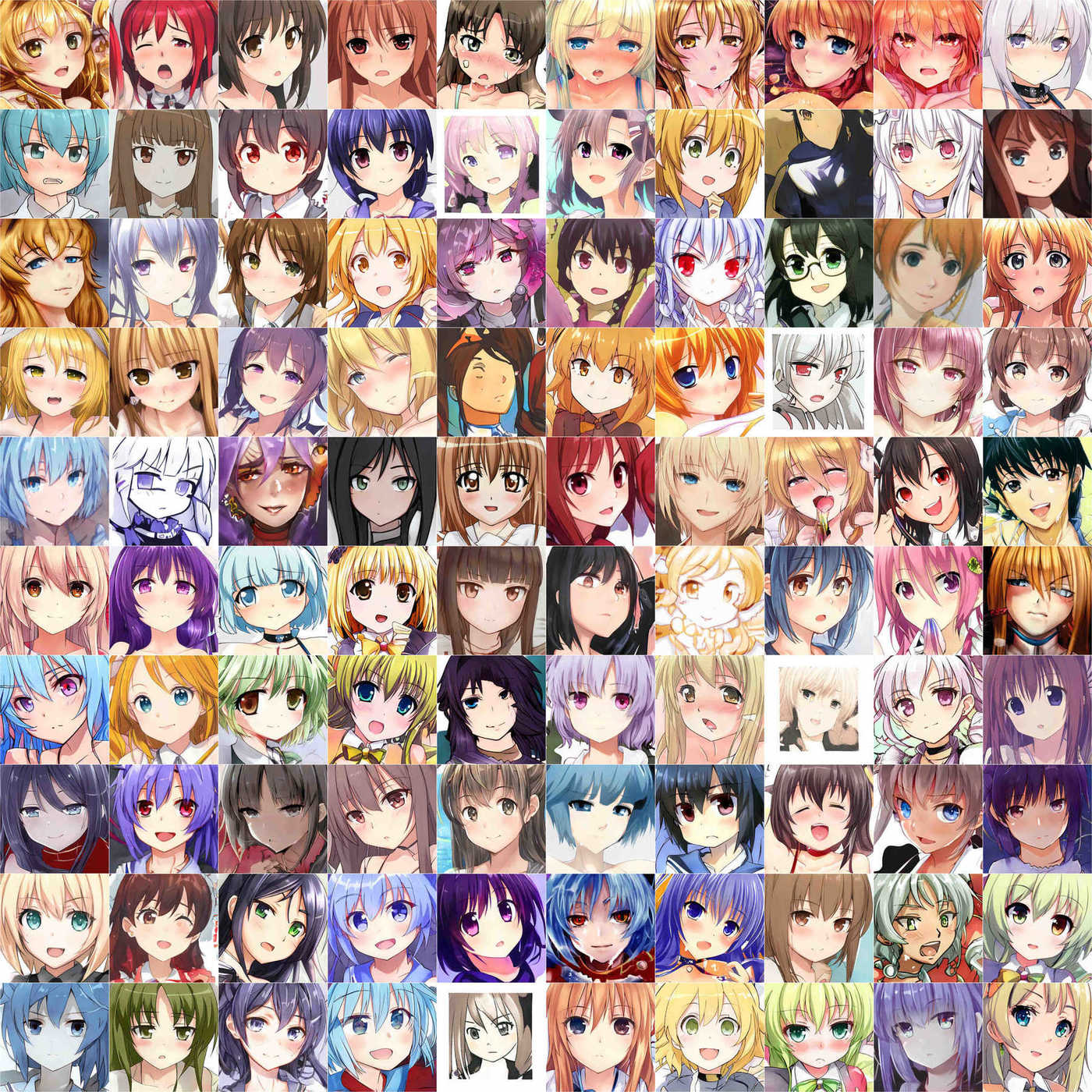
Even a quick look at the MGM & StyleGAN samples demonstrates the latter to be superior in resolution, fine details, and overall appearance (although the MGM faces admittedly have fewer global mistakes). It is also superior to my 2018 ProGAN faces. Perhaps the most striking fact about these faces, which should be emphasized for those fortunate enough not to have spent as much time looking at awful GAN samples as I have, is not that the individual faces are good, but rather that the faces are so diverse, particularly when I look through face samples with Ψ ≥ 1—it is not just the hair/eye color or head orientation or fine details that differ, but the overall style ranges from CG to cartoon sketch, and even the ‘media’ differ, I could swear many of these are trying to imitate watercolors, charcoal sketching, or oil painting rather than digital drawings, and some come off as recognizably ’90s-anime-style vs ’00s-anime-style. (I could look through samples all day despite the global errors because so many are interesting, which is not something I could say of the MGM model whose novelty is quickly exhausted, and it appears that users of my TWDNE website feel similarly as the average length of each visit is 1m:55s.)
Interpolation video of the 2019-02-11 face StyleGAN demonstrating generalization.
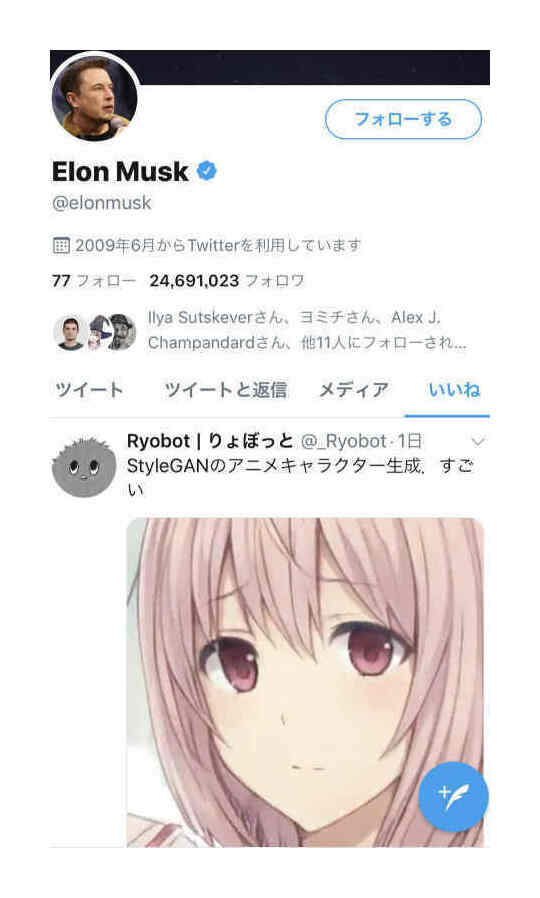
Later interpolation video (2019-03-08 face StyleGAN)
Background
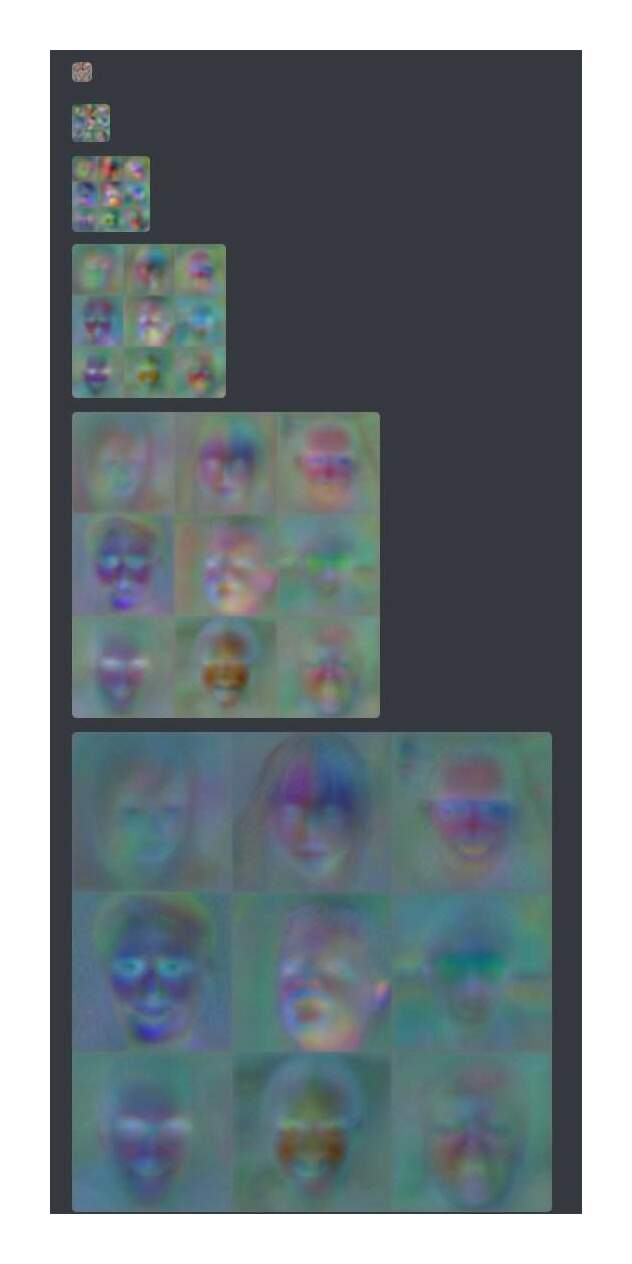
StyleGAN was published in 2018 as “A Style-Based Generator Architecture for Generative Adversarial Networks”, et al 2018 (source code; demo video/algorithmic review video/results & discussions video; Colab notebook9; GenForce PyTorch reimplementation with model zoo/Keras; explainers: Skymind.ai/Lyrn.ai/Two Minute Papers video). StyleGAN takes the standard GAN architecture embodied by ProGAN (whose source code it reuses) and, like the similar GAN architecture et al 2018 , draws inspiration from the field of “style transfer” (essentially invented by et al 2014 ), by changing the Generator (G) which creates the image by repeatedly upscaling its resolution to take, at each level of resolution from 8px→16px→32px→64px→128px etc a random input or “style noise”, which is combined with AdaIN and is used to tell the Generator how to ‘style’ the image at that resolution by changing the hair or changing the skin texture and so on. ‘Style noise’ at a low resolution like 32px affects the image relatively globally, perhaps determining the hair length or color, while style noise at a higher level like 256px might affect how frizzy individual strands of hair are. In contrast, ProGAN and almost all other GANs inject noise into the G as well, but only at the beginning, which appears to work not nearly as well (perhaps because it is difficult to propagate that randomness ‘upwards’ along with the upscaled image itself to the later layers to enable them to make consistent choices?). To put it simply, by systematically providing a bit of randomness at each step in the process of generating the image, StyleGAN can ‘choose’ variations effectively.
![Karras et al 2018, StyleGAN vs ProGAN architecture: “Figure 1. While a traditional generator [29] feeds the latent code [z] though the input layer only, we first map the input to an intermediate latent space W, which then controls the generator through adaptive instance normalization (AdaIN) at each convolution layer. Gaussian noise is added after each convolution, before evaluating the nonlinearity. Here”A” stands for a learned affine transform, and “B” applies learned per-channel scaling factors to the noise input. The mapping network f consists of 8 layers and the synthesis network g consists of 18 layers—two for each resolution (42-−10242). The output of the last layer is converted to RGB using a separate 1×1 convolution, similar to Karras et al. [29]. Our generator has a total of 26.2M trainable parameters, compared to 23.1M in the traditional generator.”](/doc/ai/nn/gan/stylegan/2018-karras-stylegan-figure1-styleganarchitecture.png)
StyleGAN makes a number of additional improvements, but they appear to be less important: for example, it introduces a new “FFHQ” face/portrait dataset with 1024px images in order to show that StyleGAN convincingly improves on ProGAN in final image quality; switches to a loss which is more well-behaved than the usual logistic-style losses; and architecture-wise, it makes unusually heavy use of fully-connected (FC) layers to process an initial random input, no less than 8 layers of 512 neurons, where most GANs use 1 or 2 FC layers.10 More striking is that it omits techniques that other GANs have found critical for being able to train at 512px–1024px scale: it does not use newer losses like the relativistic loss, SAGAN-style self-attention layers in either G/D, VGAN-style variational Discriminator bottlenecks, conditioning on a tag or category embedding11, BigGAN-style large minibatches, different noise distributions12, advanced regularization like spectral normalization, etc.13 One possible reason for StyleGAN’s success is the way it combines outputs from the multiple layers into a single final image rather than repeatedly upscaling; when we visualize the output of each layer as an RGB image in anime StyleGANs, there is a striking division of labor between layers—some layers focus on monochrome outlines, while others fill in textured regions of color, and they sum up into an image with sharp lines and good color gradients while maintaining details like eyes.
Aside from the FCs and style noise & normalization, it is a vanilla architecture. (One oddity is the use of only 3×3 convolutions & so few layers in each upscaling block; a more conventional upscaling block than StyleGAN’s 3×3→3×3 would be something like BigGAN which does 1×1 → 3×3 → 3×3 → 1×1. It’s not clear if this is a good idea as it limits the spatial influence of each pixel by providing limited receptive fields14.) Thus, if one has some familiarity with training a ProGAN or another GAN, one can immediately work with StyleGAN with no trouble: the training dynamics are similar and the hyperparameters have their usual meaning, and the codebase is much the same as the original ProGAN (with the main exception being that config.py
has been renamed train.py
(or run_training.py
in S2) and the original train.py
, which stores the critical configuration parameters, has been moved to training/training_loop.py
; there is still no support for command-line options and StyleGAN must be controlled by editing train.py
/training_loop.py
by hand).
Applications
Because of its speed and stability, when the source code was released on 2019-02-04 (a date that will long be noted in the ANNals of GANime), the Nvidia models & sample dumps were quickly perused & new StyleGANs trained on a wide variety of image types, yielding, in addition to the original faces/carts/cats of et al 2018:
-
“This Person Does Not Exist” (random samples from the 1024px FFHQ face StyleGAN)
-
quizzes: “Which Face is Real”/“Real or Fake?”
-
voting: “Judge Fake People”
-
-
cats: “These Cats Do Not Exist”, “This Cat Does Not Exist” (cat failure modes; interpolation/style-transfer)/corgies
-
hotel rooms (with char-RNN-generated text descriptions): “This Rental Does Not Exist”
-
kitchen/dining room/living room/bedroom (using transfer learning)
-
-
satellite imagery; Gothic cathedrals; Frank Gehry buildings; cityscapes; floor plans
-
“This Waifu Does Not Exist” (background/implementation)
-
large upgrade over TWDNE: random generation, exploration, image attribute editing, saving to a gallery, and crossbreeding portraits
-
interactive waifu generator (improved results, inspired by TWDNE & using Danbooru2018 as a dataset)
-
-
fonts: 1/2; Unicode characters; kanji
-
eyes (ProGAN)
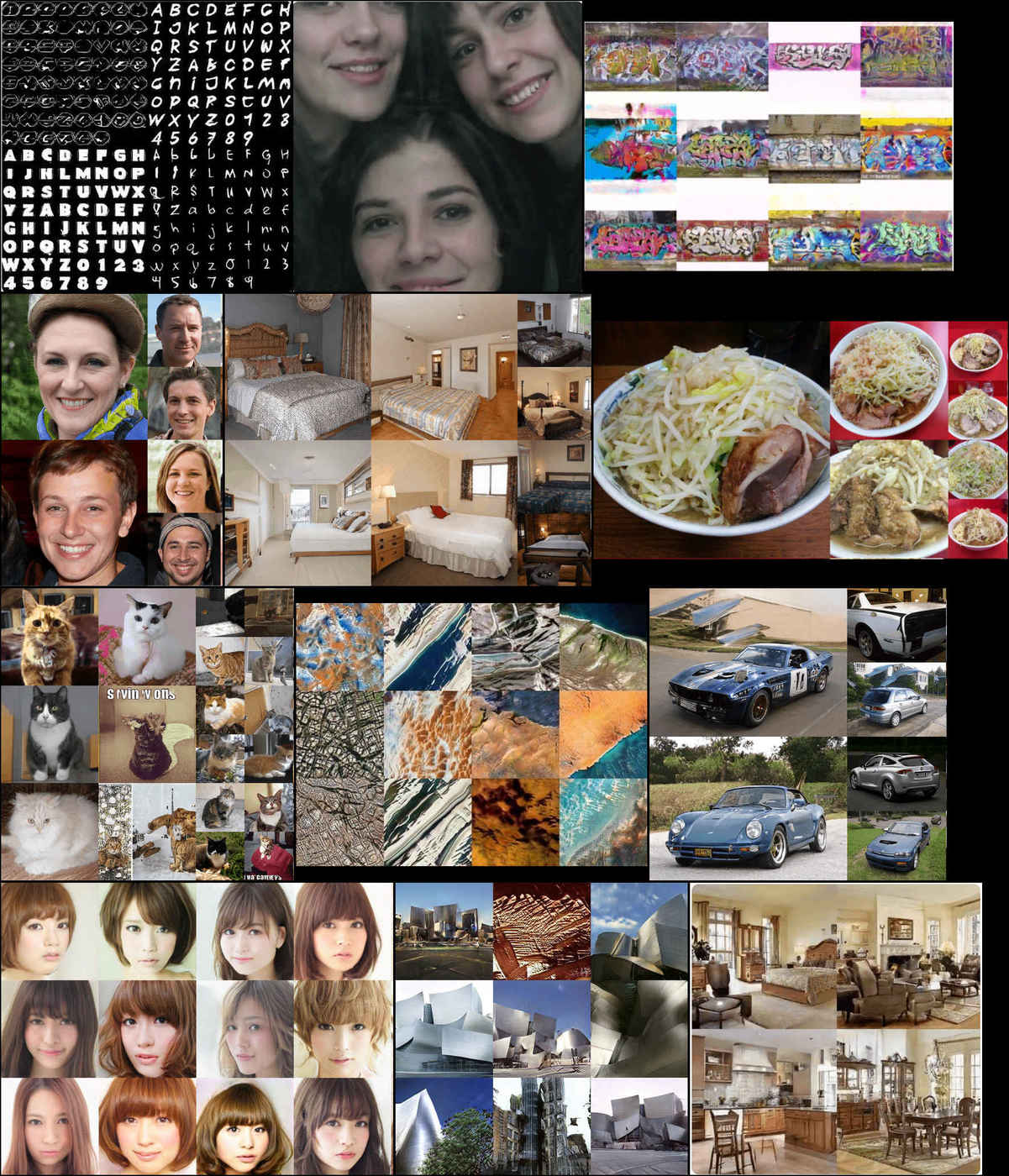
Why Don’t GANs Work?
Why does StyleGAN work so well on anime images while other GANs worked not at all or slowly at best?
The lesson I took from “Are GANs Created Equal? A Large-Scale Study”, et al 2017, is that CelebA/ CIFAR-10 are too easy, as almost all evaluated GAN architectures were capable of occasionally achieving good FID if one simply did enough iterations & hyperparameter tuning.
Interestingly, I consistently observe in training all GANs on anime that clear lines & sharpness & cel-like smooth gradients appear only toward the end of training, after typically initially blurry textures have coalesced. This suggests an inherent bias of CNNs: color images work because they provide some degree of textures to start with, but lineart/monochrome stuff fails because the GAN optimization dynamics flail around. This is consistent with et al 2018’s findings —which uses style transfer to construct a data-augmented/transformed “Stylized-ImageNet”—showing that ImageNet CNNs are lazy and, because the tasks can be achieved to some degree with texture-only classification (as demonstrated by several of et al 2018’s authors via “BagNets”), focus on textures unless otherwise forced; and by ResNeXt & 2019, who find that although CNNs are perfectly capable of emphasizing shape over texture, lower-performing models tend to rely more heavily on texture and that many kinds of training (including BigBiGAN) will induce a texture focus, suggesting texture tends to be lower-hanging fruit. So while CNNs can learn sharp lines & shapes rather than textures, the typical GAN architecture & training algorithm do not make it easy. Since CIFAR-10/CelebA can be fairly described as being just as heavy on textures as ImageNet (which is not true of anime images), it is not surprising that GANs train easily on them starting with textures and gradually refining into good samples but then struggle on anime.
This raises a question of whether the StyleGAN architecture is necessary and whether many GANs might work, if only one had good style transfer for anime images and could, to defeat the texture bias, generate many versions of each anime image which kept the shape while changing the color palette? (Current style transfer methods like the AdaIN PyTorch implementation used by et al 2018, do not work well on anime images, ironically enough, because they are trained on photographic images, typically using the old VGG model.)
FAQ
…Its social accountability seems sort of like that of designers of military weapons: unculpable right up until they get a little too good at their job.
David Foster Wallace, “E unibus pluram: Television and U.S. Fiction”
To address some common questions people have after seeing generated samples:
-
Overfitting: “Aren’t StyleGAN (or BigGAN) just overfitting & memorizing data?”
Amusingly, this is not a question anyone really bothered to ask of earlier GAN architectures, which is a sign of progress. Overfitting is a better problem to have than underfitting, because overfitting means you can use a smaller model or more data or more aggressive regularization techniques, while underfitting means your approach just isn’t working.
In any case, while there is currently no way to conclusively prove that cutting-edge GANs are not 100% memorizing (because they should be memorizing to a considerable extent in order to learn image generation, and evaluating generative models is hard in general, and for GANs in particular, because they don’t provide standard metrics like likelihoods which could be used on held-out samples), there are several reasons to think that they are not just memorizing:16
-
Sample/Dataset Overlap: a standard check for overfitting is to compare generated images to their closest matches using nearest-neighbors (where distance is defined by features like a CNN embedding) lookup; an example of this are StackGAN’s Figure 6 & BigGAN’s Figures 10–14 & 2021, where the photorealistic samples are nevertheless completely different from the most similar ImageNet datapoints. (It’s worth noting that Clearview AI’s facial recognition reportedly does not return Flickr matches for random FFHQ StyleGAN faces, suggesting the generated faces genuinely look like new faces rather than any of the original Flickr faces.)
One intriguing observation about GANs made by the BigGAN paper is that the criticisms of Generators memorizing datapoints may be precisely the opposite of reality: GANs may work primarily by the Discriminator (adaptively) overfitting to datapoints, thereby repelling the Generator away from real datapoints and forcing it to learn nearby possible images which collectively span the image distribution. (With enough data, this creates generalization because “neural nets are lazy” and only learn to generalize when easier strategies fail.)
-
Semantic Understanding: GANs appear to learn meaningful concepts like individual objects, as demonstrated by “latent space addition” or research tools like GANdissection/et al 2018 ; regression tasks, depth maps, or image edits like object deletions/additions (et al 2020 ) or segmenting objects like dogs from their backgrounds (2020/et al 2020 , et al 2021 , et al 2021 , et al 2021 , et al 2022 —as do diffusion models) are difficult to explain without some genuine understanding of images.
In the case of StyleGAN anime faces, there are encoders and controllable face generation now which demonstrate that the latent variables do map onto meaningful factors of variation & the model must have genuinely learned about creating images rather than merely memorizing real images or image patches. Similarly, when we use the “truncation trick”/ψ to sample from relatively extreme unlikely images and we look at the distortions, they show how generated images break down in semantically-relevant ways, which would not be the case if it was just plagiarism. (A particularly extreme example of the power of the learned StyleGAN primitives is et al 2019’s demonstration that Karras et al’s FFHQ faces StyleGAN can be used to generate fairly realistic images of cats/dogs/cars.)
-
Latent Space Smoothness: in general, interpolation in the latent space (z) shows smooth changes of images and logical transformations or variations of face features; if StyleGAN were merely memorizing individual datapoints, the interpolation would be expected to be low quality, yield many terrible faces, and exhibit ‘jumps’ in between points corresponding to real, memorized, datapoints. The StyleGAN anime face models do not exhibit this. (In contrast, the Holo ProGAN, which overfit badly, does show severe problems in its latent space interpolation videos.)
Which is not to say that GANs do not have issues: “mode dropping” seems to still be an issue for BigGAN despite the expensive large-minibatch training, which is overfitting to some degree, and StyleGAN presumably suffers from it too.
-
Transfer Learning: GANs have been used for semi-supervised learning (eg. generating plausible ‘labeled’ samples to train a classifier on), imitation learning like GAIL, and retraining on further datasets; if the G is merely memorizing, it is difficult to explain how any of this would work.
-
-
Compute Requirements: “Doesn’t StyleGAN take too long to train?”
StyleGAN is remarkably fast-training for a GAN. With the anime faces, I got better results after 1–3 days of StyleGAN training than I’d gotten with >3 weeks of ProGAN training. The training times quoted by the StyleGAN repo may sound scary, but they are, in practice, a steep overestimate of what you actually need, for several reasons:
-
Lower Resolution: the largest figures are for 1024px images but you may not need them to be that large or even have a big dataset of 1024px images. For anime faces, 1024px-sized faces are relatively rare, and training at 512px & upscaling 2× to 1024 with
waifu2x
17 works fine & is much faster. Since upscaling is relatively simple & easy, another strategy is to change the progressive-growing schedule: instead of proceeding to the final resolution as fast as possible, instead adjust the schedule to stop at a more feasible resolution & spend the bulk of training time there instead and then do just enough training at the final resolution to learn to upscale (eg. spend 10% of training growing to 512px, then 80% of training time at 512px, then 10% at 1024px). -
Diminishing Returns: the largest gains in image quality are seen in the first few days or weeks of training with the remaining training being not that useful as they focus on improving small details (so just a few days may be more than adequate for your purposes, especially if you’re willing to select a little more aggressively from samples)
-
Transfer Learning from a related model can save days or weeks of training, as there is no need to train from scratch; with the anime face StyleGAN, one can train a character-specific StyleGAN with a few hours or days at most, and certainly do not need to spend multiple weeks training from scratch! (assuming that wouldn’t just cause overfitting) Similarly, if one wants to train on some 1024px face dataset, why start from scratch, taking ~1000 GPU-hours, when you can start from Nvidia’s FFHQ face model which is already fully trained, and can converge in a fraction of the from-scratch time? For 1024px, you could use a super-resolution GAN like to upscale? Alternately, you could change the image progression budget to spend most of your time at 512px and then at the tail end try 1024px.
-
One-Time Costs: the upfront cost of a few hundred dollars of GPU-time (at inflated AWS prices) may seem steep, but should be kept in perspective. As with almost all NNs, training 1 StyleGAN model can be literally tens of millions of times more expensive than simply running the Generator to produce 1 image; but it also need be paid only once by only one person, and the total price need not even be paid by the same person, given transfer learning, but can be amortized across various datasets. Indeed, given how fast running the Generator is, the trained model doesn’t even need to be run on a GPU. (The rule of thumb is that a GPU is 20–30× faster than the same thing on CPU, with rare instances when overhead dominates of the CPU being as fast or faster, so since generating 1 image takes on the order of ~0.1s on GPU, a CPU can do it in ~3s, which is adequate for many purposes.)
-
-
Copyright Infringement: “Who owns StyleGAN images?”
-
The Nvidia Source Code & Released Models for StyleGAN 1 are under a CC-BY-NC license, and you cannot edit them or produce “derivative works” such as retraining their FFHQ, cat, or cat StyleGAN models. (StyleGAN 2 is under a new “Nvidia Source Code License-NC”, which appears to be effectively the same as the CC-BY-NC with the addition of a patent retaliation clause.)
If a model is trained from scratch, then that does not apply as the source code is simply another tool used to create the model and nothing about the CC-BY-NC license forces you to donate the copyright to Nvidia. (It would be odd if such a thing did happen—if your word processor claimed to transfer the copyrights of everything written in it to Microsoft!)
For those concerned by the CC-BY-NC license, a 512px FFHQ config-f StyleGAN 2 has been trained & released into the public domain by Aydao, and is available for download from Mega and my rsync mirror:
rsync --verbose rsync://176.9.41.242:873/biggan/2020-06-07-aydao-stylegan2-configf-ffhq-512-avg-tpurun1.pkl.xz ./
-
Models in general are generally considered “transformative works” and the copyright owners of whatever data the model was trained on have no copyright on the model. (The fact that the datasets or inputs are copyrighted is irrelevant, as training on them is universally considered fair use and transformative, similar to artists or search engines; see the further reading.) The model is copyrighted to whomever created it. Hence, Nvidia has copyright on the models it created but I have copyright under the models I trained (which I release under CC-0).
-
Samples are trickier. The usual widely-stated legal interpretation is that the standard copyright law position is that only human authors can earn a copyright and that machines, animals, inanimate objects or most famously, monkeys, cannot. The US Copyright Office states clearly that regardless of whether we regard a GAN as a machine or a something more intelligent like an animal, either way, it doesn’t count:
A work of authorship must possess “some minimal degree of creativity” to sustain a copyright claim. Feist, 499 U.S. at 358, 362 (citation omitted). “[T]he requisite level of creativity is extremely low.” Even a “slight amount” of creative expression will suffice. “The vast majority of works make the grade quite easily, as they possess some creative spark, ‘no matter how crude, humble or obvious it might be.’” Id. at 346 (citation omitted).
… To qualify as a work of “authorship” a work must be created by a human being. See Burrow-Giles Lithographic Co., 111 U.S. at 58. Works that do not satisfy this requirement are not copyrightable. The Office will not register works produced by nature, animals, or plants.
Examples:
-
A photograph taken by a monkey.
-
A mural painted by an elephant.
…the Office will not register works produced by a machine or mere mechanical process that operates randomly or automatically without any creative input or intervention from a human author.
A dump of random samples such as the Nvidia samples or TWDNE therefore has no copyright & by definition is in the public domain.
A new copyright can be created, however, if a human author is sufficiently ‘in the loop’, so to speak, as to exert a de minimis amount of creative effort, even if that ‘creative effort’ is simply selecting a single image out of a dump of thousands of them or twiddling knobs (eg. on Make Girls.Moe). Crypko, for example, take this position.
-
Further reading on computer-generated art copyrights:
-
“Copyrights In Computer-Generated Works: Whom, If Anyone, Do We Reward?”, 2001
-
“Ex Machina: Copyright Protection For Computer-Generated Works”, 2016
-
“Computer Generated Works and Copyright: Selfies, Traps, Robots, AI and Machine Learning”, 2017
-
“Who holds the Copyright in AI Created Art?”, Steve Schlackman (2018)
-
“The Machine as Author”, 2019
-
“Why Is AI Art Copyright So Complicated?”, Jason Bailey (2019)
-
“We’ve been warned about AI and music for over 50 years, but no one’s prepared: ‘This road is literally being paved as we’re walking on it’” (The Verge 2019)
-
Copyright
Per the copyright point above, all my generated videos and samples and models are released under the CC-0 (public domain equivalent) license. Source code listed may be derivative works of Nvidia’s CC-BY-NC-licensed StyleGAN code, and may be CC-BY-NC.
Training Requirements
Data
The road of excess leads to the palace of wisdom
…If the fool would persist in his folly he would become wise
…You never know what is enough unless you know what is more than enough.
…If others had not been foolish, we should be so.William Blake, “Proverbs of Hell”, The Marriage of Heaven and Hell
The necessary size for a dataset depends on the complexity of the domain and whether transfer learning is being used. StyleGAN’s default settings yield a 1024px Generator with 26.2M parameters, which is a large model and can soak up potentially millions of images, so there is no such thing as too much.
For learning decent-quality anime faces from scratch, a minimum of 5000 appears to be necessary in practice; for learning a specific character when using the anime face StyleGAN, potentially as little as ~500 (especially with data augmentation) can give good results. For domains as complicated as “any cat photo” like et al 2018’s cat StyleGAN which is trained on the LSUN CATS category of ~1.8M18 cat photos, that appears to either not be enough or StyleGAN was not trained to convergence; et al 2018 note that “CATS continues to be a difficult dataset due to the high intrinsic variation in poses, zoom levels, and backgrounds.”19
Compute
To fit reasonable minibatch sizes, one will want GPUs with >11GB VRAM. At 512px, that will only train n = 4, and going below that means it’ll be even slower (and you may have to reduce learning rates to avoid unstable training). So, 1080ti & up would be good. (Reportedly, AMD/OpenCL works for running StyleGAN models, and there is one report of successful training with “Radeon VII with tensorflow-rocm
1.13.2 and rocm
2.3.14”.)
The StyleGAN repo provide the following estimated training times for 1–8 GPU systems (which I convert to total GPU-hours & provide a worst-case AWS-based cost estimate):
Estimated StyleGAN wallclock training times for various resolutions & GPU-clusters (source: StyleGAN repo)
GPUs
10242
5122
2562
[March 2019 AWS Costs20]
1
41 days 4 hours [988 GPU-hours]
24 days 21 hours [597 GPU-hours]
14 days 22 hours [358 GPU-hours]
[$320, $194, $115]
2
21 days 22 hours [1,052]
13 days 7 hours [638]
9 days 5 hours [442]
[NA]
4
11 days 8 hours [1,088]
7 days 0 hours [672]
4 days 21 hours [468]
[NA]
8
6 days 14 hours [1,264]
4 days 10 hours [848]
3 days 8 hours [640]
[$2,730, $1,831, $1,382]
AWS GPU instances are some of the most expensive ways to train a NN and provide an upper bound (compare Vast.ai); 512px is often an acceptable (or necessary) resolution; and in practice, the full quoted training time is not really necessary—with my anime face StyleGAN, the faces themselves were high quality within 48 GPU-hours, and what training it for ~1000 additional GPU-hours accomplished was primarily to improve details like the shoulders & backgrounds. (ProGAN/StyleGAN particularly struggle with backgrounds & edges of images because those are cut off, obscured, and highly-varied compared to the faces, whether anime or FFHQ. I hypothesize that the telltale blurry backgrounds are due to the impoverishment of the backgrounds/edges in cropped face photos, and they could be fixed by transfer-learning or pretraining on a more generic dataset like ImageNet, so it learns what the backgrounds even are in the first place; then in face training, it merely has to remember them & defocus a bit to generate correct blurry backgrounds.)
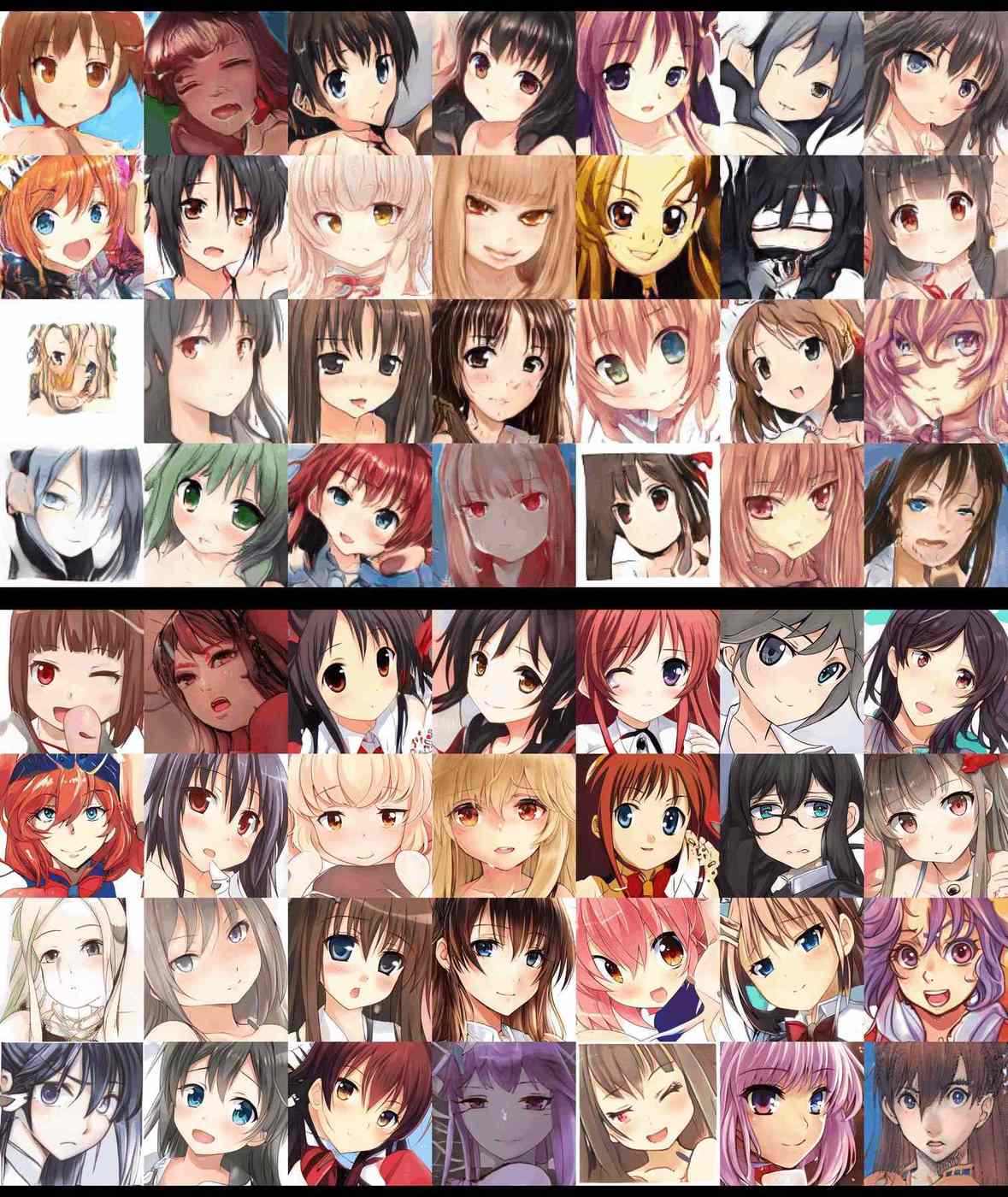
Training on a desktop is a bit painful because GPU use is not controlled by nice
/ionice
priorities and any training will badly degrade the usability/latency of your GUI, which is a frustration that builds up over month-long runtimes. If you have multiple GPUs, you can offload training to the spare GPUs; if you have only 1 GPU, you can try using lazy
: a tool for running processes in idle time—it will cost you some throughput as it pauses training while you are actively using your computer, but it’ll be worth it.
Data Preparation
The most difficult part of running StyleGAN is preparing the dataset properly. StyleGAN does not, unlike most GAN implementations (particularly PyTorch ones), support reading a directory of files as input; it can only read its unique .tfrecord
format which stores each image as raw arrays at every relevant resolution.21 Thus, input files must be perfectly uniform, (slowly) converted to the .tfrecord
format by the special dataset_tool.py
tool, and will take up ~19× more disk space.22
A StyleGAN dataset must consist of images all formatted exactly the same way.
Images must be precisely 512×512px or 1024×1024px etc (any eg. 512×513px images will kill the entire run), they must all be the same colorspace (you cannot have sRGB and Grayscale JPGs—and I doubt other color spaces work at all), they must not be transparent, the filetype must be the same as the model you intend to (re)train (ie. you cannot retrain a PNG-trained model on a JPG dataset, StyleGAN will crash every time with inscrutable convolution/channel-related errors)23, and there must be no subtle errors like CRC checksum errors which image viewers or libraries like ImageMagick often ignore.
Faces Preparation
My workflow:
-
Download raw images from Danbooru2021 if necessary
-
Extract from the JSON Danbooru2018 metadata all the IDs of a subset of images if a specific Danbooru tag (such as a single character) is desired, using
jq
and shell scripting -
Crop square anime faces from raw images using Nagadomi’s
lbpcascade_animeface
(regular face-detection methods do not work on anime images) -
Delete empty files, monochrome or grayscale files, & exact-duplicate files
-
Convert to JPG
-
Upscale below the target resolution (512px) images with
waifu2x
-
Convert all images to exactly 512×512 resolution sRGB JPG images
-
If feasible, improve data quality by checking for low-quality images by hand, removing near-duplicates images found by
findimagedupes
, and filtering with a pretrained GAN’s Discriminator -
Convert to StyleGAN format using
dataset_tool.py
The goal is to turn this:
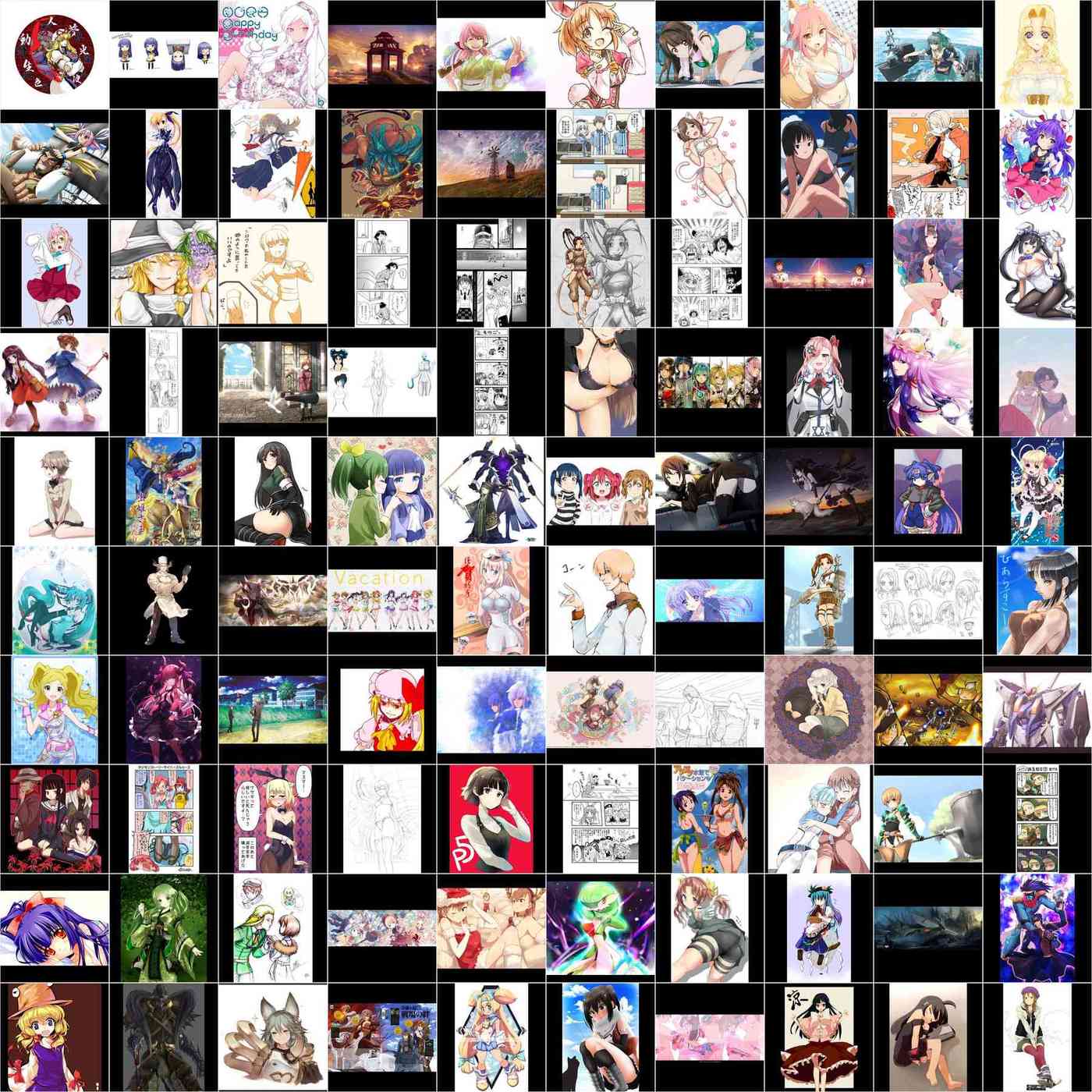
into this:
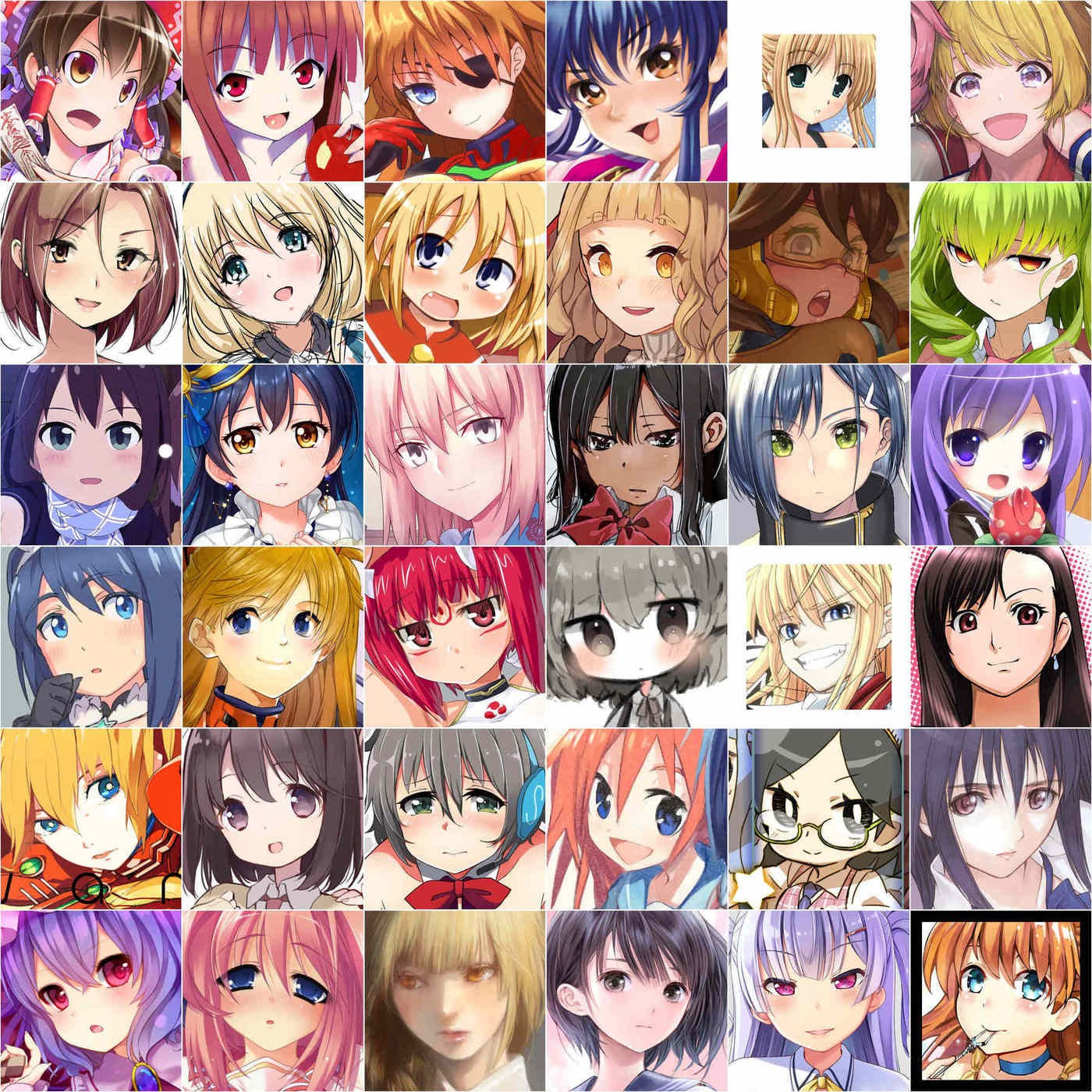
Below I use shell scripting to prepare the dataset. A possible alternative is danbooru-utility
, which aims to help “explore the dataset, filter by tags, rating, and score, detect faces, and resize the images”.
Cropping
The Danbooru download can be done via rsync, which provides a JSON metadata tarball which unpacks into metadata/2*
& a folder structure of {original,512px}/{0-999}/$ID.{png,jpg,...}
.
For training on SFW whole images, the 512px/
version of Danbooru2018 would work, but it is not a great idea for faces because by scaling images down to 512px, a lot of face detail has been lost, and getting high-quality faces is a challenge. The SFW IDs can be extracted from the filenames in 512px/
directly or from the metadata by extracting the id
& rating
fields (and saving to a file):
find ./512px/ -type f | sed -e 's/.*\/\([[:digit:]]*\)\.jpg/\1/'
# 967769
# 1853769
# 2729769
# 704769
# 1799769
# ...
tar xf metadata.json.tar.xz
cat metadata/20180000000000* | jq '[.id, .rating]' -c | grep -F '"s"' | cut -d '"' -f 2 # "
# ...
After installing and testing Nagadomi’s lbpcascade_animeface
to make sure it & OpenCV works, one can use a simple script which crops the face(s) from a single input image. The accuracy on Danbooru images is fairly good, perhaps 90% excellent faces, 5% low-quality faces (genuine but either awful art or tiny little faces on the order of 64px which useless), and 5% outright errors—non-faces like armpits or elbows (oddly enough). It can be improved by making the script more restrictive, such as requiring 250×250px regions, which eliminates most of the low-quality faces & mistakes. (There is an alternative more-difficult-to-run library by Nakatomi which offers a face-cropping script, animeface-2009’s face_collector.rb
, which Nakatomi says is better at cropping faces, but I was not impressed when I tried it out.) crop.py
:
import cv2
import sys
import os.path
def detect(cascade_file, filename, outputname):
if not os.path.isfile(cascade_file):
raise RuntimeError("%s: not found" % cascade_file)
cascade = cv2.CascadeClassifier(cascade_file)
image = cv2.imread(filename)
gray = cv2.cvtColor(image, cv2.COLOR_BGR2GRAY)
gray = cv2.equalizeHist(gray)
## NOTE: Suggested modification: increase minSize to '(250,250)' px,
## increasing proportion of high-quality faces & reducing
## false positives. Faces which are only 50×50px are useless
## and often not faces at all.
## FOr my StyleGANs, I use 250 or 300px boxes
faces = cascade.detectMultiScale(gray,
# detector options
scaleFactor = 1.1,
minNeighbors = 5,
minSize = (50, 50))
i=0
for (x, y, w, h) in faces:
cropped = image[y: y + h, x: x + w]
cv2.imwrite(outputname+str(i)+".png", cropped)
i=i+1
if len(sys.argv) != 4:
sys.stderr.write("usage: detect.py <animeface.xml file> <input> <output prefix>\n")
sys.exit(-1)
detect(sys.argv[1], sys.argv[2], sys.argv[3])
The IDs can be combined with the provided lbpcascade_animeface
script using xargs
, however this will be far too slow and it would be better to exploit parallelism with xargs --max-args=1 --max-procs=16
or parallel
. It’s also worth noting that lbpcascade_animeface
seems to use up GPU VRAM even though GPU use offers no apparent speedup (a slowdown if anything, given limited VRAM), so I find it helps to explicitly disable GPU use by setting CUDA_VISIBLE_DEVICES=""
. (For this step, it’s quite helpful to have a many-core system like a Threadripper.)
Combining everything, parallel face-cropping of an entire Danbooru2018 subset can be done like this:
cropFaces() {
BUCKET=$(printf "%04d" $(( $@ % 1000 )) )
ID="$@"
CUDA_VISIBLE_DEVICES="" nice python ~/src/lbpcascade_animeface/examples/crop.py \
~/src/lbpcascade_animeface/lbpcascade_animeface.xml \
./original/$BUCKET/$ID.* "./faces/$ID"
}
export -f cropFaces
mkdir ./faces/
cat sfw-ids.txt | parallel --progress cropFaces
# NOTE: because of the possibility of multiple crops from an image, the script appends a N counter;
# remove that to get back the original ID & filepath: eg
#
## original/0196/933196.jpg → portrait/9331961.jpg
## original/0669/1712669.png → portrait/17126690.jpg
## original/0997/3093997.jpg → portrait/30939970.jpg
Nvidia StyleGAN, by default and like most image-related tools, expects square images like 512×512px, but there is nothing inherent to neural nets or convolutions that requires square inputs or outputs, and rectangular convolutions are possible. In the case of faces, they tend to be more rectangular than square, and we’d prefer to use a rectangular convolution if possible to focus the image on the relevant dimension rather than either pay the severe performance penalty of increasing total dimensions to 1024×1024px or stick with 512×512px & waste image outputs on emitting black bars/backgrounds. A properly-sized rectangular convolution can offer a nice speedup (eg. Fast.ai’s training ImageNet in 18m for $40 using them among other tricks). Nolan Kent’s StyleGAN re-implemention (released October 2019) does support rectangular convolutions, and as he demonstrates in his blog post, it works nicely.
Cleaning & Upscaling
Miscellaneous cleanups can be done:
## Delete failed/empty files
find faces/ -size 0 -type f -delete
## Delete 'too small' files which is indicative of low quality:
find faces/ -size -40k -type f -delete
## Delete exact duplicates:
fdupes --delete --omitfirst --noprompt faces/
## Delete monochrome or minimally-colored images:
### the heuristic of <257 unique colors is imperfect but better than anything else I tried
deleteBW() { if [[ `identify -format "%k" "$@"` -lt 257 ]];
then rm "$@"; fi; }
export -f deleteBW
find faces -type f | parallel --progress deleteBW
I remove black-white or grayscale images from all my GAN experiments because in my earliest experiments, their inclusion appeared to increase instability: mixed datasets were extremely unstable, monochrome datasets failed to learn at all, but color-only runs made some progress. It is likely that StyleGAN is now powerful enough to be able to learn on mixed datasets (and some later experiments by other people suggest that StyleGAN can handle both monochrome & color anime-style faces without a problem), but I have not risked a full month-long run to investigate, and so I continue doing color-only.
Discriminator Ranking: Using a Trained Discriminator to Rank and Clean Data
The Discriminator of a GAN is trained to detect outliers or bad datapoints. So it can be used for cleaning the original dataset of aberrant samples. This works reasonably well and I obtained BigGAN/StyleGAN quality improvements by manually deleting the worst samples (typically badly-cropped or low-quality faces), but has peculiar behavior which indicates that the Discriminator is not learning anything equivalent to a “quality” score but may be doing some form of memorization of specific real datapoints. What does this mean for how GANs work?
What is a D doing? I find that the highest ranked images often contain many anomalies or low-quality images which need to be deleted. Why? The BigGAN paper notes a well-trained D which achieves 98% real vs fake classification performance on the ImageNet training dataset falls to 50–55% accuracy when run on the validation dataset, suggesting the D’s role is about memorizing the training data rather than some measure of ‘realism’.
A good trick with GANs is, after training to reasonable levels of quality, reusing the Discriminator to rank the real datapoints; images the trained D assigns the lowest probability/score of being real are often the worst-quality ones and going through the bottom decile (or deleting them entirely) should remove many anomalies and may improve the GAN. The GAN is then trained on the new cleaned dataset, making this a kind of “active learning”.
Since rating images is what the D already does, no new algorithms or training methods are necessary, and almost no code is necessary: run the D on the whole dataset to rank each image (faster than it seems since the G & backpropagation are unnecessary, even a large dataset can be ranked in a wallclock hour or two), then one can review manually the bottom & top X%, or perhaps just delete the bottom X% sight unseen if enough data is available.
Perhaps because the D ranking is not necessarily a ‘quality’ score but simply a sort of confidence rating that an image is from the real dataset; if the real images contain certain easily-detectable images which the G can’t replicate, then the D might memorize or learn them quickly. For example, in face crops, whole figure crops are common mistaken crops, making up a tiny percentage of images; how could a face-only G learn to generate whole realistic bodies without the intermediate steps being instantly detected & defeated as errors by D, while D is easily able to detect realistic bodies as definitely real? This would explain the polarized rankings. And given the close connections between GANs & DRL, I have to wonder if there is more memorization going on than suspected in things like “Deep reinforcement learning from human preferences”? Incidentally, this may also explain the problems with using Discriminators for visualization (image generation via gradient ascent) & semi-supervised representation learning: if the D is memorizing datapoints to force the G to generalize, then its internal representations would be expected to be useless—to memorize, it is doing things like learning datapoint-specific details or learning non-robust features, which would lead to visualizations which just look like high-frequency noise to a human viewer or to ungeneralizable ‘embeddings’. (For embeddings, one would instead want to extract knowledge from the G, perhaps by encoding an image into z and using the z as the representation because it has so many disentangled semantics encoded into it.)
An alternative perspective is offered by a crop of 2020 papers (et al 2020b ; et al 2020 ; et al 2020 ; et al 2020c ) examining how useful GAN data augmentation requires it to be done during training, and one must augment all images.24 et al 2020c & et al 2020 observe, with regular GAN training, there is a striking steady decline of D performance on heldout data, and increase on training data, throughout the course of training, confirming the BigGAN observation but also showing it is a dynamic phenomenon, and probably a bad one. Adding in correct data augmentation reduces this overfitting—and markedly improves sample-efficiency & final quality. This suggests that the D does indeed memorize, but that this is not a good thing. et al 2020 describes what happens as
Convergence is now achieved [with ADA/data augmentation] regardless of the training set size and overfitting no longer occurs. Without augmentations, the gradients the generator receives from the discriminator become very simplistic over time—the discriminator starts to pay attention to only a handful of features, and the generator is free to create otherwise nonsensical images. With ADA, the gradient field stays much more detailed which prevents such deterioration.
In other words, just as the G can ‘mode collapse’ by focusing on generating images with only a few features, the D can also ‘feature collapse’ by focusing on a few features which happen to correctly split the training data’s reals from fakes, such as by memorizing them outright. This technically works, but not well. This also explains why BigGAN training stabilized when training on JFT-300M: divergence/collapse usually starts with D winning; if D wins because it memorizes, then a sufficiently large dataset should make memorization infeasible; and JFT-300M turns out to be sufficiently large. (This would predict that if Brock et al had checked the JFT-300M BigGAN D’s classification performance on a held-out JFT-300M, rather than just on their ImageNet BigGAN, then they would have found that it classified reals vs fake well above chance.) Perhaps the way GANs work is that the G is constantly trying to generate samples which are near real datapoints, but then the D ‘repels’ samples too near a real datapoint, so G orbits the real datapoints; the D successfully memorizing distinguishing features of each real datapoint breaks the dynamics because it can either always detect G’s samples or G can get arbitrarily close & it memorize. (et al 2018 suggests that Discriminators may need to grow large for GANs to be able to learn many datapoints; to me this suggests that there may be useful ways to increase the capacity of Discriminators which are not simply increasing parameter size. For example, by analogy to minibatch discrimination, what we might call minibatch retrieval using retrieval methods to boost Discriminator capacity by retrieving the real nearest-neighbors of every generated sample to pass into the Discriminator to make its job much easier. By adding in hard negatives from the dataset—at scale, minibatch discrimination would stop being useful because it is ever more unlikely, even with minibatches of thousands of images like BigGAN, that any of the other images in the minibatch are all that similar to a fake or exhibit the same flaws. This could be seen as parallel to retrieval diffusion models. See also “novelty nets”.)
If so, this suggests that for D ranking, it may not be too useful to take the D from the end of a run, if not using data augmentation, because that D be the version with the greatest degree of memorization!
Here is a simple StyleGAN2 script (ranker.py
) to open a StyleGAN .pkl
and run it on a list of image filenames to print out the D score, courtesy of Shao Xuning:
import pickle
import numpy as np
import cv2
import dnnlib.tflib as tflib
import random
import argparse
import PIL.Image
from training.misc import adjust_dynamic_range
def preprocess(file_path):
# print(file_path)
img = np.asarray(PIL.Image.open(file_path))
# Preprocessing from dataset_tool.create_from_images
img = img.transpose([2, 0, 1]) # HWC => CHW
# img = np.expand_dims(img, axis=0)
img = img.reshape((1, 3, 512, 512))
# Preprocessing from training_loop.process_reals
img = adjust_dynamic_range(data=img, drange_in=[0, 255], drange_out=[-1.0, 1.0])
return img
def main(args):
random.seed(args.random_seed)
minibatch_size = args.minibatch_size
input_shape = (minibatch_size, 3, 512, 512)
# print(args.images)
images = args.images
images.sort()
tflib.init_tf()
_G, D, _Gs = pickle.load(open(args.model, "rb"))
# D.print_layers()
image_score_all = [(image, []) for image in images]
# Shuffle the images and process each image in multiple minibatches.
# Note: networks.stylegan2.minibatch_stddev_layer
# calculates the standard deviation of a minibatch group as a feature channel,
# which means that the output of the discriminator actually depends
# on the companion images in the same minibatch.
for i_shuffle in range(args.num_shuffles):
# print('shuffle: {}'.format(i_shuffle))
random.shuffle(image_score_all)
for idx_1st_img in range(0, len(image_score_all), minibatch_size):
idx_img_minibatch = []
images_minibatch = []
input_minibatch = np.zeros(input_shape)
for i in range(minibatch_size):
idx_img = (idx_1st_img + i) % len(image_score_all)
idx_img_minibatch.append(idx_img)
image = image_score_all[idx_img][0]
images_minibatch.append(image)
img = preprocess(image)
input_minibatch[i, :] = img
output = D.run(input_minibatch, None, resolution=512)
print('shuffle: {}, indices: {}, images: {}'
.format(i_shuffle, idx_img_minibatch, images_minibatch))
print('Output: {}'.format(output))
for i in range(minibatch_size):
idx_img = idx_img_minibatch[i]
image_score_all[idx_img][1].append(output[i][0])
with open(args.output, 'a') as fout:
for image, score_list in image_score_all:
print('Image: {}, score_list: {}'.format(image, score_list))
avg_score = sum(score_list)/len(score_list)
fout.write(image + ' ' + str(avg_score) + '\n')
def parse_arguments():
parser = argparse.ArgumentParser()
parser.add_argument('--model', type=str, required=True,
help='.pkl model')
parser.add_argument('--images', nargs='+')
parser.add_argument('--output', type=str, default='rank.txt')
parser.add_argument('--minibatch_size', type=int, default=4)
parser.add_argument('--num_shuffles', type=int, default=5)
parser.add_argument('--random_seed', type=int, default=0)
return parser.parse_args()
if __name__ == '__main__':
main(parse_arguments())
Depending on how noisy the rankings are in terms of ‘quality’ and available sample size, one can either review the worst-ranked images by hand, or delete the bottom X%. One should check the top-ranked images as well to make sure the ordering is right; there can also be some odd images in the top X% as well which should be removed.
It might be possible to use ranker.py
to improve the quality of generated samples as well, as a simple version of discriminator rejection sampling.
Upscaling
The next major step is upscaling images using waifu2x
, which does an excellent job on 2× upscaling of anime images, which are nigh-indistinguishable from a higher-resolution original and greatly increase the usable corpus. The downside is that it can take 1–10s per image, must run on the GPU (I can reliably fit ~9 instances on my 2×1080ti), and is written in a now-unmaintained DL framework, Torch, with no current plans to port to PyTorch, and is gradually becoming harder to get running (one hopes that by the time CUDA updates break it entirely, there will be another super-resolution GAN I or someone else can train on Danbooru to replace it). If pressed for time, one can just upscale the faces normally with ImageMagick but I believe there will be some quality loss and it’s worthwhile.
. ~/src/torch/install/bin/torch-activate
upscaleWaifu2x() {
SIZE1=$(identify -format "%h" "$@")
SIZE2=$(identify -format "%w" "$@");
if (( $SIZE1 < 512 && $SIZE2 < 512 )); then
echo "$@" $SIZE
TMP=$(mktemp "/tmp/XXXXXX.png")
CUDA_VISIBLE_DEVICES="$((RANDOM % 2 < 1))" nice th ~/src/waifu2x/waifu2x.lua -model_dir \
~/src/waifu2x/models/upconv_7/art -tta 1 -m scale -scale 2 \
-i "$@" -o "$TMP"
convert "$TMP" "$@"
rm "$TMP"
fi; }
export -f upscaleWaifu2x
find faces/ -type f | parallel --progress --jobs 9 upscaleWaifu2x
Quality Checks & Data Augmentation
The single most effective strategy to improve a GAN is to clean the data. StyleGAN cannot handle too-diverse datasets composed of multiple objects or single objects shifted around, and rare or odd images cannot be learned well. Karras et al get such good results with StyleGAN on faces in part because they constructed FFHQ to be an extremely clean consistent dataset of just centered well-lit clear human faces without any obstructions or other variation. Similarly, Arfa’s “This Fursona Does Not Exist” (TFDNE) S2 generates much better furry portraits than my own “This Waifu Does Not Exist” (TWDNE) S2 anime portraits, due partly to training longer to convergence on a TPU pod but mostly due to his investment in data cleaning: aligning the faces and heavy filtering of samples—this left him with only n = 50k but TFDNE nevertheless outperforms TWDNE’s n = 300k. (Data cleaning/augmentation is one of the more powerful ways to improve results; if we imagine deep learning as ‘programming’ or ‘Software 2.0’25 in Andrej Karpathy’s terms, data cleaning/augmentation is one of the easiest ways to finetune the loss function towards what we really want by gardening our data to remove what we don’t want and increase what we do.)
At this point, one can do manual quality checks by viewing a few hundred images, running findimagedupes -t 99%
to look for near-identical faces, or dabble in further modifications such as doing “data augmentation”. Working with Danbooru2018, at this point one would have ~600–700,000 faces, which is more than enough to train StyleGAN and one will have difficulty storing the final StyleGAN dataset because of its sheer size (due to the ~18× size multiplier). After cleaning etc, my final face dataset is the portrait dataset with n = 300k.
However, if that is not enough or one is working with a small dataset like for a single character, data augmentation may be necessary. The mirror/horizontal flip is not necessary as StyleGAN has that built-in as an option26, but there are many other possible data augmentations. One can stretch, shift colors, sharpen, blur, increase/decrease contrast/brightness, crop, and so on. An example, extremely aggressive, set of data augmentations could be done like this:
dataAugment () {
image="$@"
target=$(basename "$@")
suffix="png"
convert -deskew 50 "$image" "$target".deskew."$suffix"
convert -resize 110%x100% "$image" "$target".horizstretch."$suffix"
convert -resize 100%x110% "$image" "$target".vertstretch."$suffix"
convert -blue-shift 1.1 "$image" "$target".midnight."$suffix"
convert -fill red -colorize 5% "$image" "$target".red."$suffix"
convert -fill orange -colorize 5% "$image" "$target".orange."$suffix"
convert -fill yellow -colorize 5% "$image" "$target".yellow."$suffix"
convert -fill green -colorize 5% "$image" "$target".green."$suffix"
convert -fill blue -colorize 5% "$image" "$target".blue."$suffix"
convert -fill purple -colorize 5% "$image" "$target".purple."$suffix"
convert -adaptive-blur 3x2 "$image" "$target".blur."$suffix"
convert -adaptive-sharpen 4x2 "$image" "$target".sharpen."$suffix"
convert -brightness-contrast 10 "$image" "$target".brighter."$suffix"
convert -brightness-contrast 10x10 "$image" "$target".brightercontraster."$suffix"
convert -brightness-contrast -10 "$image" "$target".darker."$suffix"
convert -brightness-contrast -10x10 "$image" "$target".darkerlesscontrast."$suffix"
convert +level 5% "$image" "$target".contraster."$suffix"
convert -level 5%\! "$image" "$target".lesscontrast."$suffix"
}
export -f dataAugment
find faces/ -type f | parallel --progress dataAugment
Upscaling & Conversion
Once any quality fixes or data augmentation are done, it’d be a good idea to save a lot of disk space by converting to JPG & lossily reducing quality (I find 33% saves a ton of space at no visible change):
convertPNGToJPG() { convert -quality 33 "$@" "$@".jpg && rm "$@"; }
export -f convertPNGToJPG
find faces/ -type f -name "*.png" | parallel --progress convertPNGToJPG
Remember that StyleGAN models are only compatible with images of the type they were trained on, so if you are using a StyleGAN pretrained model which was trained on PNGs (like, IIRC, the FFHQ StyleGAN models), you will need to keep using PNGs.
Doing the final scaling to exactly 512px can be done at many points but I generally postpone it to the end in order to work with images in their ‘native’ resolutions & aspect-ratios for as long as possible. At this point we carefully tell ImageMagick to rescale everything to 512×51227, not preserving the aspect ratio by filling in with a black background as necessary on either side:
find faces/ -type f | xargs --max-procs=16 -n 9000 \
mogrify -resize 512x512\> -extent 512x512\> -gravity center -background black
Any slightly-different image could crash the import process. Therefore, we delete any image which is even slightly different from the 512×512 sRGB JPG they are supposed to be:
find faces/ -type f | xargs --max-procs=16 -n 9000 identify | \
# remember the warning: images must be identical, square, and sRGB/grayscale:
grep -F -v " JPEG 512x512 512x512+0+0 8-bit sRGB"| cut -d ' ' -f 1 | \
xargs --max-procs=16 -n 10000 rm
Having done all this, we should have a large consistent high-quality dataset.
Finally, the faces can now be converted to the ProGAN or StyleGAN dataset format using dataset_tool.py
. It is worth remembering at this point how fragile that is and the requirements ImageMagick’s identify
command is handy for looking at files in more details, particularly their resolution & colorspace, which are often the problem.
Because of the extreme fragility of dataset_tool.py
, I strongly advise that you edit it to print out the filenames of each file as they are being processed so that when (not if) it crashes, you can investigate the culprit and check the rest. The edit could be as simple as this:
diff --git a/dataset_tool.py b/dataset_tool.py
index 4ddfe44..e64e40b 100755
--- a/dataset_tool.py
+++ b/dataset_tool.py
@@ -519,6 +519,7 @@ def create_from_images(tfrecord_dir, image_dir, shuffle):
with TFRecordExporter(tfrecord_dir, len(image_filenames)) as tfr:
order = tfr.choose_shuffled_order() if shuffle else np.arange(len(image_filenames))
for idx in range(order.size):
+ print(image_filenames[order[idx]])
img = np.asarray(PIL.Image.open(image_filenames[order[idx]]))
if channels == 1:
img = img[np.newaxis, :, :] # HW => CHW
There should be no issues if all the images were thoroughly checked earlier, but should any images crash it, they can be checked in more detail by identify
. (I advise just deleting them and not trying to rescue them.)
Then the conversion is just (assuming StyleGAN prerequisites are installed, see next section):
source activate MY_TENSORFLOW_ENVIRONMENT
python dataset_tool.py create_from_images datasets/faces /media/gwern/Data/danbooru2018/faces/
Congratulations, the hardest part is over. Most of the rest simply requires patience (and a willingness to edit Python files directly in order to configure StyleGAN).
Training
Installation
I assume you have CUDA installed & functioning. If not, good luck. (On my Ubuntu Bionic 18.04.2 LTS OS, I have successfully used the Nvidia driver version #410.104, CUDA 10.1, and TensorFlow 1.13.1.)
A Python ≥3.628 virtual environment can be set up for StyleGAN to keep dependencies tidy, TensorFlow & StyleGAN dependencies installed:
conda create -n stylegan pip python=3.6
source activate stylegan
## TF:
pip install tensorflow-gpu
## Test install:
python -c "import tensorflow as tf; tf.enable_eager_execution(); \
print(tf.reduce_sum(tf.random_normal([1000, 1000])))"
pip install tensorboard
## StyleGAN:
## Install pre-requisites:
pip install pillow numpy moviepy scipy opencv-python lmdb # requests?
## Download:
git clone 'https://github.com/NVlabs/stylegan.git' && cd ./stylegan/
## Test install:
python pretrained_example.py
## ./results/example.png should be a photograph of a middle-aged man
StyleGAN can also be trained on the interactive Google Colab service, which provides free slices of K80 GPUs 12-GPU-hour chunks, using this Colab notebook. Colab is much slower than training on a local machine & the free instances are not enough to train the best StyleGANs, but this might be an useful option for people who simply want to try it a little or who are doing something quick like extremely low-resolution training or transfer-learning where a few GPU-hours on a slow small GPU might be enough.
Configuration
StyleGAN doesn’t ship with any support for CLI options; instead, one must edit train.py
and train/training_loop.py
:
-
train/training_loop.py
The core configuration is done in the function defaults to
training_loop
beginning line 112.The key arguments are
G_smoothing_kimg
&D_repeats
(affects the learning dynamics),network_snapshot_ticks
(how often to save the pickle snapshots—more frequent means less progress lost in crashes, but as each one weighs 300MB+, can quickly use up gigabytes of space),resume_run_id
(set to"latest"
), andresume_kimg
.Don’t Erase Your Model
resume_kimg
governs where in the overall progressive-growing training schedule StyleGAN starts from. If it is set to 0, training begins at the beginning of the progressive-growing schedule, at the lowest resolution, regardless of how much training has been previously done. It is vitally important when doing transfer learning that it is set to a sufficiently high number (eg. 10000) that training begins at the highest desired resolution like 512px, as it appears that layers are erased when added during progressive-growing. (resume_kimg
may also need to be set to a high value to make it skip straight to training at the highest resolution if you are training on small datasets of small images, where there’s risk of it overfitting under the normal training schedule and never reaching the highest resolution.) This trick is unnecessary in StyleGAN 2, which is simpler in not using progressive growing.More experimentally, I suggest setting
minibatch_repeats = 1
instead ofminibatch_repeats = 5
; in line with the suspiciousness of the gradient-accumulation implementation in ProGAN/StyleGAN, this appears to make training both stabler & faster.Note that some of these variables, like learning rates, are overridden in
train.py
. It’s better to set those there or else you may confuse yourself badly (like I did in wondering why ProGAN & StyleGAN seemed extraordinarily robust to large changes in the learning rates…). -
train.py
(previouslyconfig.py
in ProGAN; renamedrun_training.py
in StyleGAN 2)Here we set the number of GPUs, image resolution, dataset, learning rates, horizontal flipping/mirroring data augmentation, and minibatch sizes. (This file includes settings intended ProGAN—watch out that you don’t accidentally turn on ProGAN instead of StyleGAN & confuse yourself.) Learning rate & minibatch should generally be left alone (except towards the end of training when one wants to lower the learning rate to promote convergence or rebalance the G/D), but the image resolution/dataset/mirroring do need to be set, like thus:
desc += '-faces'; dataset = EasyDict(tfrecord_dir='faces', resolution=512); train.mirror_augment = True
This sets up the 512px face dataset which was previously created in
dataset/faces
, turns on mirroring (because while there may be writing in the background, we don’t care about it for face generation), and sets a title for the checkpoints/logs, which will now appear inresults/
with the ‘-faces’ string.Assuming you do not have 8 GPUs (as you probably do not), you must change the
-preset
to match your number of GPUs, StyleGAN will not automatically choose the correct number of GPUs. If you fail to set it correctly to the appropriate preset, StyleGAN will attempt to use GPUs which do not exist and will crash with the opaque error message (note that CUDA uses zero-indexing soGPU:0
refers to the first GPU,GPU:1
refers to my second GPU, and thus/device:GPU:2
refers to my—nonexistent—third GPU):tensorflow.python.framework.errors_impl.InvalidArgumentError: Cannot assign a device for operation \ G_synthesis_3/lod: {{node G_synthesis_3/lod}}was explicitly assigned to /device:GPU:2 but available \ devices are [ /job:localhost/replica:0/task:0/device:CPU:0, /job:localhost/replica:0/task:0/device:GPU:0, \ /job:localhost/replica:0/task:0/device:GPU:1, /job:localhost/replica:0/task:0/device:XLA_CPU:0, \ /job:localhost/replica:0/task:0/device:XLA_GPU:0, /job:localhost/replica:0/task:0/device:XLA_GPU:1 ]. \ Make sure the device specification refers to a valid device. [[{{node G_synthesis_3/lod}}]]
For my 2×1080ti I’d set:
desc += '-preset-v2-2gpus'; submit_config.num_gpus = 2; sched.minibatch_base = 8; sched.minibatch_dict = \ {4: 256, 8: 256, 16: 128, 32: 64, 64: 32, 128: 16, 256: 8}; sched.G_lrate_dict = {512: 0.0015, 1024: 0.002}; \ sched.D_lrate_dict = EasyDict(sched.G_lrate_dict); train.total_kimg = 99000
So my results get saved to
results/00001-sgan-faces-2gpu
etc (the run ID increments, ‘sgan’ because StyleGAN rather than ProGAN, ‘-faces’ as the dataset being trained on, and ‘2gpu’ because it’s multi-GPU).
Running
I typically run StyleGAN in a screen
session which can be detached and keeps multiple shells organized: 1 terminal/shell for the StyleGAN run, 1 terminal/shell for TensorBoard, and 1 for Emacs.
With Emacs, I keep the two key Python files open (train.py
and train/training_loop.py
) for reference & easy editing.
With the “latest” patch, StyleGAN can be thrown into a while-loop to keep running after crashes, like:
while true; do nice py train.py; date; (xmessage "alert: StyleGAN crashed" &); sleep 10s; done
TensorBoard is a logging utility which displays little time-series of recorded variables which one views in a web browser, eg:
tensorboard --logdir results/02022-sgan-faces-2gpu/
# TensorBoard 1.13.0 at http://127.0.0.1:6006 (Press CTRL+C to quit)
Note that TensorBoard can be backgrounded, but needs to be updated every time a new run is started as the results will then be in a different folder.
Training StyleGAN is much easier & more reliable than other GANs, but it is still more of an art than a science. (We put up with it because while GANs suck, everything else sucks more.) Notes on training:
-
Crashproofing:
The initial release of StyleGAN was prone to crashing when I ran it, segfaulting at random. Updating TensorFlow appeared to reduce this but the root cause is still unknown. Segfaulting or crashing is also reportedly common if running on mixed GPUs (eg. a 1080ti + Titan V).
Unfortunately, StyleGAN has no setting for simply resuming from the latest snapshot after crashing/exiting (which is what one usually wants), and one must manually edit the
resume_run_id
line intraining_loop.py
to set it to the latest run ID. This is tedious and error-prone—at one point I realized I had wasted 6 GPU-days of training by restarting from a 3-day-old snapshot because I had not updated theresume_run_id
after a segfault!If you are doing any runs longer than a few wallclock hours, I strongly advise use of nshepperd’s patch to automatically restart from the latest snapshot by setting
resume_run_id = "latest"
:diff --git a/training/misc.py b/training/misc.py index 50ae51c..d906a2d 100755 --- a/training/misc.py +++ b/training/misc.py @@ -119,6 +119,14 @@ def list_network_pkls(run_id_or_run_dir, include_final=True): del pkls[0] return pkls +def locate_latest_pkl(): + allpickles = sorted(glob.glob(os.path.join(config.result_dir, '0*', 'network-*.pkl'))) + latest_pickle = allpickles[-1] + resume_run_id = os.path.basename(os.path.dirname(latest_pickle)) + RE_KIMG = re.compile('network-snapshot-(\d+).pkl') + kimg = int(RE_KIMG.match(os.path.basename(latest_pickle)).group(1)) + return (locate_network_pkl(resume_run_id), float(kimg)) + def locate_network_pkl(run_id_or_run_dir_or_network_pkl, snapshot_or_network_pkl=None): for candidate in [snapshot_or_network_pkl, run_id_or_run_dir_or_network_pkl]: if isinstance(candidate, str): diff --git a/training/training_loop.py b/training/training_loop.py index 78d6fe1..20966d9 100755 --- a/training/training_loop.py +++ b/training/training_loop.py @@ -148,7 +148,10 @@ def training_loop( # Construct networks. with tf.device('/gpu:0'): if resume_run_id is not None: - network_pkl = misc.locate_network_pkl(resume_run_id, resume_snapshot) + if resume_run_id == 'latest': + network_pkl, resume_kimg = misc.locate_latest_pkl() + else: + network_pkl = misc.locate_network_pkl(resume_run_id, resume_snapshot) print('Loading networks from "%s"...' % network_pkl) G, D, Gs = misc.load_pkl(network_pkl) else:
(The diff can be edited by hand, or copied into the repo as a file like
latest.patch
& then applied withgit apply latest.patch
.) -
Tuning Learning Rates
The LR is one of the most critical hyperparameters: too-large updates based on too-small minibatches are devastating to GAN stability & final quality. The LR also seems to interact with the intrinsic difficulty or diversity of an image domain; et al 2019 use 0.003 G/D LRs on their FFHQ dataset (which has been carefully curated and the faces aligned to put landmarks like eyes/mouth in the same locations in every image) when training on 8-GPU machines with minibatches of n = 32, but I find lower to be better on my anime face/portrait datasets where I can only do n = 8. From looking at training videos of whole-Danbooru2018 StyleGAN runs, I suspect that the necessary LRs would be lower still. Learning rates are closely related to minibatch size (a common rule of thumb in supervised learning of CNNs is that the relationship of biggest usable LR follows a square-root curve in minibatch size) and the BigGAN research argues that minibatch size itself strongly influences how bad mode dropping is, which suggests that smaller LRs may be more necessary the more diverse/difficult a dataset is.
-
Balancing G/D:
Later in training, if the G is not making good progress towards the ultimate goal of a 0.5 loss (and the D’s loss gradually decreasing towards 0.5), and has a loss stubbornly stuck around −1 or something, it may be necessary to change the balance of G/D. This can be done several ways but the easiest is to adjust the LRs in
train.py
,sched.G_lrate_dict
&sched.D_lrate_dict
.One needs to keep an eye on the G/D losses and also the perceptual quality of the faces (since we don’t have any good FID equivalent yet for anime faces, which requires a good open-source Danbooru tagger to create embeddings), and reduce both LRs (or usually just the D’s LR) based on the face quality and whether the G/D losses are exploding or otherwise look imbalanced. What you want, I think, is for the G/D losses to be stable at a certain absolute amount for a long time while the quality visibly improves, reducing D’s LR as necessary to keep it balanced with G; and then once you’ve run out of time/patience or artifacts are showing up, then you can decrease both LRs to converge onto a local optima.
I find the default of 0.003 can be too high once quality reaches a high level with both faces & portraits, and it helps to reduce it by a third to 0.001 or a tenth to 0.0003. If there still isn’t convergence, the D may be too strong and it can be turned down separately, to a tenth or a fiftieth even. (Given the stochasticity of training & the relativity of the losses, one should wait several wallclock hours or days after each modification to see if it made a difference.)
-
Skipping FID metrics:
Some metrics are computed for logging/reporting. The FID metrics are calculated using an old ImageNet CNN; what is realistic on ImageNet may have little to do with your particular domain and while a large FID like 100 is concerning, FIDs like 20 or even increasing are not necessarily a problem or useful guidance compared to just looking at the generated samples or the loss curves. Given that computing FID metrics is not free & potentially irrelevant or misleading on many image domains, I suggest disabling them entirely. (They are not used in the training for anything, and disabling them is safe.)
They can be edited out of the main training loop by commenting out the call to
metrics.run
like so:@@ -261,7 +265,7 @@ def training_loop() if cur_tick % network_snapshot_ticks == 0 or done or cur_tick == 1: pkl = os.path.join(submit_config.run_dir, 'network-snapshot-%06d.pkl' % (cur_nimg // 1000)) misc.save_pkl((G, D, Gs), pkl) # metrics.run(pkl, run_dir=submit_config.run_dir, num_gpus=submit_config.num_gpus, tf_config=tf_config)
-
‘Blob’ & ‘Crack’ Artifacts:
During training, ‘blobs’ often show up or move around. These blobs appear even late in training on otherwise high-quality images and are unique to StyleGAN (at least, I’ve never seen another GAN whose training artifacts look like the blobs). That they are so large & glaring suggests a weakness in StyleGAN somewhere. The source of the blobs was unclear. If you watch training videos, these blobs seem to gradually morph into new features such as eyes or hair or glasses. I suspect they are part of how StyleGAN ‘creates’ new features, starting with a feature-less blob superimposed at approximately the right location, and gradually refined into something useful. The StyleGAN 2 paper investigated the blob artifacts & found it to be due to the Generator working around a flaw in StyleGAN’s use of AdaIN normalization. et al 2019 note that images without a blob somewhere are severely corrupted; because the blobs are in fact doing something useful, it is unsurprising that the Discriminator doesn’t fix the Generator. StyleGAN 2 changes the AdaIN normalization to eliminate this problem, improving overall quality.29
If blobs are appearing too often or one wants a final model without any new intrusive blobs, it may help to lower the LR to try to converge to a local optima where the necessary blob is hidden away somewhere unobtrusive.
In training anime faces, I have seen additional artifacts, which look like ‘cracks’ or ‘waves’ or elephant skin wrinkles or the sort of fine crazing seen in old paintings or ceramics, which appear toward the end of training on primarily skin or areas of flat color; they happen particularly fast when transfer learning on a small dataset. The only solution I have found so far is to either stop training or get more data. In contrast to the blob artifacts (identified as an architectural problem & fixed in StyleGAN 2), I currently suspect the cracks are a sign of overfitting rather than a peculiarity of normal StyleGAN training, where the G has started trying to memorize noise in the fine detail of pixelation/lines, and so these are a kind of overfitting/mode collapse. (More speculatively: another possible explanation is that the cracks are caused by the StyleGAN D being single-scale rather than multi-scale—as in MSG-GAN and a number of others—and the ‘cracks’ are actually high-frequency noise created by the G in specific patches as adversarial examples to fool the D. They reportedly do not appear in MSG-GAN or StyleGAN 2, which both use multi-scale Ds.)
-
Gradient Accumulation:
ProGAN/StyleGAN’s codebase claims to support gradient accumulation, which is a way to fake large minibatch training (eg. n = 2048) by not doing the backpropagation update every minibatch, but instead summing the gradients over many minibatches and applying them all at once. This is an useful trick for stabilizing training, and large minibatch NN training can differ qualitatively from small minibatch NN training—BigGAN performance increased with increasingly large minibatches (n = 2048) and the authors speculate that this is because such large minibatches mean that the full diversity of the dataset is represented in each ‘minibatch’ so the BigGAN models cannot simply ‘forget’ rarer datapoints which would otherwise not appear for many minibatches in a row, resulting in the GAN pathology of ‘mode dropping’ where some kinds of data just get ignored by both G/D.
However, the ProGAN/StyleGAN implementation of gradient accumulation does not resemble that of any other implementation I’ve seen in TensorFlow or PyTorch, and in my own experiments with up to n = 4096, I didn’t observe any stabilization or qualitative differences, so I am suspicious the implementation is wrong.
Here is what a successful training progression looks like for the anime face StyleGAN:
Training montage video of the first 9k iterations of the anime face StyleGAN.
The anime face model is obsoleted by the StyleGAN 2 portrait model.
The anime face model as of 2019-03-08, trained for 21,980 iterations or ~21m images or ~38 GPU-days, is available for download. (It is still not fully-converged, but the quality is good.)
Sampling
Having successfully trained a StyleGAN, now the fun part—generating samples!
Psi/“Truncation Trick”
The Ψ/“truncation trick” (BigGAN discussion, StyleGAN discussion; apparently first introduced by 2017) is the most important hyperparameter for all StyleGAN generation.
The truncation trick is used at sample generation time but not training time. The idea is to edit the latent vector z, which is a vector of 𝒩(0,1), to remove any variables which are above a certain size like 0.5 or 1.0, and resample those.30 This seems to help by avoiding ‘extreme’ latent values or combinations of latent values which the G is not as good at—a G will not have generated many data points with each latent variable at, say, +1.5SD. The tradeoff is that those are still legitimate areas of the overall latent space which were being used during training to cover parts of the data distribution; so while the latent variables close to the mean of 0 may be the most accurately modeled, they are also only a small part of the space of all possible images. So one can generate latent variables from the full unrestricted 𝒩(0,1) distribution for each one, or one can truncate them at something like +1SD or +0.7SD. (Like the discussion of the best distribution for the original latent distribution, there’s no good reason to think that this is an optimal method of doing truncation; there are many alternatives, such as ones penalizing the sum of the variables, either rejecting them or scaling them down, and some appear to work much better than the current truncation trick.)
At Ψ = 0, diversity is nil and all faces are a single global average face (a brown-eyed brown-haired schoolgirl, unsurprisingly); at ±0.5 you have a broad range of faces, and by ±1.2, you’ll see tremendous diversity in faces/styles/consistency but also tremendous artifacting & distortion. Where you set your Ψ will heavily influence how ‘original’ outputs look. At Ψ = 1.2, they are tremendously original but extremely hit or miss. At Ψ = 0.5 they are consistent but boring. For most of my sampling, I set Ψ = 0.7 which strikes the best balance between craziness/artifacting and quality/diversity. (Personally, I prefer to look at Ψ = 1.2 samples because they are so much more interesting, but if I released those samples, it would give a misleading impression to readers.)
Random Samples
The StyleGAN repo has a simple script pretrained_example.py
to download & generate a single face; in the interests of reproducibility, it hardwires the model and the RNG seed so it will only generate 1 particular face. However, it can be easily adapted to use a local model and (slowly31) generate, say, 1000 sample images with the hyperparameter Ψ = 0.6 (which gives high-quality but not highly-diverse images) which are saved to results/example-{0-999}.png
:
import os
import pickle
import numpy as np
import PIL.Image
import dnnlib
import dnnlib.tflib as tflib
import config
def main():
tflib.init_tf()
_G, _D, Gs = pickle.load(open("results/02051-sgan-faces-2gpu/network-snapshot-021980.pkl", "rb"))
Gs.print_layers()
for i in range(0,1000):
rnd = np.random.RandomState(None)
latents = rnd.randn(1, Gs.input_shape[1])
fmt = dict(func=tflib.convert_images_to_uint8, nchw_to_nhwc=True)
images = Gs.run(latents, None, truncation_psi=0.6, randomize_noise=True, output_transform=fmt)
os.makedirs(config.result_dir, exist_ok=True)
png_filename = os.path.join(config.result_dir, 'example-'+str(i)+'.png')
PIL.Image.fromarray(images[0], 'RGB').save(png_filename)
if __name__ == "__main__":
main()
Et Al 2018 Figures
The figures in et al 2018, demonstrating random samples and aspects of the style noise using the 1024px FFHQ face model (as well as the others), were generated by generate_figures.py
. This script needs extensive modifications to work with my 512px anime face; going through the file:
-
the code uses Ψ = 1 truncation, but faces look better with Ψ = 0.7 (several of the functions have
truncation_psi=
settings but, trickily, the Figure 3draw_style_mixing_figure
has its Ψ setting hidden away in thesynthesis_kwargs
global variable) -
the loaded model needs to be switched to the anime face model, of course
-
dimensions must be reduced 1024→512 as appropriate; some ranges are hardcoded and must be reduced for 512px images as well
-
the truncation trick figure 8 doesn’t show enough faces to give insight into what the latent space is doing so it needs to be expanded to show both more random seeds/faces, and more Ψ values
-
the bedroom/car/cat samples should be disabled
The changes I make are as follows:
diff --git a/generate_figures.py b/generate_figures.py
index 45b68b8..f27af9d 100755
--- a/generate_figures.py
+++ b/generate_figures.py
@@ -24,16 +24,13 @@ url_bedrooms = 'https://drive.google.com/uc?id=1MOSKeGF0FJcivpBI7s63V9YHloUTO
url_cars = 'https://drive.google.com/uc?id=1MJ6iCfNtMIRicihwRorsM3b7mmtmK9c3' # karras2019stylegan-cars-512x384.pkl
url_cats = 'https://drive.google.com/uc?id=1MQywl0FNt6lHu8E_EUqnRbviagS7fbiJ' # karras2019stylegan-cats-256x256.pkl
-synthesis_kwargs = dict(output_transform=dict(func=tflib.convert_images_to_uint8, nchw_to_nhwc=True), minibatch_size=8)
+synthesis_kwargs = dict(output_transform=dict(func=tflib.convert_images_to_uint8, nchw_to_nhwc=True), minibatch_size=8, truncation_psi=0.7)
_Gs_cache = dict()
def load_Gs(url):
- if url not in _Gs_cache:
- with dnnlib.util.open_url(url, cache_dir=config.cache_dir) as f:
- _G, _D, Gs = pickle.load(f)
- _Gs_cache[url] = Gs
- return _Gs_cache[url]
+ _G, _D, Gs = pickle.load(open("results/02051-sgan-faces-2gpu/network-snapshot-021980.pkl", "rb"))
+ return Gs
#----------------------------------------------------------------------------
# Figures 2, 3, 10, 11, 12: Multi-resolution grid of uncurated result images.
@@ -85,7 +82,7 @@ def draw_noise_detail_figure(png, Gs, w, h, num_samples, seeds):
canvas = PIL.Image.new('RGB', (w * 3, h * len(seeds)), 'white')
for row, seed in enumerate(seeds):
latents = np.stack([np.random.RandomState(seed).randn(Gs.input_shape[1])] * num_samples)
- images = Gs.run(latents, None, truncation_psi=1, **synthesis_kwargs)
+ images = Gs.run(latents, None, **synthesis_kwargs)
canvas.paste(PIL.Image.fromarray(images[0], 'RGB'), (0, row * h))
for i in range(4):
crop = PIL.Image.fromarray(images[i + 1], 'RGB')
@@ -109,7 +106,7 @@ def draw_noise_components_figure(png, Gs, w, h, seeds, noise_ranges, flips):
all_images = []
for noise_range in noise_ranges:
tflib.set_vars({var: val * (1 if i in noise_range else 0) for i, (var, val) in enumerate(noise_pairs)})
- range_images = Gsc.run(latents, None, truncation_psi=1, randomize_noise=False, **synthesis_kwargs)
+ range_images = Gsc.run(latents, None, randomize_noise=False, **synthesis_kwargs)
range_images[flips, :, :] = range_images[flips, :, ::-1]
all_images.append(list(range_images))
@@ -144,14 +141,11 @@ def draw_truncation_trick_figure(png, Gs, w, h, seeds, psis):
def main():
tflib.init_tf()
os.makedirs(config.result_dir, exist_ok=True)
- draw_uncurated_result_figure(os.path.join(config.result_dir, 'figure02-uncurated-ffhq.png'), load_Gs(url_ffhq), cx=0, cy=0, cw=1024, ch=1024, rows=3, lods=[0,1,2,2,3,3], seed=5)
- draw_style_mixing_figure(os.path.join(config.result_dir, 'figure03-style-mixing.png'), load_Gs(url_ffhq), w=1024, h=1024, src_seeds=[639,701,687,615,2268], dst_seeds=[888,829,1898,1733,1614,845], style_ranges=[range(0,4)]*3+[range(4,8)]*2+[range(8,18)])
- draw_noise_detail_figure(os.path.join(config.result_dir, 'figure04-noise-detail.png'), load_Gs(url_ffhq), w=1024, h=1024, num_samples=100, seeds=[1157,1012])
- draw_noise_components_figure(os.path.join(config.result_dir, 'figure05-noise-components.png'), load_Gs(url_ffhq), w=1024, h=1024, seeds=[1967,1555], noise_ranges=[range(0, 18), range(0, 0), range(8, 18), range(0, 8)], flips=[1])
- draw_truncation_trick_figure(os.path.join(config.result_dir, 'figure08-truncation-trick.png'), load_Gs(url_ffhq), w=1024, h=1024, seeds=[91,388], psis=[1, 0.7, 0.5, 0, -0.5, -1])
- draw_uncurated_result_figure(os.path.join(config.result_dir, 'figure10-uncurated-bedrooms.png'), load_Gs(url_bedrooms), cx=0, cy=0, cw=256, ch=256, rows=5, lods=[0,0,1,1,2,2,2], seed=0)
- draw_uncurated_result_figure(os.path.join(config.result_dir, 'figure11-uncurated-cars.png'), load_Gs(url_cars), cx=0, cy=64, cw=512, ch=384, rows=4, lods=[0,1,2,2,3,3], seed=2)
- draw_uncurated_result_figure(os.path.join(config.result_dir, 'figure12-uncurated-cats.png'), load_Gs(url_cats), cx=0, cy=0, cw=256, ch=256, rows=5, lods=[0,0,1,1,2,2,2], seed=1)
+ draw_uncurated_result_figure(os.path.join(config.result_dir, 'figure02-uncurated-ffhq.png'), load_Gs(url_ffhq), cx=0, cy=0, cw=512, ch=512, rows=3, lods=[0,1,2,2,3,3], seed=5)
+ draw_style_mixing_figure(os.path.join(config.result_dir, 'figure03-style-mixing.png'), load_Gs(url_ffhq), w=512, h=512, src_seeds=[639,701,687,615,2268], dst_seeds=[888,829,1898,1733,1614,845], style_ranges=[range(0,4)]*3+[range(4,8)]*2+[range(8,16)])
+ draw_noise_detail_figure(os.path.join(config.result_dir, 'figure04-noise-detail.png'), load_Gs(url_ffhq), w=512, h=512, num_samples=100, seeds=[1157,1012])
+ draw_noise_components_figure(os.path.join(config.result_dir, 'figure05-noise-components.png'), load_Gs(url_ffhq), w=512, h=512, seeds=[1967,1555], noise_ranges=[range(0, 18), range(0, 0), range(8, 18), range(0, 8)], flips=[1])
+ draw_truncation_trick_figure(os.path.join(config.result_dir, 'figure08-truncation-trick.png'), load_Gs(url_ffhq), w=512, h=512, seeds=[91,388, 389, 390, 391, 392, 393, 394, 395, 396], psis=[1, 0.7, 0.5, 0.25, 0, -0.25, -0.5, -1])
All this done, we get some fun anime face samples to parallel et al 2018’s figures:
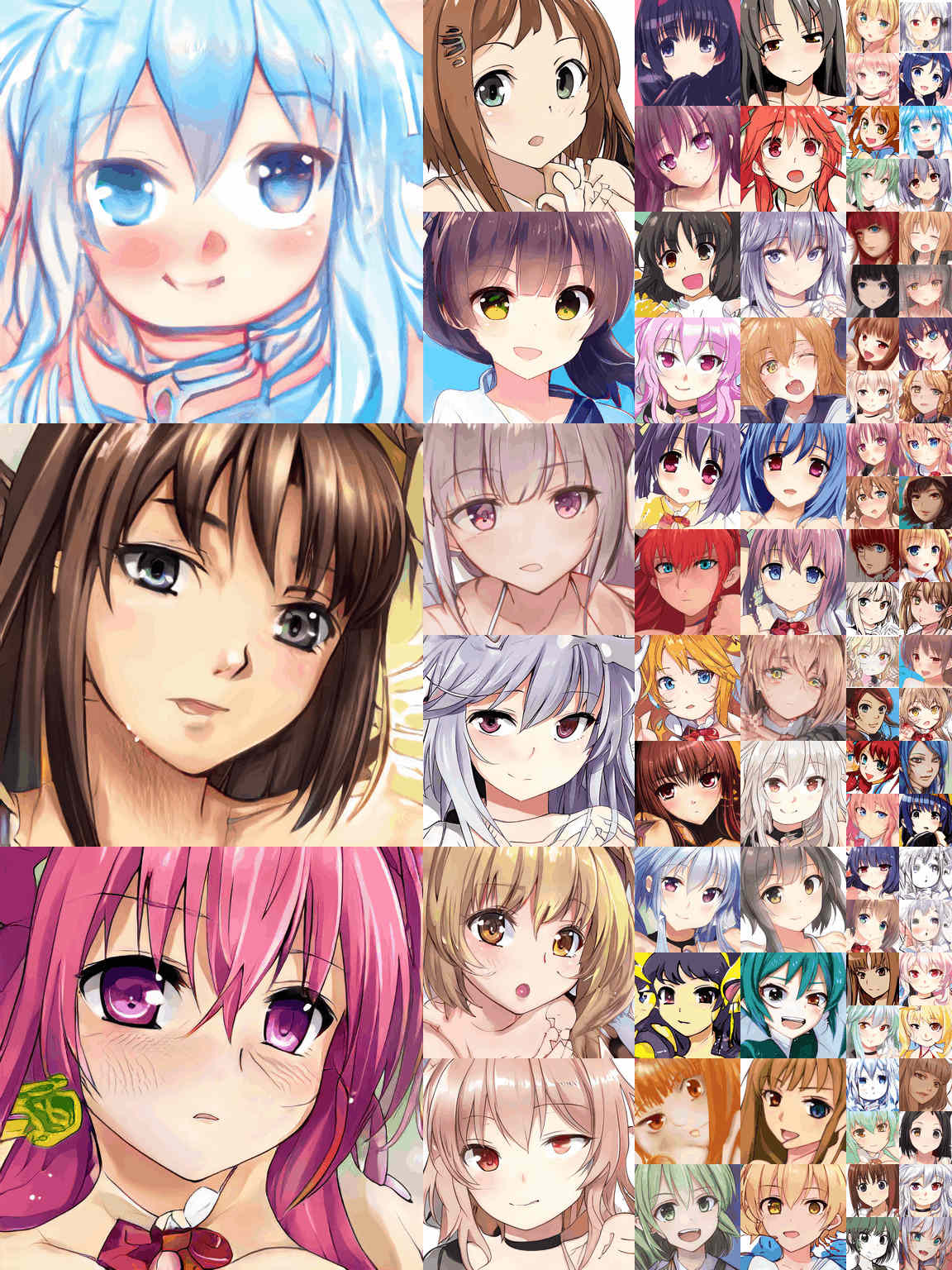
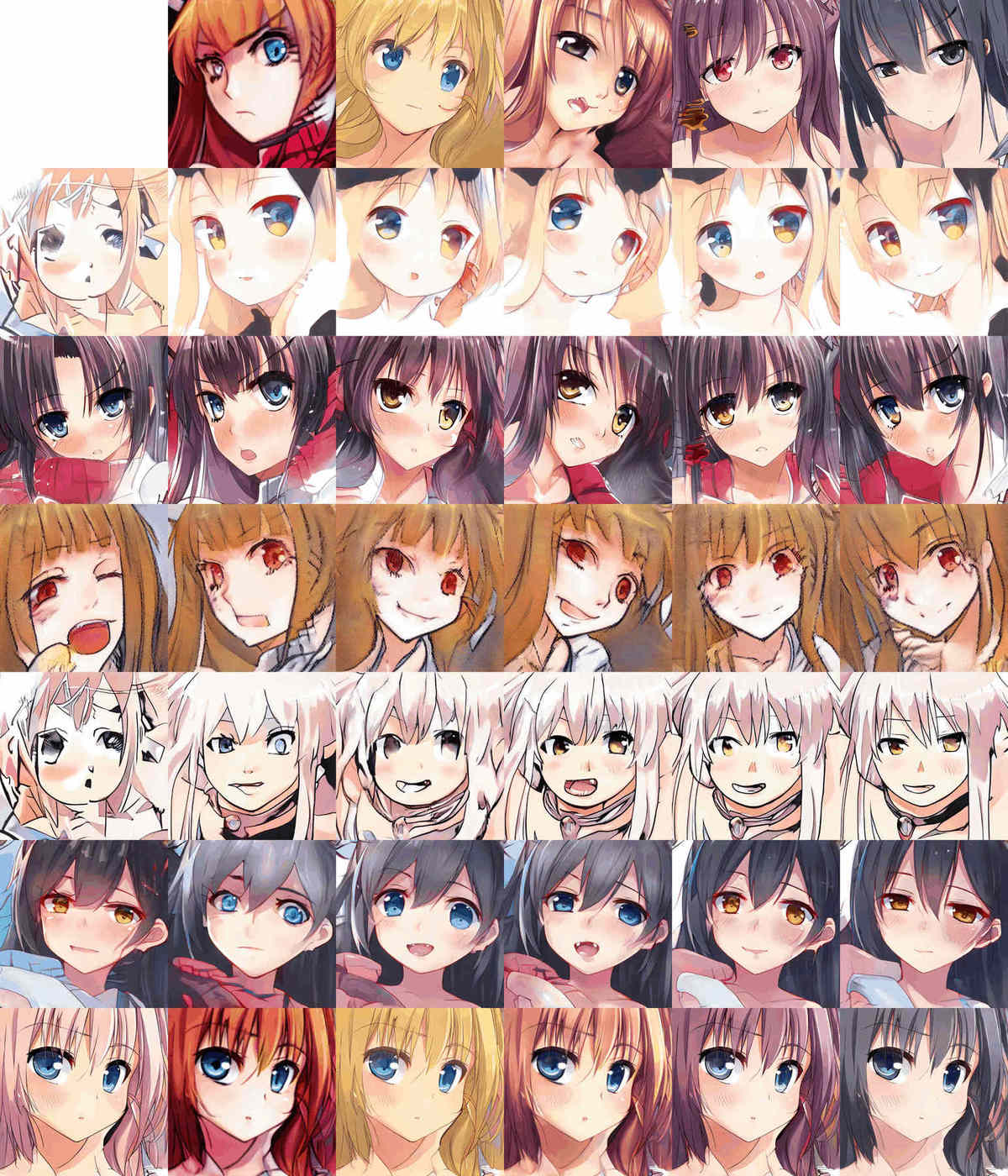
![Figure 8, the “truncation trick” visualized: 10 random faces, with the range Ψ = [1, 0.7, 0.5, 0.25, 0, −0.25, −0.5, −1]—demonstrating the tradeoff between diversity & quality, and the global average face.](/doc/ai/nn/gan/stylegan/anime/2019-03-17-gwern-stylegan-animeface-figure08-truncationtrick.jpg)
Videos
Training Montage
The easiest samples are the progress snapshots generated during training. Over the course of training, their size increases as the effective resolution increases & finer details are generated, and at the end can be quite large (often 14MB each for the anime faces) so doing lossy compression with a tool like pngnq
+advpng
or converting them to JPG with lowered quality is a good idea. To turn the many snapshots into a training montage video like above, I use FFmpeg on the PNGs:
cat $(ls ./results/*faces*/fakes*.png | sort --numeric-sort) | ffmpeg -framerate 10 \ # show 10 inputs per second
-i - # stdin
-r 25 # output frame-rate; frames will be duplicated to pad out to 25FPS
-c:v libx264 # x264 for compatibility
-pix_fmt yuv420p # force ffmpeg to use a standard colorspace - otherwise PNG colorspace is kept, breaking browsers (!)
-crf 33 # adequate high quality
-vf "scale=iw/2:ih/2" \ # shrink the image by 2×, the full detail is not necessary & saves space
-preset veryslow -tune animation \ # aim for smallest binary possible with animation-tuned settings
./stylegan-facestraining.mp4
Interpolations
The original ProGAN repo provided a config for generating interpolation videos, but that was removed in StyleGAN. Cyril Diagne (@kikko_fr
) implemented a replacement, providing 3 kinds of videos:
-
random_grid_404.mp4
: a standard interpolation video, which is simply a random walk through the latent space, modifying all the variables smoothly and animating it; by default it makes 4 of them arranged 2×2 in the video. Several interpolation videos are show in the examples section. -
interpolate.mp4
: a ‘coarse’ “style mixing” video; a single ‘source’ face is generated & held constant; a secondary interpolation video, a random walk as before is generated; at each step of the random walk, the ‘coarse’/high-level ‘style’ noise is copied from the random walk to overwrite the source face’s original style noise. For faces, this means that the original face will be modified with all sorts of orientations & facial expressions while still remaining recognizably the original character. (It is the video analog of et al 2018’s Figure 3.)A copy of Diagne’s
video.py
:import os import pickle import numpy as np import PIL.Image import dnnlib import dnnlib.tflib as tflib import config import scipy def main(): tflib.init_tf() # Load pre-trained network. # url = 'https://drive.google.com/uc?id=1MEGjdvVpUsu1jB4zrXZN7Y4kBBOzizDQ' # with dnnlib.util.open_url(url, cache_dir=config.cache_dir) as f: ## NOTE: insert model here: _G, _D, Gs = pickle.load(open("results/02047-sgan-faces-2gpu/network-snapshot-013221.pkl", "rb")) # _G = Instantaneous snapshot of the generator. Mainly useful for resuming a previous training run. # _D = Instantaneous snapshot of the discriminator. Mainly useful for resuming a previous training run. # Gs = Long-term average of the generator. Yields higher-quality results than the instantaneous snapshot. grid_size = [2,2] image_shrink = 1 image_zoom = 1 duration_sec = 60.0 smoothing_sec = 1.0 mp4_fps = 20 mp4_codec = 'libx264' mp4_bitrate = '5M' random_seed = 404 mp4_file = 'results/random_grid_%s.mp4' % random_seed minibatch_size = 8 num_frames = int(np.rint(duration_sec * mp4_fps)) random_state = np.random.RandomState(random_seed) # Generate latent vectors shape = [num_frames, np.prod(grid_size)] + Gs.input_shape[1:] # [frame, image, channel, component] all_latents = random_state.randn(*shape).astype(np.float32) import scipy all_latents = scipy.ndimage.gaussian_filter(all_latents, [smoothing_sec * mp4_fps] + [0] * len(Gs.input_shape), mode='wrap') all_latents /= np.sqrt(np.mean(np.square(all_latents))) def create_image_grid(images, grid_size=None): assert images.ndim == 3 or images.ndim == 4 num, img_h, img_w, channels = images.shape if grid_size is not None: grid_w, grid_h = tuple(grid_size) else: grid_w = max(int(np.ceil(np.sqrt(num))), 1) grid_h = max((num - 1) // grid_w + 1, 1) grid = np.zeros([grid_h * img_h, grid_w * img_w, channels], dtype=images.dtype) for idx in range(num): x = (idx % grid_w) * img_w y = (idx // grid_w) * img_h grid[y : y + img_h, x : x + img_w] = images[idx] return grid # Frame generation func for moviepy. def make_frame(t): frame_idx = int(np.clip(np.round(t * mp4_fps), 0, num_frames - 1)) latents = all_latents[frame_idx] fmt = dict(func=tflib.convert_images_to_uint8, nchw_to_nhwc=True) images = Gs.run(latents, None, truncation_psi=0.7, randomize_noise=False, output_transform=fmt) grid = create_image_grid(images, grid_size) if image_zoom > 1: grid = scipy.ndimage.zoom(grid, [image_zoom, image_zoom, 1], order=0) if grid.shape[2] == 1: grid = grid.repeat(3, 2) # grayscale => RGB return grid # Generate video. import moviepy.editor video_clip = moviepy.editor.VideoClip(make_frame, duration=duration_sec) video_clip.write_videofile(mp4_file, fps=mp4_fps, codec=mp4_codec, bitrate=mp4_bitrate) # import scipy # coarse duration_sec = 60.0 smoothing_sec = 1.0 mp4_fps = 20 num_frames = int(np.rint(duration_sec * mp4_fps)) random_seed = 500 random_state = np.random.RandomState(random_seed) w = 512 h = 512 #src_seeds = [601] dst_seeds = [700] style_ranges = ([0] * 7 + [range(8,16)]) * len(dst_seeds) fmt = dict(func=tflib.convert_images_to_uint8, nchw_to_nhwc=True) synthesis_kwargs = dict(output_transform=fmt, truncation_psi=0.7, minibatch_size=8) shape = [num_frames] + Gs.input_shape[1:] # [frame, image, channel, component] src_latents = random_state.randn(*shape).astype(np.float32) src_latents = scipy.ndimage.gaussian_filter(src_latents, smoothing_sec * mp4_fps, mode='wrap') src_latents /= np.sqrt(np.mean(np.square(src_latents))) dst_latents = np.stack(np.random.RandomState(seed).randn(Gs.input_shape[1]) for seed in dst_seeds) src_dlatents = Gs.components.mapping.run(src_latents, None) # [seed, layer, component] dst_dlatents = Gs.components.mapping.run(dst_latents, None) # [seed, layer, component] src_images = Gs.components.synthesis.run(src_dlatents, randomize_noise=False, **synthesis_kwargs) dst_images = Gs.components.synthesis.run(dst_dlatents, randomize_noise=False, **synthesis_kwargs) canvas = PIL.Image.new('RGB', (w * (len(dst_seeds) + 1), h * 2), 'white') for col, dst_image in enumerate(list(dst_images)): canvas.paste(PIL.Image.fromarray(dst_image, 'RGB'), ((col + 1) * h, 0)) def make_frame(t): frame_idx = int(np.clip(np.round(t * mp4_fps), 0, num_frames - 1)) src_image = src_images[frame_idx] canvas.paste(PIL.Image.fromarray(src_image, 'RGB'), (0, h)) for col, dst_image in enumerate(list(dst_images)): col_dlatents = np.stack([dst_dlatents[col]]) col_dlatents[:, style_ranges[col]] = src_dlatents[frame_idx, style_ranges[col]] col_images = Gs.components.synthesis.run(col_dlatents, randomize_noise=False, **synthesis_kwargs) for row, image in enumerate(list(col_images)): canvas.paste(PIL.Image.fromarray(image, 'RGB'), ((col + 1) * h, (row + 1) * w)) return np.array(canvas) # Generate video. import moviepy.editor mp4_file = 'results/interpolate.mp4' mp4_codec = 'libx264' mp4_bitrate = '5M' video_clip = moviepy.editor.VideoClip(make_frame, duration=duration_sec) video_clip.write_videofile(mp4_file, fps=mp4_fps, codec=mp4_codec, bitrate=mp4_bitrate) import scipy duration_sec = 60.0 smoothing_sec = 1.0 mp4_fps = 20 num_frames = int(np.rint(duration_sec * mp4_fps)) random_seed = 503 random_state = np.random.RandomState(random_seed) w = 512 h = 512 style_ranges = [range(6,16)] fmt = dict(func=tflib.convert_images_to_uint8, nchw_to_nhwc=True) synthesis_kwargs = dict(output_transform=fmt, truncation_psi=0.7, minibatch_size=8) shape = [num_frames] + Gs.input_shape[1:] # [frame, image, channel, component] src_latents = random_state.randn(*shape).astype(np.float32) src_latents = scipy.ndimage.gaussian_filter(src_latents, smoothing_sec * mp4_fps, mode='wrap') src_latents /= np.sqrt(np.mean(np.square(src_latents))) dst_latents = np.stack([random_state.randn(Gs.input_shape[1])]) src_dlatents = Gs.components.mapping.run(src_latents, None) # [seed, layer, component] dst_dlatents = Gs.components.mapping.run(dst_latents, None) # [seed, layer, component] def make_frame(t): frame_idx = int(np.clip(np.round(t * mp4_fps), 0, num_frames - 1)) col_dlatents = np.stack([dst_dlatents[0]]) col_dlatents[:, style_ranges[0]] = src_dlatents[frame_idx, style_ranges[0]] col_images = Gs.components.synthesis.run(col_dlatents, randomize_noise=False, **synthesis_kwargs) return col_images[0] # Generate video. import moviepy.editor mp4_file = 'results/fine_%s.mp4' % (random_seed) mp4_codec = 'libx264' mp4_bitrate = '5M' video_clip = moviepy.editor.VideoClip(make_frame, duration=duration_sec) video_clip.write_videofile(mp4_file, fps=mp4_fps, codec=mp4_codec, bitrate=mp4_bitrate) if __name__ == "__main__": main()
-
fine_503.mp4
: a ‘fine’ style mixing video; in this case, the style noise is taken from later on and instead of affecting the global orientation or expression, it affects subtler details like the precise shape of hair strands or hair color or mouths.
Circular interpolations are another interesting kind of interpolation, written by snowy halcy, which instead of random walking around the latent space freely, with large or awkward transitions, instead tries to move around a fixed high-dimensional point doing: “binary search to get the MSE to be roughly the same between frames (slightly brute force, but it looks nicer), and then did that for what is probably close to a sphere or circle in the latent space.” A later version of circular interpolation is in snowy halcy’s face editor repo, but here is the original version cleaned up into a stand-alone program:
import dnnlib.tflib as tflib
import math
import moviepy.editor
from numpy import linalg
import numpy as np
import pickle
def main():
tflib.init_tf()
_G, _D, Gs = pickle.load(open("results/02051-sgan-faces-2gpu/network-snapshot-021980.pkl", "rb"))
rnd = np.random
latents_a = rnd.randn(1, Gs.input_shape[1])
latents_b = rnd.randn(1, Gs.input_shape[1])
latents_c = rnd.randn(1, Gs.input_shape[1])
def circ_generator(latents_interpolate):
radius = 40.0
latents_axis_x = (latents_a - latents_b).flatten() / linalg.norm(latents_a - latents_b)
latents_axis_y = (latents_a - latents_c).flatten() / linalg.norm(latents_a - latents_c)
latents_x = math.sin(math.pi * 2.0 * latents_interpolate) * radius
latents_y = math.cos(math.pi * 2.0 * latents_interpolate) * radius
latents = latents_a + latents_x * latents_axis_x + latents_y * latents_axis_y
return latents
def mse(x, y):
return (np.square(x - y)).mean()
def generate_from_generator_adaptive(gen_func):
max_step = 1.0
current_pos = 0.0
change_min = 10.0
change_max = 11.0
fmt = dict(func=tflib.convert_images_to_uint8, nchw_to_nhwc=True)
current_latent = gen_func(current_pos)
current_image = Gs.run(current_latent, None, truncation_psi=0.7, randomize_noise=False, output_transform=fmt)[0]
array_list = []
video_length = 1.0
while(current_pos < video_length):
array_list.append(current_image)
lower = current_pos
upper = current_pos + max_step
current_pos = (upper + lower) / 2.0
current_latent = gen_func(current_pos)
current_image = images = Gs.run(current_latent, None, truncation_psi=0.7, randomize_noise=False, output_transform=fmt)[0]
current_mse = mse(array_list[-1], current_image)
while current_mse < change_min or current_mse > change_max:
if current_mse < change_min:
lower = current_pos
current_pos = (upper + lower) / 2.0
if current_mse > change_max:
upper = current_pos
current_pos = (upper + lower) / 2.0
current_latent = gen_func(current_pos)
current_image = images = Gs.run(current_latent, None, truncation_psi=0.7, randomize_noise=False, output_transform=fmt)[0]
current_mse = mse(array_list[-1], current_image)
print(current_pos, current_mse)
return array_list
frames = generate_from_generator_adaptive(circ_generator)
frames = moviepy.editor.ImageSequenceClip(frames, fps=30)
# Generate video.
mp4_file = 'results/circular.mp4'
mp4_codec = 'libx264'
mp4_bitrate = '3M'
mp4_fps = 20
frames.write_videofile(mp4_file, fps=mp4_fps, codec=mp4_codec, bitrate=mp4_bitrate)
if __name__ == "__main__":
main()
An interesting use of interpolations is Kyle McLean’s “Waifu Synthesis” video: a singing anime video mashing up StyleGAN anime faces + GPT-2 lyrics + Project Magenta music.
Models
Anime Faces
The primary model I’ve trained, the anime face model is described in the data processing & training section. It is a 512px StyleGAN model trained on n = 218,794 faces cropped from all of Danbooru2017, cleaned, & upscaled, and trained for 21,980 iterations or ~21m images or ~38 GPU-days.
Downloads (I recommend using the more-recent portrait StyleGAN unless cropped faces are specifically desired):
-
random samples generated on 2091-02-14 with an extreme Ψ = 1.2 (165MB, JPG)
-
the StyleGAN model used for TWDNEv1 samples as of 2019-02-26 (294MB,
.pkl
)-
all TWDNE faces via rsync
-
-
the anime face StyleGAN model, further trained, as of 2019-03-8
The anime face model is obsoleted by the StyleGAN 2 portrait model.
TWDNE
To show off the anime faces, and as a joke, on 2019-02-14, I set up “This Waifu Does Not Exist”, a standalone static website which displays a random anime face (out of 100,000), generated with various Ψ, and paired with GPT-2-117M text snippets prompted on anime plot summaries. The details of the site implementation & generating the faces/text are too length to go into here
But the site was amusing & an enormous success. It went viral overnight and by the end of March 2019, ~1 million unique visitors (most from China) had visited TWDNE, spending over 2 minutes each looking at the NN-generated faces & text; people began hunting for hilariously-deformed faces, using TWDNE as a screensaver, picking out faces as avatars, creating packs of faces for video games, painting their own collages of faces, using it as a character designer for inspiration, etc.
Anime Bodies
Aaron Gokaslan experimented with a custom 256px anime game image dataset which has individual characters posed in whole-person images to see how StyleGAN coped with more complex geometries. Progress required additional data cleaning and lowering the learning rate but, trained on a 4-GPU system for week or two, the results are promising (even down to reproducing the copyright statements in the images), providing preliminary evidence that StyleGAN can scale:
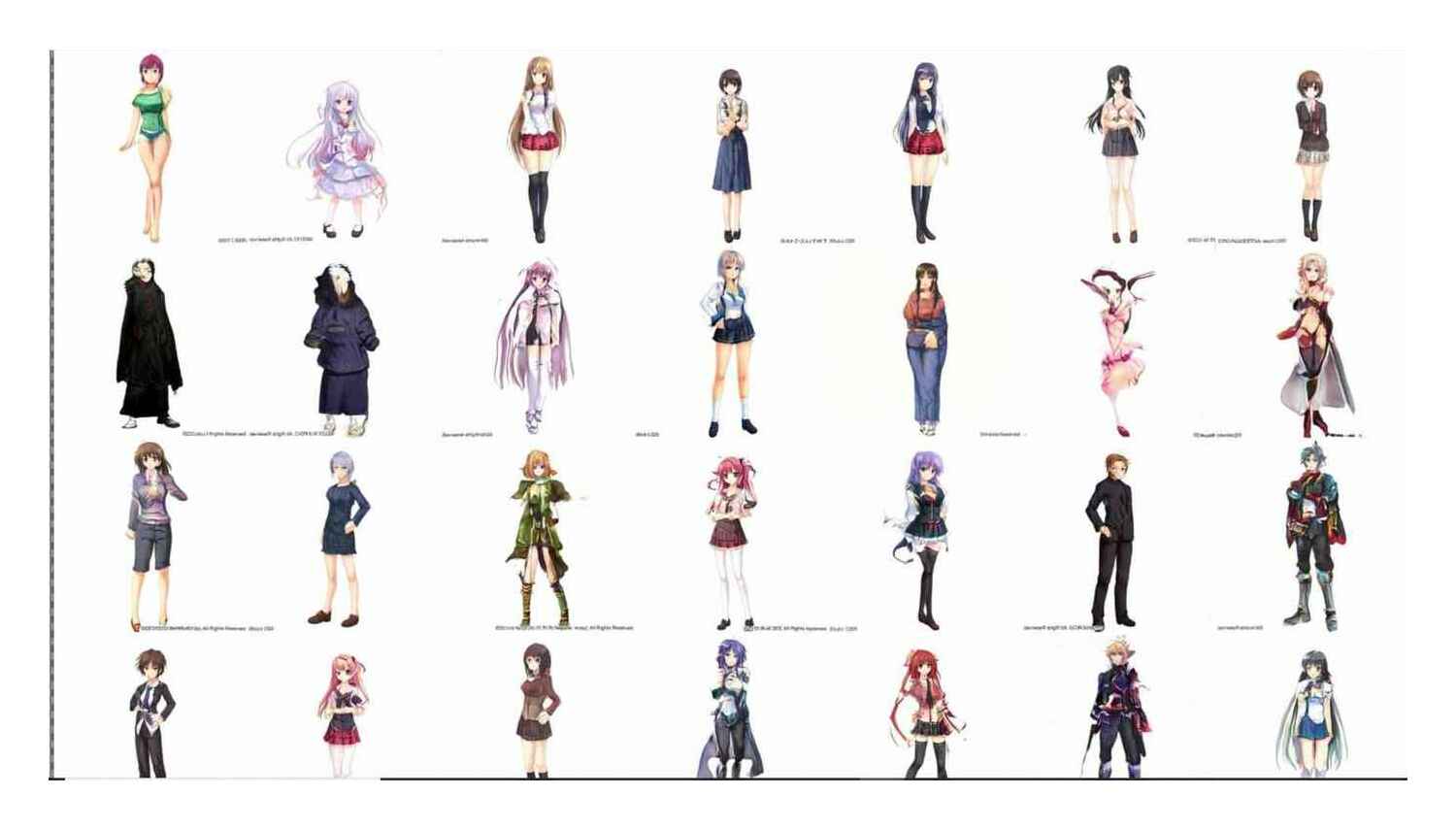
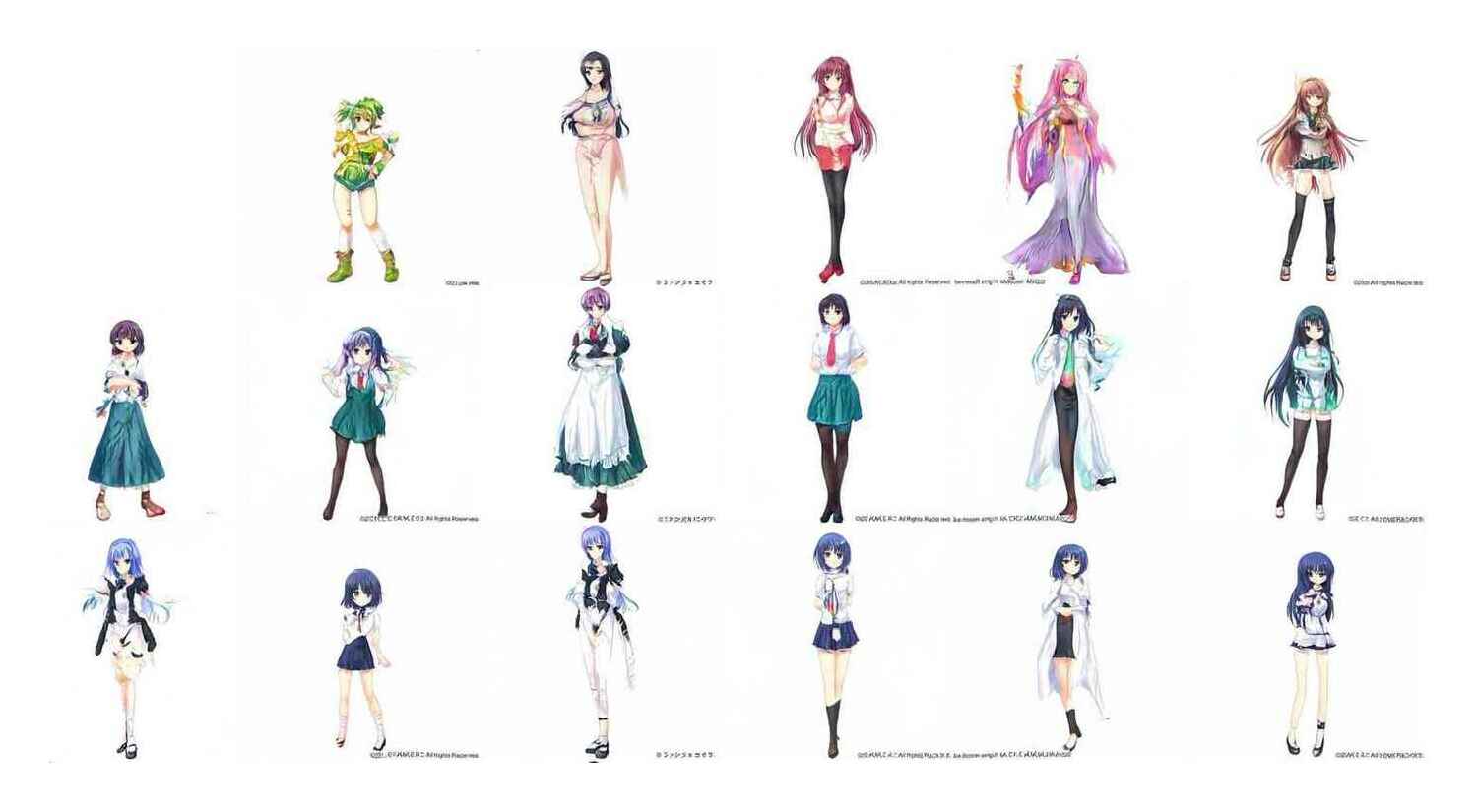
Conditional Anime Faces, Arfafax
In March 2020, Arfafax trained a conditional anime face StyleGAN: it takes a list of tags (a subset of Danbooru2019 tags relevant to faces), processes them via doc2vec into a fixed-size input, and feeds them into a conditional StyleGAN.32 (While almost all uses of StyleGAN are unconditional—you just dump in a big pile of images and it learns to generate random samples—the code actually supports conditional use where a category or set of variables is turned into an output image, and a few other people have experimented with it.)
![Sample generated for the tags: ['0:white_hair', '0:blue_eyes', '0:long_hair']](/doc/ai/nn/gan/stylegan/anime/arfafax-conditionalstylegan-animefaces-blueeyeswhitehair.png)
![Tags: ['0:blonde_hair', '0:blue_eyes', '0:long_hair']](/doc/ai/nn/gan/stylegan/anime/arfafax-conditionalstylegan-animefaces-blueeyesblondehair.png)
![Tags: ['4:hirasawa_yui']](/doc/ai/nn/gan/stylegan/anime/arfafax-conditionalstylegan-animefaces-hirasawayui.png)
Conditional GAN Problems
In theory, a conditional anime face GAN would have two major benefits over the regular kind: because additional information is supplied by the human-written tags describing each datapoint, the model should be able to learn higher-quality faces; and because the faces are generated based on a specific description, one can directly control the output without any complex encoding/editing tricks. The final model was easier to control, but the quality was only OK.
What went wrong? In practice, Arfafax ran into challenges with overfitting, quality, and the model seeming to ignore many tags (and focusing instead on a few dimensions like hair color); my suspicion is that he rediscovered the same conditional-GAN issues that the StackGAN authors encountered, where the tag embedding is so high-dimensional that each face is effectively unique (unlike categorical conditioning where there will be hundreds or thousands of other images with the same category label), leading to Discriminator memorization & training collapse. (StackGAN’s remedy was “conditioning augmentation” : regularizing D’s use of the embedding by adding random Gaussian noise to each use of an embedding, and this is a trick which has surfaced repeatedly in conditional GANs since, such as textStyleGAN so it seems no one has a better idea how to fix it. The data augmentation GANs appear to do this sort of regularization implicitly by modifying the images instead, and sometimes by adding a ‘consistency loss’ on z, which requires noising it. Also of interest is CLIP’s observation that training by predicting the exact text of a caption from the image didn’t work well, and it was much better to instead do contrastive learning within a minibatch: in effect, instead of trying to predict the caption text for a image, trying to predict which of the images inside the minibatch is most likely to match which of the caption texts compared to the others.)
To test this theory, Arfafax ran an additional StyleGAN experiment (Colab notebook), using the “Cartoon Set” 100K dataset: a collection of simple emoji-like cartoon faces which were defined using 18 (10 vs 4 vs 4 categories = 1013 unique faces) variables & a large subset generated. Because the dataset is synthetic, the variables can be encoded both as categorical variables and as doc2vec embeddings, the set of variables should uniquely specify each image, and it is easy to determine the amount of mode-dropping by looking. Arfafax compared the standard unconditional StyleGAN, a multi-categorical embedding StyleGAN, and a doc2vec StyleGAN. The unconditional did fine as usual, the categorical one did somewhat poorly, and the doc2vec one collapsed badly—failing to generate entire swaths of Cartoon Set face-space. So the problem does appear to be the embedding.
Tag → Face Usage
Arfafax has provided a Google Colab notebook (code) for generating anime faces from tag inputs and for generating interpolations.
Complete supported tag list (excluding individual characters, which are tagged like miyu_(vampire_princess_miyu)
or morrigan_aensland
):
>>> face_tags
['angry', 'anger_vein', 'annoyed', 'clenched_teeth', 'blush', 'blush_stickers', 'embarrassed', 'bored',
'closed_eyes', 'confused', 'crazy', 'disdain', 'disgust', 'drunk', 'envy', 'expressionless', 'evil', 'facepalm',
'flustered', 'frustrated', 'grimace', 'guilt', 'happy', 'kubrick_stare', 'lonely', 'nervous', 'nosebleed',
'one_eye_closed', 'open_mouth', 'closed_mouth', 'parted_lips', 'pain', 'pout', 'raised_eyebrow', 'rape_face',
'rolling_eyes', 'sad', 'depressed', 'frown', 'gloom_(expression)', 'tears', 'horns', 'scared', 'panicking',
'worried', 'serious', 'sigh', 'sleepy', 'tired', 'sulking', 'thinking', 'pensive', 'wince', 'afterglow',
'ahegao', 'fucked_silly', 'naughty_face', 'torogao', 'smile', 'crazy_smile', 'evil_smile', 'fingersmile',
'forced_smile', 'glasgow_smile', 'grin', 'fang', 'evil_grin', 'light_smile', 'sad_smile', 'seductive_smile',
'stifled_laugh', 'smug', 'doyagao', 'smirk', 'smug', 'troll_face', 'surprised', 'scared', '/\\/\\/\\',
'color_drain', 'horror_(expression)', 'screaming', 'turn_pale', 'trembling', 'wavy_mouth', ';)', ':d',
';d', 'xd', 'd:', ':}', ':{', ':3', ';3', 'x3', '3:', 'uwu', '=.w.=', ':p', ';p', ':q', ';q', '>:)', '>:(',
':t', ':i', ':/', ':x', ':c', 'c:', ':<', ';<', ':<>', ':>', ':>=', ';>=', ':o', ';o', '=', '=)', '=d',
'=o', '=v', '|3', '|d', '|o', 'o3o', '(-3-)', '>3<', 'o_o', '0_0', '._.', '•_•', 'solid_circle_eyes',
'♥_♥', 'heart_eyes', '^_^', '^o^', '\\(^o^)/', '└(^o^)┐≡', '^q^', '>_<', 'xd', 'x3', '>o<', '<_>', ';_;',
'@_@', '>_@', '+_+', '+_-', '-_-', '\\_/', '=_=', '=^=', '=v=', '<o>_<o>', 'constricted_pupils', 'cross_eyed',
'rectangular_mouth', 'sideways_mouth', 'no_nose', 'no_mouth', 'wavy_mouth', 'wide-eyed', 'mouth_drool',
'awesome_face', 'foodgasm', 'henohenomoheji', 'nonowa', 'portrait', 'profile', 'smiley_face', 'uso_da',
'food_awe', 'breast_awe', 'penis_awe']
>>> eye_tags
['aqua_eyes', 'black_eyes', 'blue_eyes', 'brown_eyes', 'green_eyes', 'grey_eyes', 'orange_eyes', 'lavender_eyes',
'pink_eyes', 'purple_eyes', 'red_eyes', 'silver_eyes', 'white_eyes', 'yellow_eyes', 'heterochromia', 'multicolored_eyes',
'al_bhed_eyes', 'pac-man_eyes', 'ringed_eyes', 'constricted_pupils', 'dilated_pupils', 'horizontal_pupils',
'no_pupils', 'slit_pupils', 'symbol-shaped_pupils', '+_+', 'heart-shaped_pupils', 'star-shaped_pupils',
'blue_sclera', 'black_sclera', 'blank_eyes', 'bloodshot_eyes', 'green_sclera', 'mismatched_sclera', 'orange_sclera',
'red_sclera', 'yellow_sclera', 'bags_under_eyes', 'bruised_eye', 'flaming_eyes', 'glowing_eyes', 'glowing_eye',
'mako_eyes', 'amphibian_eyes', 'button_eyes', 'cephalopod_eyes', 'compound_eyes', 'frog_eyes', 'crazy_eyes',
'empty_eyes', 'heart_eyes', 'nonowa', 'solid_circle_eyes', 'o_o', '0_0', 'jitome', 'tareme', 'tsurime',
'sanpaku', 'sharingan', 'mangekyou_sharingan', 'eye_reflection', 'text_in_eyes', 'missing_eye', 'one-eyed',
'third_eye', 'extra_eyes', 'no_eyes']
>>> eye_expressions
['>_<', 'x3', 'xd', 'o_o', '0_0', '3_3', '6_9', '@_@', '^_^', '^o^', '9848', '26237', '=_=', '+_+', '._.',
'<o>_<o>', 'blinking', 'closed_eyes', 'wince', 'one_eye_closed', ';<', ';>', ';p']
>>> eye_other
['covering_eyes', 'hair_over_eyes', 'hair_over_one_eye', 'bandage_over_one_eye', 'blindfold', 'hat_over_eyes',
'eyepatch', 'eyelashes', 'colored_eyelashes', 'fake_eyelashes', 'eyes_visible_through_hair', 'glasses',
'makeup', 'eyeliner', 'eyeshadow', 'mascara', 'eye_contact', 'looking_afar', 'looking_at_another', 'looking_at_breasts',
'looking_at_hand', 'looking_at_mirror', 'looking_at_phone', 'looking_at_viewer', 'looking_away', 'looking_back',
'looking_down', 'looking_out_window', 'looking_over_glasses', 'looking_through_legs', 'looking_to_the_side',
'looking_up', 'akanbe', 'blind', 'cross-eyed', 'drawn_on_eyes', 'eyeball', 'eye_beam', 'eye_poke', 'eye_pop',
'persona_eyes', 'shading_eyes', 'squinting', 'staring', 'uneven_eyes', 'upturned_eyes', 'wall-eyed', 'wide-eyed', 'wince']
>>> ears_tags
['animal_ears', 'bear_ears', 'bunny_ears', 'cat_ears', 'dog_ears', 'fake_animal_ears', 'fox_ears', 'horse_ears',
'kemonomimi_mode', 'lion_ears', 'monkey_ears', 'mouse_ears', 'raccoon_ears', 'sheep_ears', 'tiger_ears',
'wolf_ears', 'pointy_ears', 'robot_ears', 'extra_ears', 'ear_piercing', 'ear_protection', 'earrings',
'single_earring', 'headphones', 'covering_ears', 'ear_biting', 'ear_licking', 'ear_grab']
>>> hair_tags
['heartbreak_haircut', 'hand_in_hair', 'adjusting_hair', 'bunching_hair', 'hair_flip', 'hair_grab', 'hair_pull',
'hair_tucking', 'hair_tousle', 'hair_twirling', 'hair_sex', 'hair_brush', 'hair_dryer', 'shampoo', 'bun_cover',
'hairpods', 'chopsticks', 'comb', 'hair_ornament', 'hair_bell', 'hair_bobbles', 'hair_bow', 'hair_ribbon',
'hairclip', 'hairpin', 'hair_flower', 'hair_tubes', 'kanzashi', 'hair_tie', 'hairband', 'hair_weapon',
'headband', 'scrunchie', 'wig', 'facial_hair', 'beard', 'bearded_girl', 'goatee', 'mustache', 'fake_mustache',
'stubble', 'fiery_hair', 'prehensile_hair', 'helicopter_hair', 'tentacle_hair', 'living_hair', 'detached_hair',
'severed_hair', 'floating_hair', 'hair_spread_out', 'wet_hair']
>>> hair_color_tags
['aqua_hair', 'black_hair', 'blonde_hair', 'blue_hair', 'light_blue_hair', 'brown_hair', 'light_brown_hair',
'green_hair', 'grey_hair', 'magenta_hair', 'orange_hair', 'pink_hair', 'purple_hair', 'lavender_hair',
'red_hair', 'auburn_hair', 'maroon_hair', 'silver_hair', 'white_hair', 'multicolored_hair', 'colored_inner_hair',
'gradient_hair', 'rainbow_hair', 'streaked_hair', 'two-tone_hair', 'highlights', 'colored_tips', 'alternate_hair_color']
>>> hair_style_tags
['very_short_hair', 'short_hair', 'medium_hair', 'long_hair', 'very_long_hair', 'absurdly_long_hair',
'big_hair', 'bald', 'bald_girl', 'alternate_hairstyle', 'hair_down', 'hair_up', 'curly_hair', 'drill_hair',
'twin_drills', 'flipped_hair', 'hair_flaps', 'messy_hair', 'pointy_hair', 'ringlets', 'spiked_hair', 'wavy_hair',
'bangs', 'asymmetrical_bangs', 'blunt_bangs', 'hair_over_eyes', 'hair_over_one_eye', 'parted_bangs', 'swept_bangs',
'hair_between_eyes', 'hair_intakes', 'sidelocks', "widow's_peak", 'ahoge', 'heart_ahoge', 'huge_ahoge',
'antenna_hair', 'comb_over', 'hair_pulled_back', 'hair_slicked_back', 'mohawk', 'hair_bikini', 'hair_censor',
'hair_in_mouth', 'hair_over_breasts', 'hair_over_one_breast', 'hair_over_crotch', 'hair_over_shoulder',
'hair_scarf', 'bow_by_hair', 'braid', 'braided_bangs', 'front_braid', 'side_braid', 'french_braid', 'crown_braid',
'single_braid', 'multiple_braids', 'twin_braids', 'tri_braids', 'quad_braids', 'hair_bun', 'braided_bun',
'double_bun', 'triple_bun', 'hair_rings', 'half_updo', 'one_side_up', 'two_side_up', 'low-braided_long_hair',
'low-tied_long_hair', 'mizura', 'multi-tied_hair', 'nihongami', 'ponytail', 'folded_ponytail', 'front_ponytail',
'high_ponytail', 'short_ponytail', 'side_ponytail', 'split_ponytail', 'topknot', 'twintails', 'low_twintails',
'short_twintails', 'uneven_twintails', 'tri_tails', 'quad_tails', 'quin_tails', 'bob_cut', 'bowl_cut',
'buzz_cut', 'chonmage', 'crew_cut', 'flattop', 'pixie_cut', 'undercut', 'cornrows', 'hairlocs', 'hime_cut',
'mullet', 'afro', 'huge_afro', 'beehive_hairdo', 'pompadour', 'quiff', 'shouten_pegasus_mix_mori']
>>> skin_color_tags
['dark_skin', 'pale_skin', 'tan', 'tanlines', 'sun_tattoo', 'black_skin', 'blue_skin', 'green_skin', 'grey_skin',
'orange_skin', 'pink_skin', 'purple_skin', 'red_skin', 'white_skin', 'yellow_skin', 'shiny_skin']
>>> headwear_tags
['crown', 'hat', 'helmet', 'black_headwear', 'blue_headwear', 'brown_headwear', 'green_headwear', 'grey_headwear',
'orange_headwear', 'pink_headwear', 'purple_headwear', 'red_headwear', 'white_headwear', 'yellow_headwear',
'ajirogasa', 'animal_hat', 'cat_hat', 'penguin_hat', 'baseball_cap', 'beanie', 'beret', 'bicorne', 'boater_hat',
'bowl_hat', 'bowler_hat', 'bucket_hat', 'cabbie_hat', 'chef_hat', 'toque_blanche', 'flat_top_chef_hat',
'cloche_hat', 'cowboy_hat', 'deerstalker', 'deviruchi_hat', 'dixie_cup_hat', 'eggshell_hat', 'fedora',
'female_service_cap', 'flat_cap', 'fur_hat', 'garrison_cap', 'jester_cap', 'kepi', 'mian_guan', 'mitre',
'mob_cap', 'mortarboard', 'nightcap', 'nurse_cap', 'party_hat', 'peaked_cap', 'pillow_hat', 'pirate_hat',
'porkpie_hat', 'pumpkin_hat', 'rice_hat', 'robe_and_wizard_hat', 'sailor_hat', 'santa_hat', 'mini_santa_hat',
'shako_cap', 'shampoo_hat', 'sombrero', 'sun_hat', "tam_o'_shanter", 'tate_eboshi', 'tokin_hat', 'top_hat',
'mini_top_hat', 'tricorne', 'ushanka', 'witch_hat', 'mini_witch_hat', 'wizard_hat', 'veil', 'zun_hat',
'baseball_helmet', 'bicycle_helmet', 'brodie_helmet', 'diving_helmet', 'football_helmet', 'hardhat', 'horned_helmet',
'helm', 'kabuto', 'motorcycle_helmet', 'pickelhaube', 'pith_helmet', 'stahlhelm', 'tank_helmet', 'winged_helmet',
'circlet', 'diadem', 'mini_crown', 'saishi', 'tiara', 'aviator_cap', 'bandana', 'bonnet', 'dalachi_(headdress)',
'habit', 'hijab', 'keffiyeh', 'shower_cap', 'visor_cap', 'checkered_hat', 'frilled_hat', 'military_hat',
'mini_hat', 'multicolored_hat', 'police_hat', 'print_hat', 'school_hat', 'straw_hat', 'adjusting_hat',
'hand_on_headwear', 'hands_on_headwear', 'hat_basket', 'hat_loss', 'hat_on_chest', 'hat_over_eyes', 'hat_over_one_eye',
'hat_removed', 'hat_tip', 'holding_hat', 'torn_hat', 'no_hat', 'hat_bow', 'hat_feather', 'hat_flower',
'hat_ribbon', 'hat_with_ears', 'adjusting_hat', 'backwards_hat', 'hat_removed', 'holding_hat', 'torn_hat',
'hair_bow', 'hair_ribbon', 'hairband', 'headband', 'forehead_protector', 'sweatband', 'hachimaki', 'nejiri_hachimaki',
'mongkhon', 'headdress', 'maid_headdress', 'veil', 'hood']
>>> eyewear_tags
['glasses', 'monocle', 'sunglasses', 'aqua-framed_eyewear', 'black-framed_eyewear', 'blue-framed_eyewear',
'brown-framed_eyewear', 'green-framed_eyewear', 'grey-framed_eyewear', 'orange-framed_eyewear', 'pink-framed_eyewear',
'purple-framed_eyewear', 'red-framed_eyewear', 'white-framed_eyewear', 'yellow-framed_eyewear', 'blue-tinted_eyewear',
'brown-tinted_eyewear', 'green-tinted_eyewear', 'orange-tinted_eyewear', 'pink-tinted_eyewear', 'purple-tinted_eyewear',
'red-tinted_eyewear', 'yellow-tinted_eyewear', 'heart-shaped_eyewear', 'round_eyewear', 'over-rim_eyewear',
'rimless_eyewear', 'semi-rimless_eyewear', 'under-rim_eyewear', 'adjusting_eyewear', 'eyewear_on_head',
'eyewear_removed', 'eyewear_hang', 'eyewear_in_mouth', 'holding_eyewear', 'eyewear_strap', 'eyewear_switch',
'looking_over_eyewear', 'no_eyewear', '3d_glasses', 'coke-bottle_glasses', 'diving_mask', 'fancy_glasses',
'heart-shaped_eyewear', 'funny_glasses', 'goggles', 'nodoka_glasses', 'opaque_glasses', 'pince-nez', 'safety_glasses',
'shooting_glasses', 'ski_goggles', 'x-ray_glasses', 'bespectacled', 'kamina_shades', 'star_shades']
>>> piercings_tags
['ear_piercing', 'eyebrow_piercing', 'anti-eyebrow_piercing', 'eyelid_piercing', 'lip_piercing', 'labret_piercing',
'nose_piercing', 'bridge_piercing', 'tongue_piercing']
>>> format_tags
['3d', 'animated', 'animated_png', 'flash', 'music_video', 'song', 'video', 'animated_gif', 'non-looping_animation',
'archived_file', 'artbook', 'bmp', 'calendar_(medium)', 'card_(medium)', 'comic', '2koma', '3koma', '4koma',
'multiple_4koma', '5koma', 'borderless_panels', 'doujinshi', 'eromanga', 'left-to-right_manga', 'right-to-left_comic',
'silent_comic', 'corrupted_image', 'cover', 'album_cover', 'character_single', 'cover_page', 'doujin_cover',
'dvd_cover', 'fake_cover', 'game_cover', 'magazine_cover', 'manga_cover', 'fake_screenshot', 'game_cg',
'gyotaku_(medium)', 'highres', 'absurdres', 'incredibly_absurdres', 'lowres', 'thumbnail', 'huge_filesize',
'icon', 'logo', 'kirigami', 'lineart', 'no_lineart', 'outline', 'long_image', 'tall_image', 'wide_image',
'mosaic_art', 'photomosaic', 'oekaki', 'official_art', 'phonecard', 'photo', 'papercraft', 'paper_child',
'paper_cutout', 'pixel_art', 'postcard', 'poster', 'revision', 'bad_revision', 'artifacted_revision',
'censored_revision', 'corrupted_revision', 'lossy_revision', 'watermarked_revision', 'scan', 'screencap',
'shitajiki', 'tegaki', 'transparent_background', 'triptych_(art)', 'vector_trace', 'wallpaper', 'dual_monitor',
'ios_wallpaper', 'official_wallpaper', 'phone_wallpaper', 'psp_wallpaper', 'tileable', 'wallpaper_forced', 'widescreen']
>>> style_tags
['abstract', 'art_deco', 'art_nouveau', 'fine_art_parody', 'flame_painter', 'impressionism', 'nihonga',
'sumi-e', 'ukiyo-e', 'minimalism', 'realistic', 'photorealistic', 'sketch', 'style_parody', 'list_of_style_parodies',
'surreal', 'traditional_media', 'faux_traditional_media', 'work_in_progress', 'backlighting', 'blending',
'bloom', 'bokeh', 'caustics', 'chiaroscuro', 'chromatic_aberration', 'chromatic_aberration_abuse', 'diffraction_spikes',
'depth_of_field', 'dithering', 'drop_shadow', 'emphasis_lines', 'foreshortening', 'gradient', 'halftone',
'lens_flare', 'lens_flare_abuse', 'motion_blur', 'motion_lines', 'multiple_monochrome', 'optical_illusion',
'anaglyph', 'exif_thumbnail_surprise', 'open_in_internet_explorer', 'open_in_winamp', 'stereogram', 'scanlines',
'silhouette', 'speed_lines', 'vignetting']
Download:
-
rsync --verbose --recursive rsync://176.9.41.242:873/biggan/2021-01-09-arfafax-stylegan-danbooruportraits-tagconditional.tar.xz ./
(all files: doc2vec, StyleGAN model, notebook, videos)
Extended StyleGAN2 Danbooru2019, Aydao
Aydao (Twitter), in parallel with the BigGAN experiments, worked on gradually extending StyleGAN2’s modeling powers to cover Danbooru2019 SFW. His results were excellent and power nearcyan’s (Twitter) This Anime Does Not Exist.ai (TADNE). Samples:
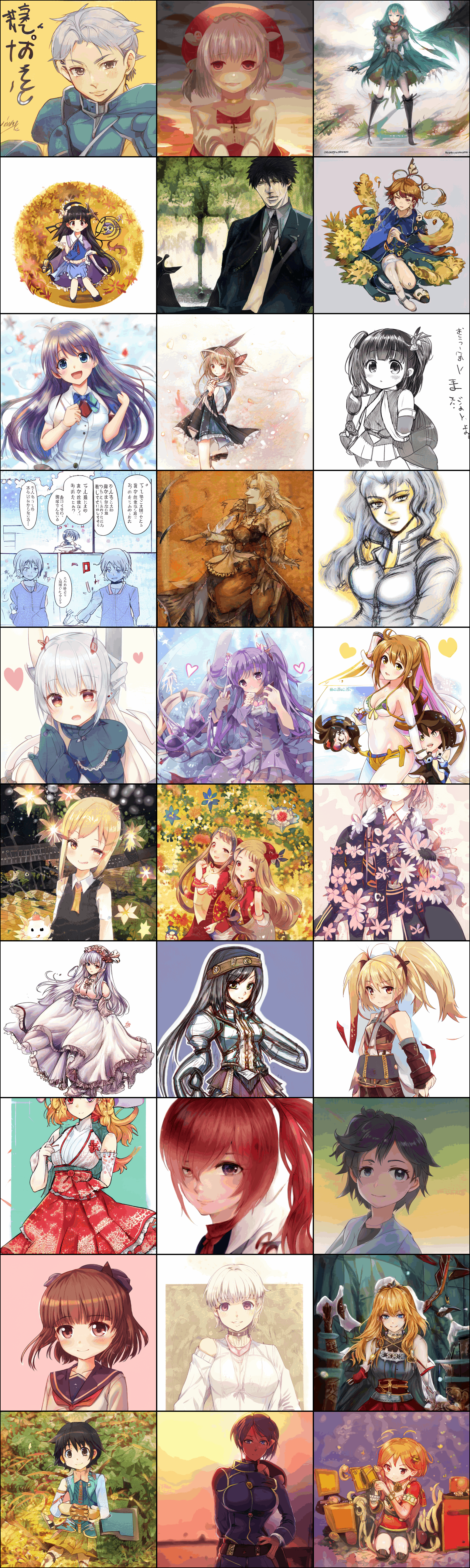
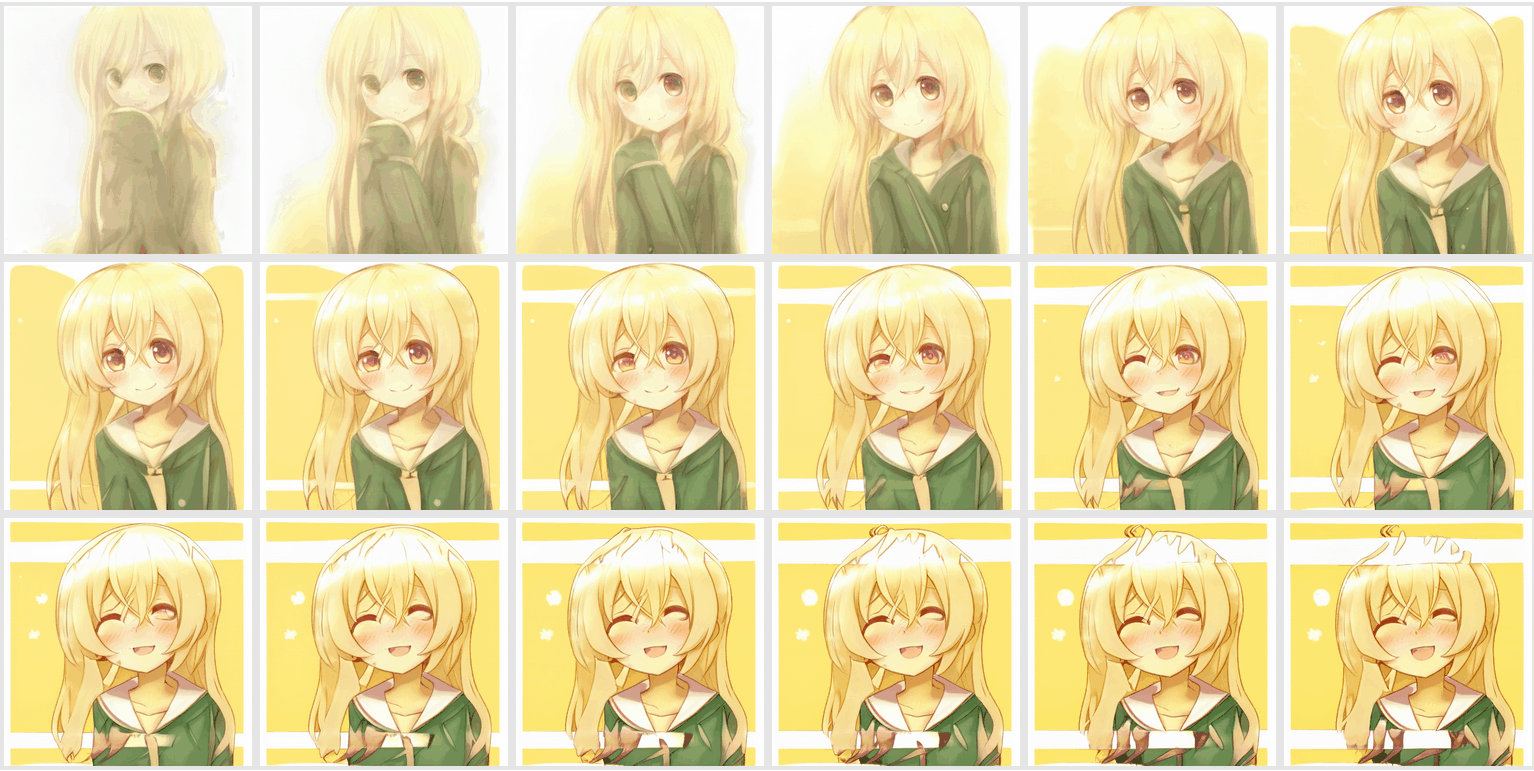
3×3 TADNE interpolation video (MP4); additional interpolation videos: 1, 2, 3, 4, 5, 6, 7
StyleGAN2-Ext Modifications
We call this modified StyleGAN2, StyleGAN2-ext. Broadly, Aydao’s StyleGAN2-ext increases the model size and disables regularizations, which are useful for restricted domains like faces but fail badly on more complex and multimodal domains.
His config, available in his StyleGAN2-ext repo (.ckpt
→ .pkl
/.pt
; Colab for generating images & interpolation videos, Ganspace, CLIP-based text → anime generator eg. text input “Asuka”, slider GUI editing notebook ):
-
disables perceptual path length regularization, reverting to the simple G logistic loss
-
disables style noise mixing (cf. 2021)
-
disables normalizing latents
-
disables mirroring/flipping data augmentation
-
does not use StyleGAN2-ADA data augmentation training: we have struggled to implement them on TPUs. (It’s unclear how much this matters, as most of ther 2020 flurry of data augmentation GAN research, and et al 2020’s StyleGAN2-ADA results, shows the best results in the small n regime: we and other StyleGAN2-ADA users have seen it make a huge difference when working with n < 5000, but no one has reported more than subtle improvements on ImageNet & beyond.)
-
-
weakens the R1 gradient penalty’s gamma parameter 10 → 5
-
model size increase (more than doubles, to 1GB total):
-
increases G feature-maps’ initial base value 10 → 32
-
increases the max feature-map value 512 → 1024
-
increased the latent w mapping/embedding module from 8×512 FC layers to 4×1024 FC layers33
l4rz has particularly noted benefits from expanding the width to 1024 (while decreasing layer count to keep model size in check), yielding his best results yet on eg. anime cosplayers (mirror:
rsync://176.9.41.242:873/biggan/2021-10-12-l4rz-stylegan2-xxxl-cosplayface-snapshot-001360-19520-FID359.pkl
) -
increases minibatch standard deviation to 32, and number of features to 4
-
TADNE Training
Training-wise:
-
the model was run for 5.26m iterations on a pre-emptible TPUv3-32 pod for a month or two (with interruptions) up to 2020-11-27, provided by Google TRC; quality was poor up to 2m, and then increased steadily to 4m
-
on Danbooru2019 original/full-sized SFW images (n = 2.82m), combined with Portraits (n = 0.3m), Figures (n = 0.85m), & PALM (n = 0.05m) for data augmentation (total n ~ 4m)
-
random cropping was initially used for the original images, but we learned midway through training from et al 2020 that random cropping severely damaged ImageNet performance of BigGAN and switched to top-cropping34 everywhere; StyleGAN2-ext benefited
-
decayed learning rate late in training
-
top-k training enabled late in training (minimal benefit, perhaps because enabled too late)
Observations:
-
Training remained much more stable than BigGAN runs, with no divergences
-
We observe that StyleGAN2-ext vastly outperforms baseline StyleGAN2 runs on Danbooru2019: the problems with StyleGAN2 appear to be less inherent weakness of the AdaIN architecture (as compared to BigGAN etc) than the extremely heavy regularization built into it by default (catering to small n/simple domains like human faces).
The key is disabling path length regularization & style mixing, at which point StyleGAN2 is able to scale & make good use of model size increases (rather than underfitting & churning endlessly).
Changes that did not help (much): adding self-attention to StyleGAN35, removing the “4×4 const” input, removing the w deep FC stack (adding it to BigGAN also proved destabilizing), removing the Laplacian pyramid/additive image architecture (didn’t help BigGAN much when added), large batch training.
-
Also of note is the surprisingly high quality writing—while disabling mirroring makes it possible to learn non-gibberish letters, we were still surprised how well StyleGAN2-ext can learn to write.
-
Hands have long been a weak point, but its hands are better than usual, and the addition of PALM to training caused an immediate quality improvement, demonstrating the benefits of carefully-targeted data augmentation.
-
PALM’s drawback: affecting G. The drawback of using PALM is that the final model generates closeup hands occasionally. Our original plan was to remove the data augmentation datasets at the end of training and finetune for a short period, to teach it to not generate the PALM-style hands—but when Aydao tried that, the hands in the regular images began to degrade as StyleGAN2-ext apparently began to forget how to do hands! So it would be better to leave the data augmentation datasets in, and instead, screen out generated hands using the original PALM YOLOv3 hand-detection model.
-
-
But despite the quality, the usual artifacts remain: artifacts that I’ve observed in earlier StyleGAN2 runs (like This Fursona Does Not Exist/This Pony Does Not Exist), continue to appear, such as strangely smooth transitions of discrete objects such as necklaces or sleeves, and ‘cellular division’ of 1 🡺 2 heads/characters/bodies.
I speculate these artifacts, which remain present despite great increases in quality, are caused by the smooth z/w latent space which is forced to transform Gaussian variables into binary indicators, and does so poorly (eg. in TPDNE, many samples of ponies have stubby pseudo-horns, even though ponies in the original artwork either have a horn or do not, and in TWDNE, there are faces with ambiguous half-glasses smeared on them); the BigGAN paper notes that there were gains from using non-normal variables like binary variables, and I would predict that salting in binary variables or censored (rectified) normals (a change I call ‘mixture latents’) to the latent space would fix this.
-
Interestingly, the latent space is qualitatively different and ‘larger’ than our earlier StyleGANs: whereas the face/portrait StyleGANs favor a sweet spot of ψ ~ 0.7, and samples seriously deteriorate by ψ ~ 1.2, the Danbooru2019 StyleGAN2-ext is best at ψ ~ 1.1 and only begins noticeably degrading by ψ ~ 1.4, with good samples findable all the way up to 2.0 (!).
-
-
Fine details/textures are poor; this suggests l4rz’s scaling strategy of reallocating parameters from the global/low-resolution layers to the higher-resolution layers would be useful
-
Overall, we can do even better!—we expect that a fresh training run incorporating the final hyperparameters, combined with additional images from Danbooru2021 & possibly a new arm/torso-focused data augmentation, would be noticeably better. (However, as surprisingly well as StyleGAN2-ext scaled, I personally still think BigGAN will scale better, and there are better architectures on the horizon, particularly DALL·E & diffusion models.)
-
We have not tried transfer learning (aside from Arfa’s preliminary furry StyleGAN2-ext), but given how well transfer learning has worked for n as small as n ~ 100 for the Face/Portrait models, we expect it to work well for this model too—and potentially give even better results on faces, as it was trained on a superset of the Face/Portrait data & knows more about bodies/backgrounds/headwear/etc.
-
TADNE Download
Download (as with all our models, the model/samples is licensed under CC-0 or public domain):
-
Model:
-
Mega (1GB)
-
Rsync mirror:
rsync -v rsync://176.9.41.242:873/biggan/2020-11-27-aydao-stylegan2ext-danbooru2019s-512px-5268480.pkl ./
-
-
Images: tarballs of all images, ordered by ψ (>1.8m images; >618GB)
Transfer Learning
In the days when Sussman was a novice, Minsky once came to him as he sat hacking at the PDP-6.
“What are you doing?”, asked Minsky. “I am training a randomly wired neural net to play Tic-Tac-Toe”, Sussman replied. “Why is the net wired randomly?”, asked Minsky. “I do not want it to have any preconceptions of how to play”, Sussman said.
Minsky then shut his eyes. “Why do you close your eyes?”, Sussman asked his teacher. “So that the room will be empty.”
At that moment, Sussman was enlightened.
“Sussman attains enlightenment”, “AI Koans”, Jargon File
One of the most useful things to do with a trained model on a broad data corpus is to use it as a launching pad to train a better model quicker on lesser data, called “transfer learning”. For example, one might transfer learn from Nvidia’s FFHQ face StyleGAN model to a different celebrity dataset, or from bedrooms → kitchens. Or with the anime face model, one might retrain it on a subset of faces—all characters with red hair, or all male characters, or just a single specific character. Even if a dataset seems different, starting from a pretrained model can save time; after all, while male and female faces may look different and it may seem like a mistake to start from a mostly-female anime face model, the alternative of starting from scratch means starting with a model generating random rainbow-colored static, and surely male faces look far more like female faces than they do random static?36 Indeed, you can quickly train a photographic face model starting from the anime face model.
This extends the reach of good StyleGAN models from those blessed with both big data & big compute to those with little of either. Transfer learning works particularly well for specializing the anime face model to a specific character: the images of that character would be too little to train a good StyleGAN on, too data-impoverished for the sample-inefficient StyleGAN1–237, but having been trained on all anime faces, the StyleGAN has learned well the full space of anime faces and can easily specialize down without overfitting. Trying to do, say, faces ↔︎ landscapes is probably a bridge too far.
Data-wise, for doing face specialization, the more the better but n = 500–5000 is an adequate range, but even as low as n = 50 works surprisingly well. I don’t know to what extent data augmentation can substitute for original datapoints but it’s probably worth a try especially if you have n < 5000.
Compute-wise, specialization is rapid. Adaptation can happen within a few ticks, possibly even 1. This is surprisingly fast given that StyleGAN is not designed for few-shot/transfer learning. I speculate that this may be because the StyleGAN latent space is expressive enough that even new faces (such as new human faces for a FFHQ model, or a new anime character for an anime-face model) are still already present in the latent space. Examples of the expressivity are provided by et al 2019 , who find that “although the StyleGAN generator is trained on a human face dataset [FFHQ], the embedding algorithm is capable of going far beyond human faces. As Figure 1 shows, although slightly worse than those of human faces, we can obtain reasonable and relatively high-quality embeddings of cats, dogs and even paintings and cars.” If even images as different as cars can be encoded successfully into a face StyleGAN, then clearly the latent space can easily model new faces and so any new face training data is in some sense already learned; so the training process is perhaps not so much about learning ‘new’ faces as about making the new faces more ‘important’ by expanding the latent space around them & contracting it around everything else, which seems like a far easier task.
How does one actually do transfer learning? Since StyleGAN is (currently) unconditional with no dataset-specific categorical or text or metadata encoding, just a flat set of images, all that has to be done is to encode the new dataset and simply start training with an existing model. One creates the new dataset as usual, and then edits training.py
with a new -desc
line for the new dataset, and if resume_kimg
is set correctly (see next paragraph) and resume_run_id = "latest"
enabled as advised, you can then run python train.py
and presto, transfer learning.
The main problem seems to be that training cannot be done from scratch/0 iterations, as one might naively assume—when I tried this, it did not work well and StyleGAN appeared to be ignoring the pretrained model. My hypothesis is that as part of the progressive growing/fading in of additional resolution/layers, StyleGAN simply randomizes or wipes out each new layer and overwrites them—making it pointless. This is easy to avoid: simply jump the training schedule all the way to the desired resolution. For example, to start at one’s maximum size (here 512px) one might set resume_kimg=7000
in training_loop.py
. This forces StyleGAN to skip all the progressive growing and load the full model as-is. To make sure you did it right, check the first sample (fakes07000.png
or whatever), from before any transfer learning training has been done, and it should look like the original model did at the end of its training. Then subsequent training samples should show the original quickly morphing to the new dataset. (Anything like fakes00000.png
should not show up because that indicates beginning from scratch.)
Anime Faces → Character Faces
Holo
The first transfer learning was done with Holo of Spice and Wolf. It used a 512px Holo face dataset created with Nagadomi’s cropper from all of Danbooru2017, upscaled with waifu2x
, cleaned by hand, and then data-augmented from n = 3900 to n = 12600; mirroring was enabled since Holo is symmetrical. I then used the anime face model as of 2019-02-09—it was not fully converged, indeed, wouldn’t converge with weeks more training, but the quality was so good I was too curious as to how well retraining would work so I switched gears.
It’s worth mentioning that this dataset was used previously with ProGAN, where after weeks of training, ProGAN overfit badly as demonstrated by the samples & interpolation videos.
Training happened remarkably quickly, with all the faces converted to recognizably Holo faces within a few hundred iterations:
Training montage of a Holo face model initialized from the anime face StyleGAN (blink & you’ll miss it)
Interpolation video of the Holo face model initialized from the anime face StyleGAN
The best samples were convincing without exhibiting the failures of the ProGAN:
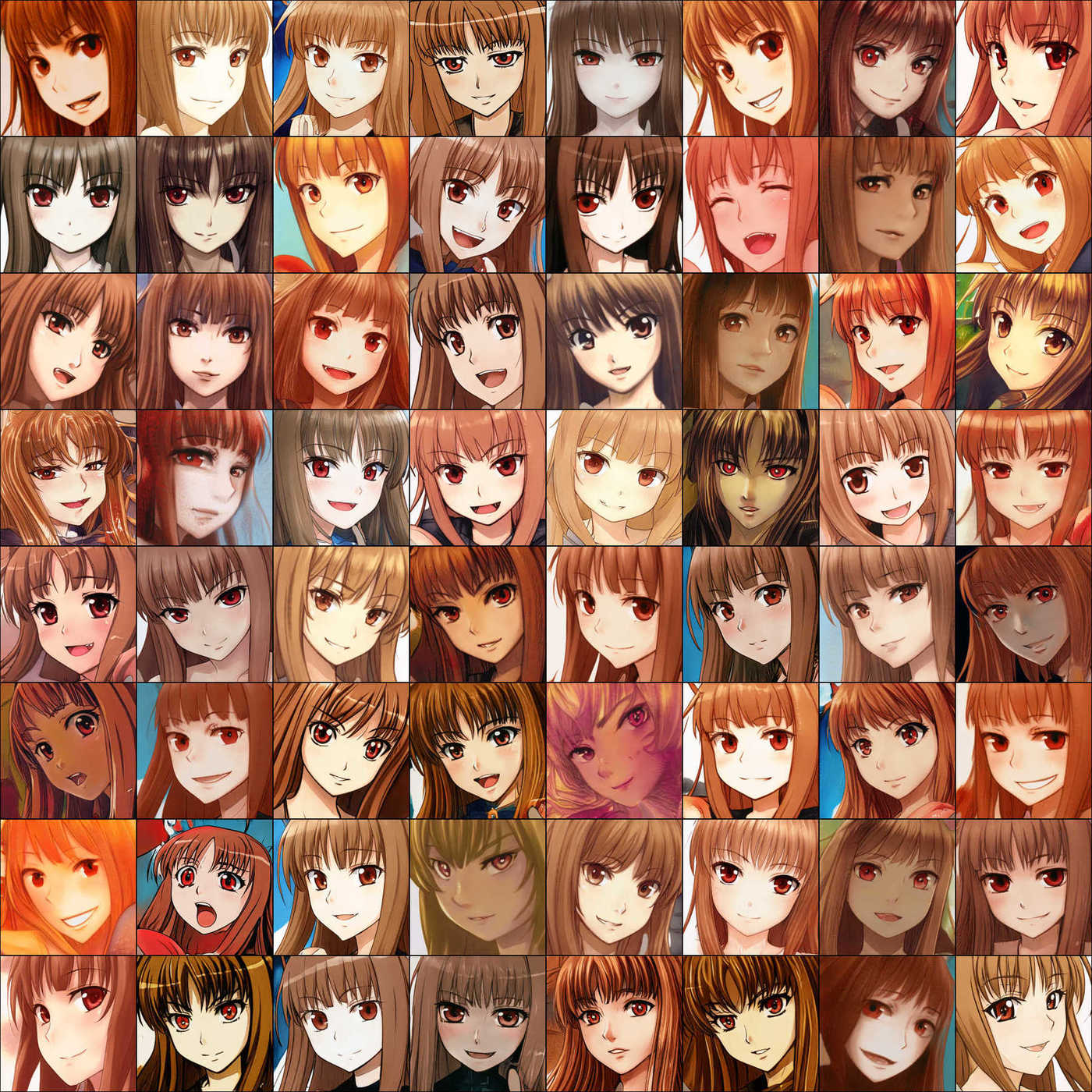
The StyleGAN was much more successful, despite a few failure latent points carried over from the anime faces. Indeed, after a few hundred iterations, it was starting to overfit with the ‘crack’ artifacts & smearing in the interpolations. The latest I was willing to use was iteration #11370, and I think it is still somewhat overfit anyway. I thought that with its total n (after data augmentation), Holo would be able to train longer (being 1⁄7th the size of FFHQ), but apparently not. Perhaps the data augmentation is considerably less valuable than 1-for-1, either because the invariants encoded in aren’t that useful (suggesting that et al 2018-like style transfer data augmentation is what’s necessary) or that they would be but the anime face StyleGAN has already learned them all as part of the previous training & needs more real data to better understand Holo-like faces. It’s also possible that the results could be improved by using one of the later anime face StyleGANs since they did improve when I trained them further after my 2 Holo/Asuka transfer experiments.
Nevertheless, impressed, I couldn’t help but wonder if they had reached human-levels of verisimilitude: would an unwary viewer assume they were handmade?
So I selected ~100 of the best samples (24MB; Imgur mirror) from a dump of 2000, cropped about 5% from the left/right sides to hide the background artifacts a little bit, and submitted them on 2019-02-11 to /r/SpiceandWolf under an alt account. I made the mistake of sorting by filesize & thus leading with a face that was particularly suspicious (streaky hair) so one Redditor voiced the suspicion they were from MGM (absurd yet not entirely wrong) but all the other commenters took the faces in stride or praising them, and the submission received +248 votes (99% positive) by March. A Redditor then turned them all into a GIF video which earned +192 (100%) and many positive comments with no further suspicions until I explained. Not bad indeed.
The #11370 Holo StyleGAN model is available for download.
Asuka
After the Holo training & link submission went so well, I knew I had to try my other character dataset, Asuka, using n = 5300 data-augmented to n = 58,000.38 Keeping in mind how data seemed to limit the Holo quality, I left mirroring enabled for Asuka, even though she is not symmetrical due to her 3.0 eyepatch over her left eye (as purists will no doubt note).
Training montage of an Asuka face model initialized from the anime face StyleGAN
Interpolation video of the Asuka face model initialized from the anime face StyleGAN
Interestingly, while Holo trained within 4 GPU-hours, Asuka proved much more difficult and did not seem to be finished training or showing the cracks despite training twice as long. Is this due to having ~35% more real data, having 10× rather than 3× data augmentation, or some inherent difference like Asuka being more complex (eg. because of more variations in her appearance like the eyepatches or plugsuits)?
I generated 1000 random samples with Ψ = 1.2 because they were particularly interesting to look at. As with Holo, I picked out the best 100 (13MB; Imgur mirror) from ~2000:
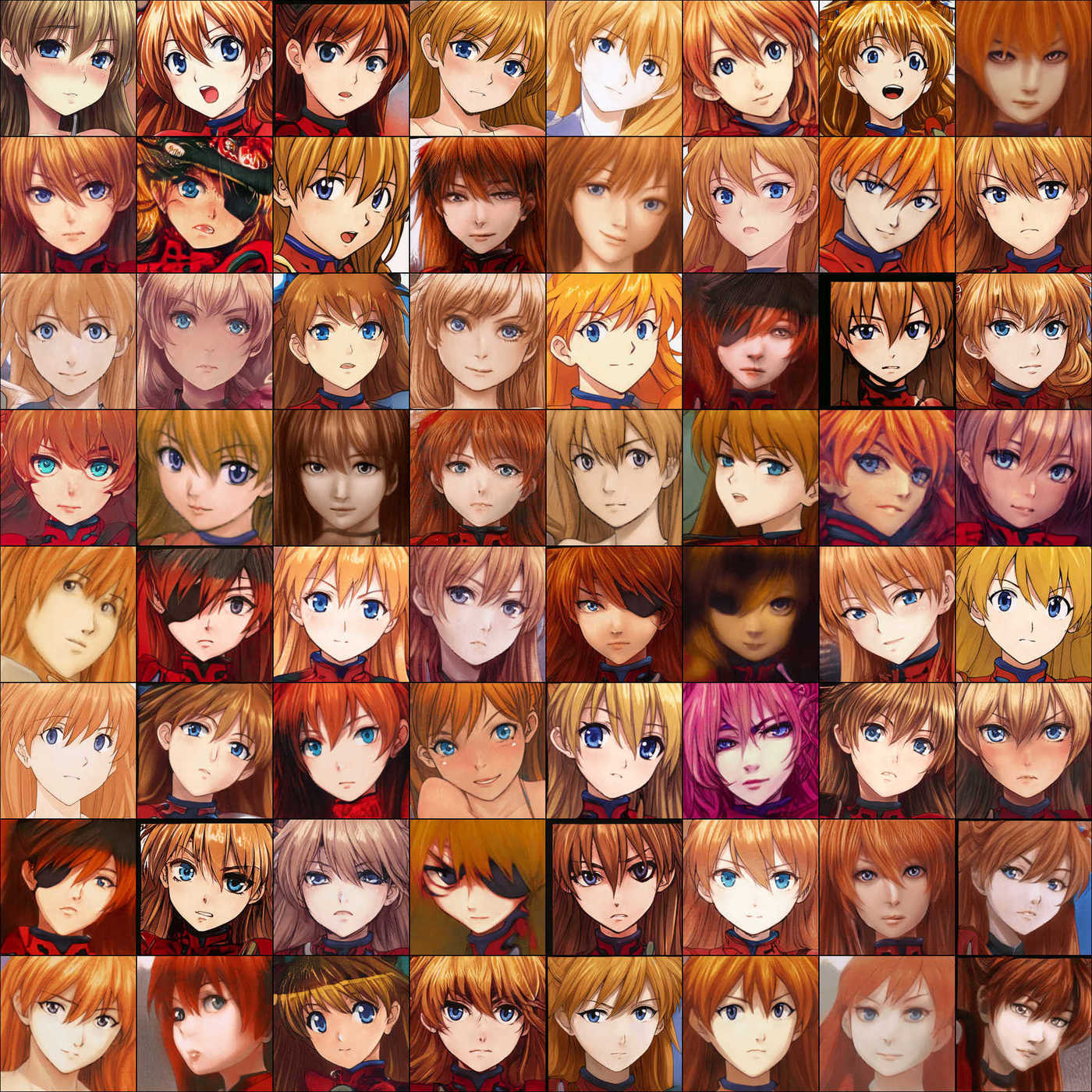
And I submitted to the /r/Evangelion subreddit, where it also did well (+109, 98%); there were no speculations about the faces being NN-generated before I revealed it, merely requests for more. Between the two, it appears that with adequate data (n > 3000) and moderate curation, a simple kind of art Turing test can be passed.
The #7903 Asuka StyleGAN model is available for download.
Zuihou
In early February 2019, using the then-released model, Redditor Ending_Credits tried transfer learning to n = 500 faces of the Kantai Collection Zuihou for ~1 tick (~60k iterations).
The samples & interpolations have many artifacts, but the sample size is tiny and I’d consider this good finetuning from a model never intended for few-shot learning:
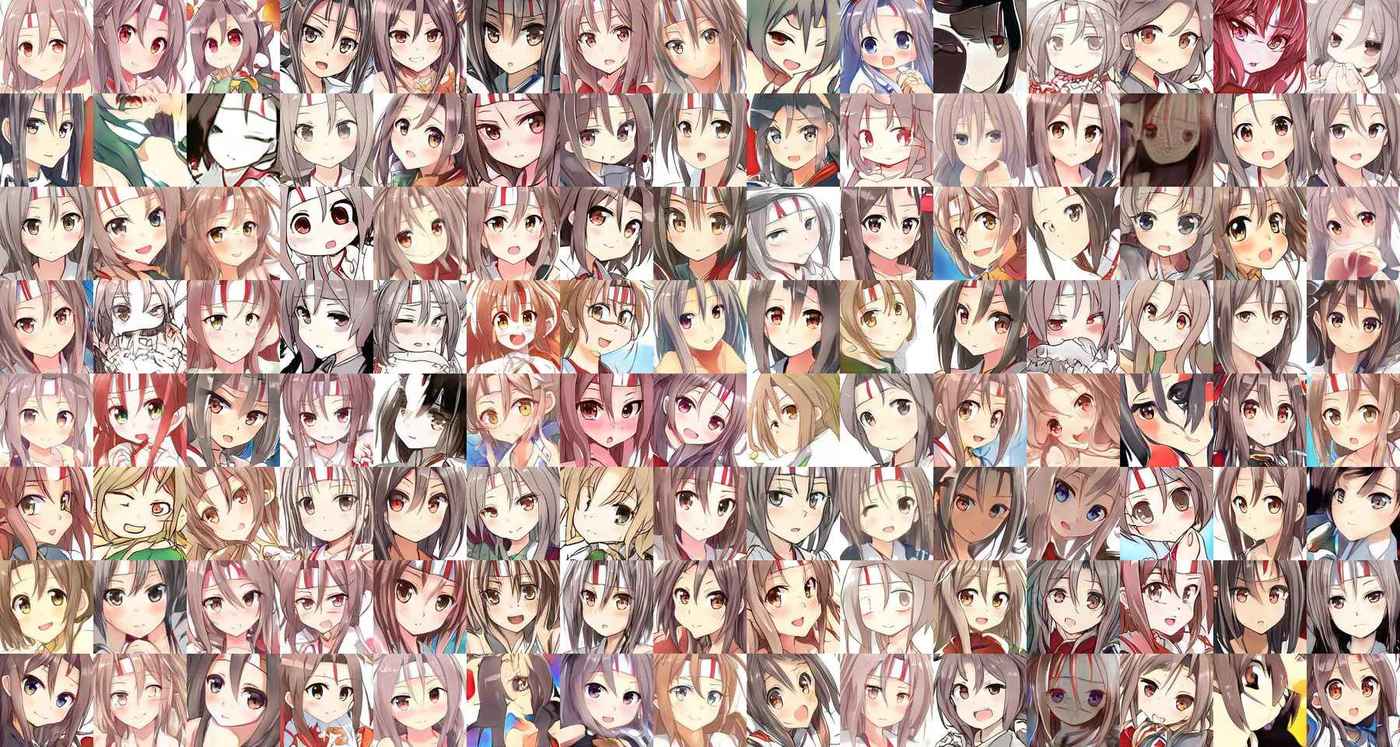
Interpolation video (4×4) of the Zuihou face model initialized from the anime face StyleGAN, trained by Ending_Credits
Interpolation video (1×1) of the Zuihou face model initialized from the anime face StyleGAN, trained by Ending_Credits
Probably it could be made better by starting from the latest anime face StyleGAN model, and using aggressive data augmentation. Another option would be to try to find as many characters which look similar to Zuihou (matching on hair color might work) and train on a joint dataset—unconditional samples would then need to be filtered for just Zuihou faces, but perhaps that drawback could be avoided by a third stage of Zuihou-only training?
Ganso
Akizuki
Another Kancolle character, Akizuki, was trained in April 2019 by Ganso.
Ptilopsis
In January 2020, Ganso trained a StyleGAN 2 model from the S2 portrait model on a tiny corpus of Ptilopsis images, a character from Arknights, a 2017 Chinese tower defense RPG mobile game.
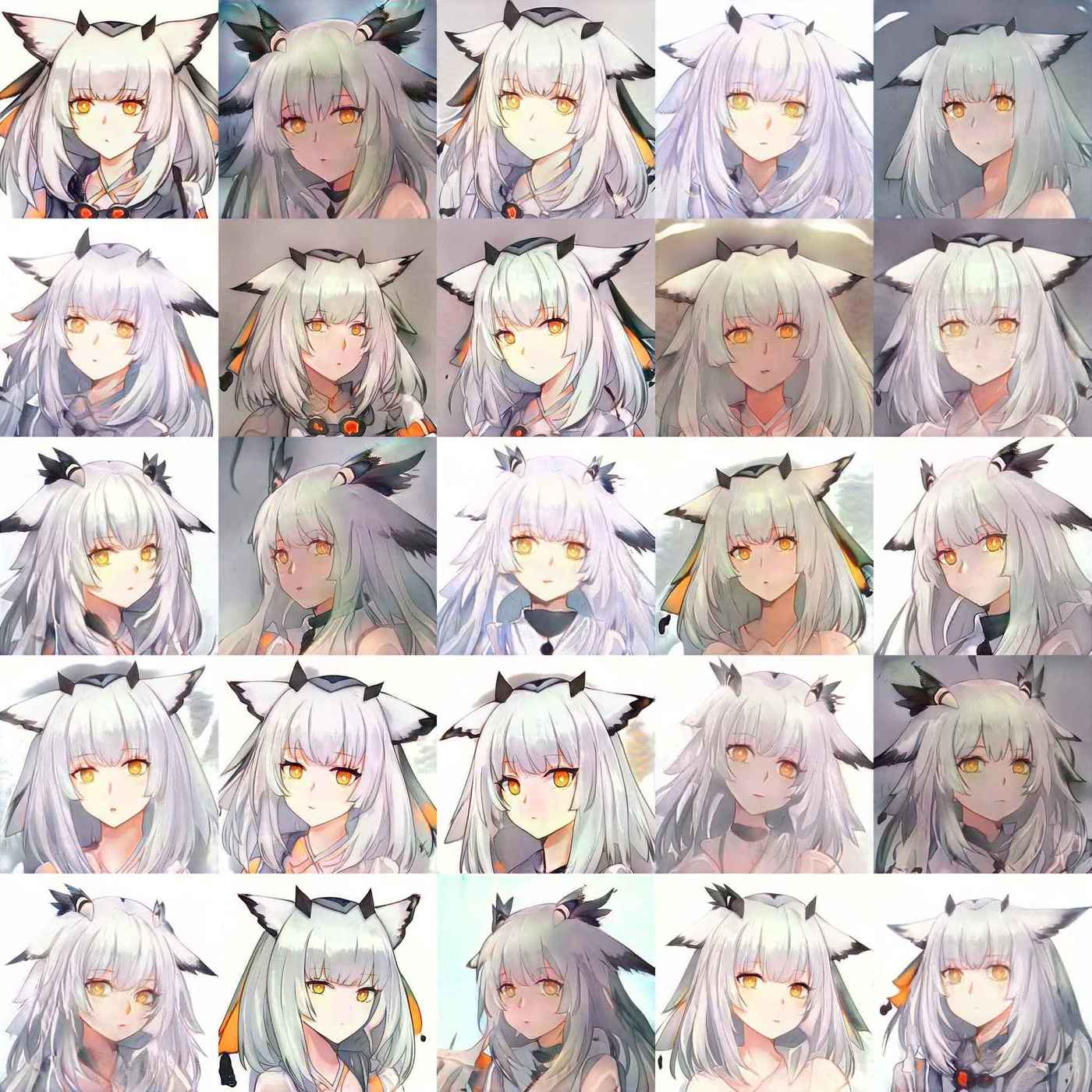
Ptilopsis are owls, and her character design shows prominent ears; despite the few images to work with (just 21 on Danbooru as of 2020-01-19), the interpolation shows smooth adjustments of the ears in all positions & alignments, demonstrating the power of transfer learning:
Interpolation video (4×4) of the Ptilopsis face model initialized from the anime face StyleGAN 2, trained by Ganso
Fate
Saber
Ending_Credits likewise did transfer to Saber (Fate/stay night), n = 4000. The results look about as expected given the sample sizes and previous transfer results:
Interpolation video (4×4) of the Saber face model initialized from the anime face StyleGAN, trained by Ending_Credits
Fate/Grand Order
Michael Sugimura in May 2019 experimented with transfer learning from the 512px anime portrait GAN to faces cropped from ~6k Fate/Grand Order wallpapers he downloaded via Google search queries. His results for Saber & related characters look reasonable but more broadly, somewhat low-quality, which Sugimura suspects is due to inadequate data cleaning (“there are a number of lower quality images and also images of backgrounds, armor, non-character images left in the dataset which causes weird artifacts in generated images or just lower quality generated images.”).
Louise
Finally, Ending_Credits did transfer to Louise (Zero no Tsukaima), n = 350:
Interpolation video (4×4) of the Louise face model initialized from the anime face StyleGAN, trained by Ending_Credits
Not as good as Saber due to the much smaller sample size.
Lelouch
roadrunner01 experimented with a number of transfers, including a transfer of the male character Lelouch Lamperouge (Code Geass) with n = 50 (!), which is not nearly as garbage as it should be.
Asashio
FlatisDogchi experimented with transfer to n = 988 (augmented to n = 18772) Asashio (KanColle) faces, creating “This Asashio Does Not Exist”.
Marisa Kirisame & the Komeijis
A Japanese user mei_miya posted an interpolation video of the Touhou character Marisa Kirisame by transfer learning on 5000 faces. They also did the Touhou characters Satori/Koishi Komeiji with n = 6000.
The Reddit user Jepacor also has done Marisa, using Danbooru samples.
Lexington
A Chinese user 3D_DLW (S2 writeup/tutorial: 1/2) in February 2020 did transfer-learning from the S2 portrait model to Pixiv artwork of the character Lexington from Warship Girls. He used a similar workflow: cropping faces with lbpcascade_animeface
, upscaling with waifu2x, and cleaning with ranker.py
(using the original S2 model’s Discriminator & producing datasets of varying cleanliness at n = 302–1659). Samples:
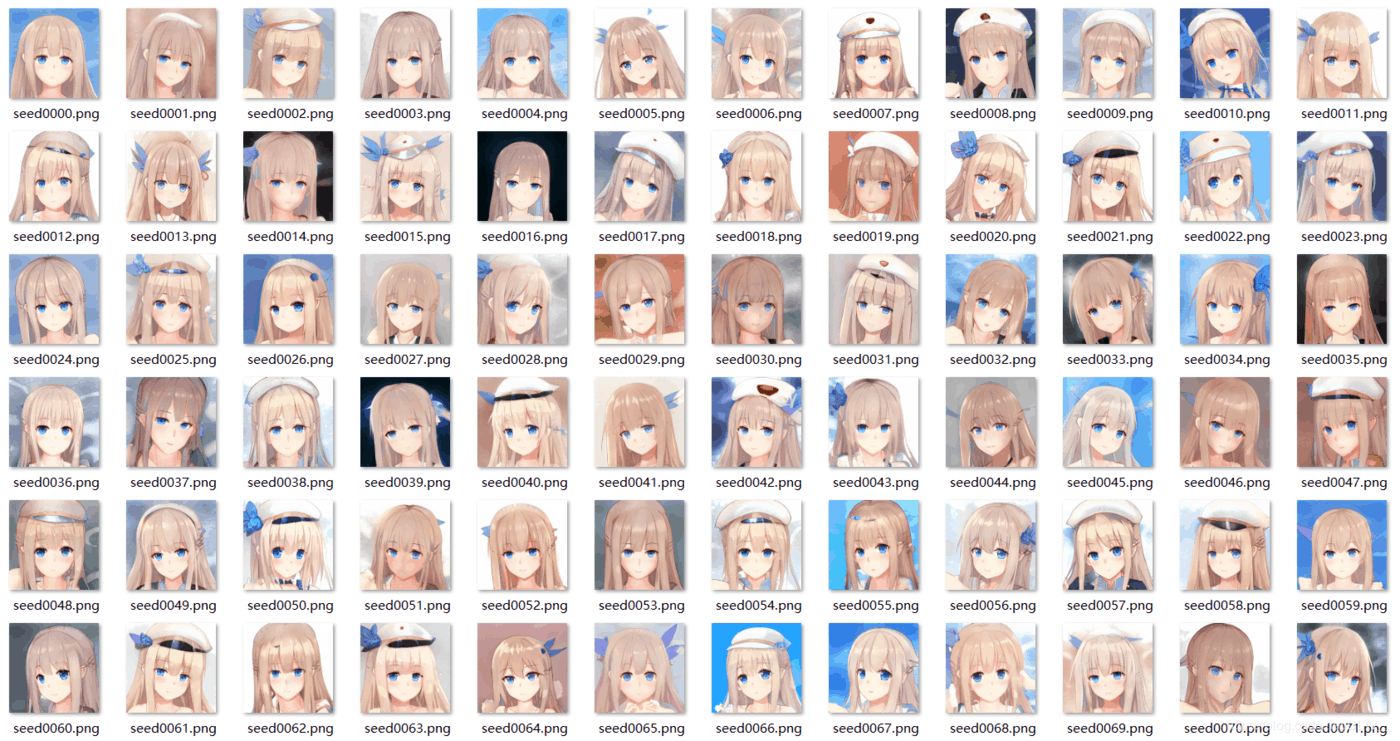
Hayasaka Ai
Tazik Shahjahan finetuned S2 on Kaguya-sama: Love Is War’s Hayasaka Ai, providing a Colab notebook demonstrating how he scraped Pixiv and filtered out invalid images to create the training corpus
Ahegao
CaJI9I created an “ahegao” StyleGAN; unspecified corpus or method:
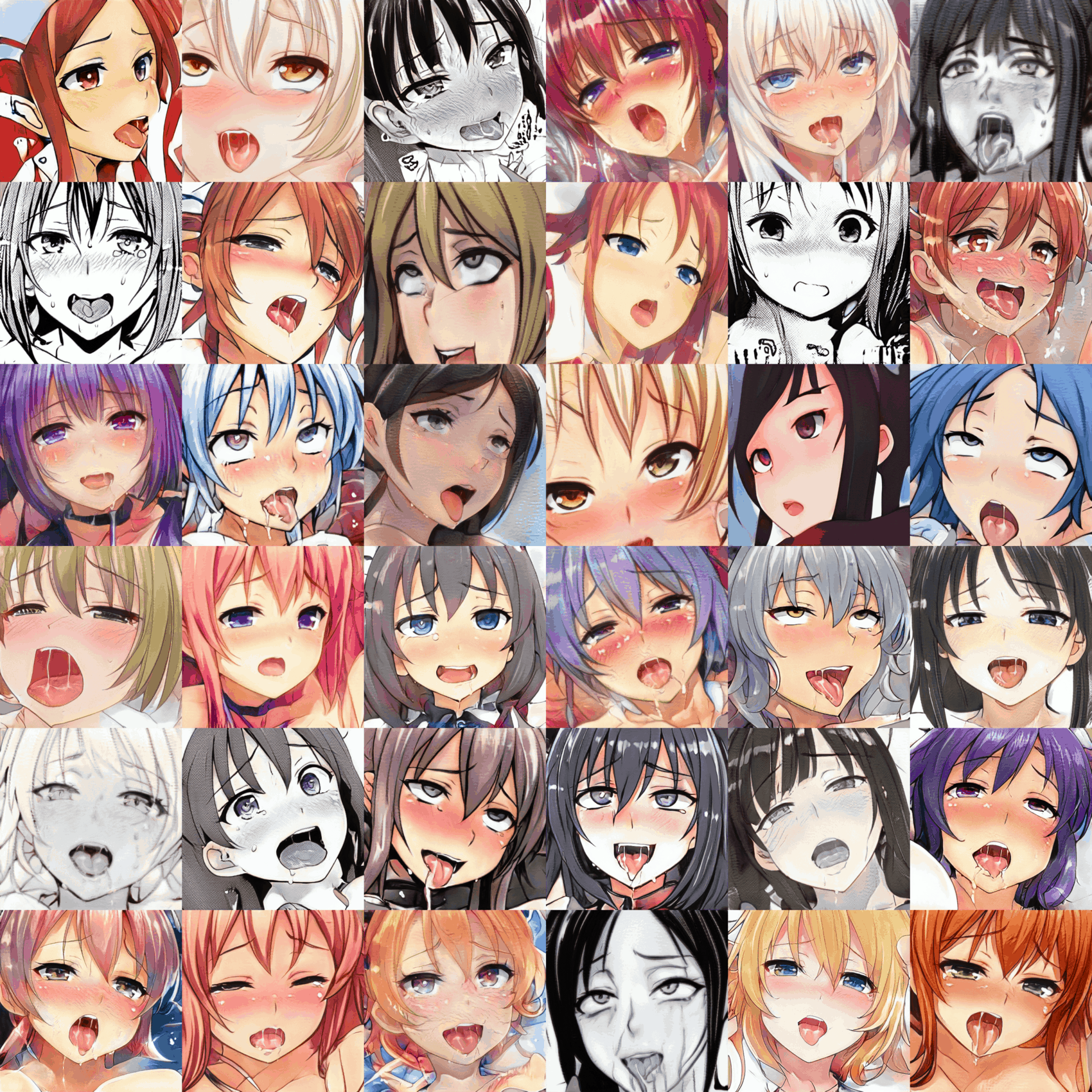
Re:Zero
Emilia
Interpolation video (4×4) of the Emilia Re:Zero face model initialized from the Portrait StyleGAN, trained by shipblazer420
Rem
Andy8744 (2022-02-13) used the face model to train on 725 cropped (using a custom YOLOv5) Pixiv images of Re:Zero character Rem, trained for ~20 GPU-hours.
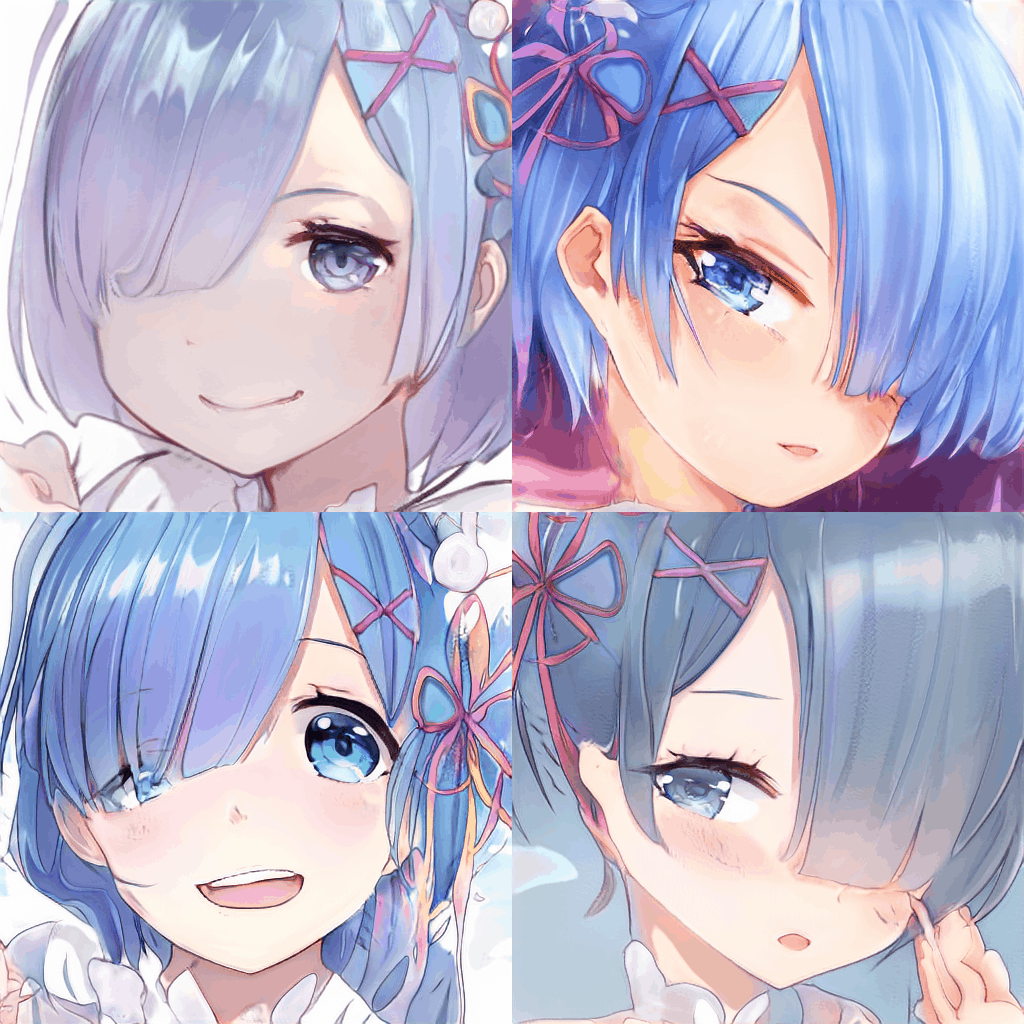
Lord_YuanYuan
Po-Hung Yeh has transfered (2022-02-13) the face model to faces from a Taiwanese artist, Neko_YuanYuan, dubbing it “YuanGAN”.
Ganyu (Genshin Impact)
Andy8744 (2022-02-22) made a Kaggle notebook for finetuning on n = 850 512×512px faces of the gacha game franchise Genshin Impact character Ganyu, training for ~145kimg or ~11 GPU-hours. (It may have been transfer-learned from his Rem model.)
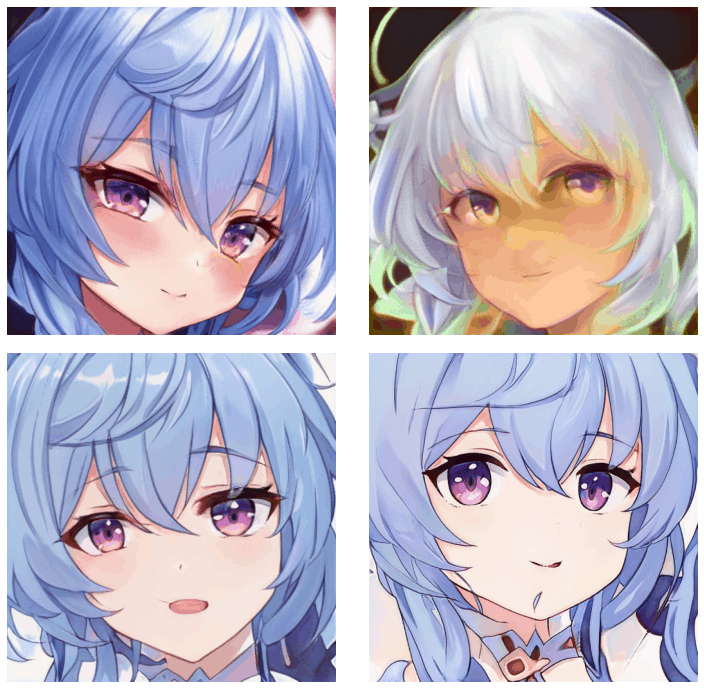
Anime Faces → Anime Headshots
Twitter user Sunk did transfer learning to an image corpus of a specific artist, Kurehito Misaki (深崎暮人), n ≈ 1000. His images work well and the interpolation looks nice:
Interpolation video (4×4) of the Louise face model initialized from the Kurehito Misaki StyleGAN, trained by sunk
Anime Faces → Portrait
TWDNE was a huge success and popularized the anime face StyleGAN. It was not perfect, though, and flaws were noted.
Portrait Improvements
The portraits could be improved by more carefully selecting SFW images to avoid overly-suggestive faces, expanding the crops to avoid cutting off edges of heads like hairstyles,
For details and downloads, please see Danbooru2019 Portraits.
Portrait Results
After retraining the final face StyleGAN 2019-03-08–2019-04-30 on the new improved portraits dataset, the results improved:
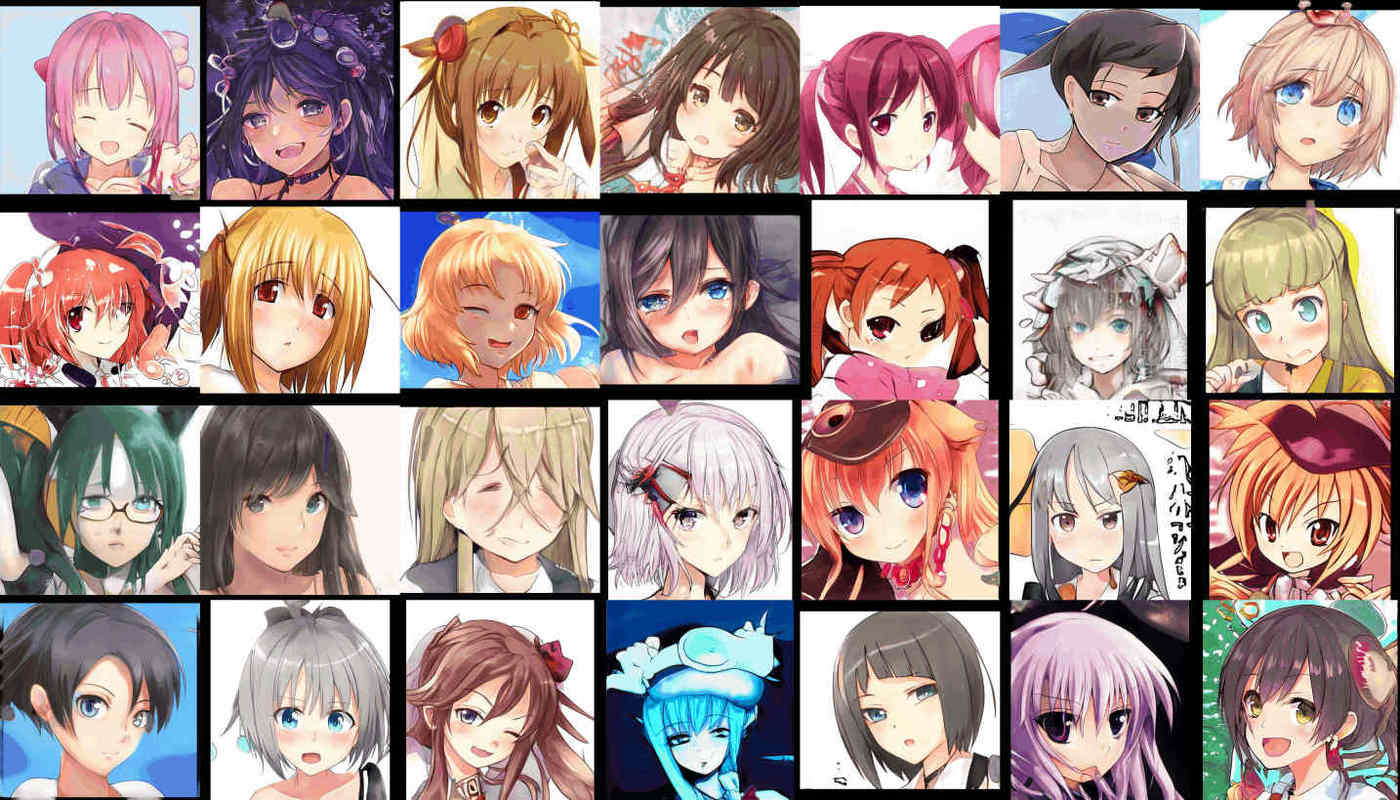
Interpolation video (4×4) of the Danbooru2018 portrait model initialized from the Danbooru2017 face StyleGAN
This S1 anime portrait model is obsoleted by the StyleGAN 2 portrait model.
The final model from 2019-04-30 is available for download.
I used this model at 𝛙=0.5 to generate 100,000 new portraits for TWDNE (#100,000–199,999), balancing the previous faces.
I was surprised how difficult upgrading to portraits seemed to be; I spent almost two months training it before giving up on further improvements, while I had been expecting more like a week or two. The portrait results are indeed better than the faces (I was right that not cropping off the top of the head adds verisimilitude), but the upgrade didn’t impress me as much as the original faces did compared to earlier GANs. And our other experimental runs on whole-Danbooru2018 images never progressed beyond suggestive blobs during this period.
I suspect that StyleGAN—at least, on its default architecture & hyperparameters, without a great deal more compute—is reaching its limits here, and that changes may be necessary to scale to richer images. (Self-attention is probably the easiest to add since it should be easy to plug in additional layers to the convolution code.)
Anime Faces → Male Faces
A few people have observed that it would be nice to have an anime face GAN for male characters instead of always generating female ones. The anime face StyleGAN does in fact have male faces in its dataset as I did no filtering—it’s merely that female faces are overwhelmingly frequent (and it may also be that male anime faces are relatively androgynous/feminized anyway so it’s hard to tell any difference between a female with short hair & a guy39).
Training a male-only anime face StyleGAN would be another good application of transfer learning.
The faces can be easily extracted out of Danbooru2018 by querying for "male_focus"
, which will pick up ~150k images. More narrowly, one could search "1boy"
& "solo"
, to ensure that the only face in the image is a male face (as opposed to, say, 1boy 1girl
, where a female face might be cropped out as well). This provides n = 99k raw hits. It would be good to also filter out ‘trap’ or overly-female-looking faces (else what’s the point?), by filtering on tags like cat ears or particularly popular ‘trap’ characters like Fate/Grand Order’s Astolfo. A more complicated query to pick up scenes with multiple males could be to search for both "1boy"
& "multiple_boys"
and then filter out "1girl"
& "multiple_girls"
, in order to select all images with 1 or more males and then remove all images with 1 or more females; this doubles the raw hits to n = 198k. (A downside is that the face-cropping will often unavoidably yield crops with two faces, a primary face and an overlapping face, which is bad and introduces artifacting when I tried this with all faces.)
Combined with transfer learning from the general anime face StyleGAN, the results should be as good as the general (female) faces.
I settled for "1boy"
& "solo"
, and did considerable cleaning by hand. The raw count of images turned out to be highly misleading, and many faces are unusable for a male anime face StyleGAN: many are so highly stylized (such as action scenes) as to be damaging to a GAN, or they are almost indistinguishable from female faces (because they are bishonen or trap or just androgynous), which would be pointless to include (the regular portrait StyleGAN covers those already). After hand cleaning & use of ranker.py
, I was left with n~3k, so I used heavy data augmentation to bring it up to n~57k, and I initialized from the final portrait StyleGAN for the highest quality.
It did not overfit after ~4 days of training, but the results were not noticeably improving, so I stopped (in order to start training the GPT-2-345M, which OpenAI had just released, on poetry). There are hints in the interpolation videos, I think, that it is indeed slightly overfitting, in the form of ‘glitches’ where the image abruptly jumps slightly, presumably to another mode/face/character of the original data; nevertheless, the male face StyleGAN mostly works.
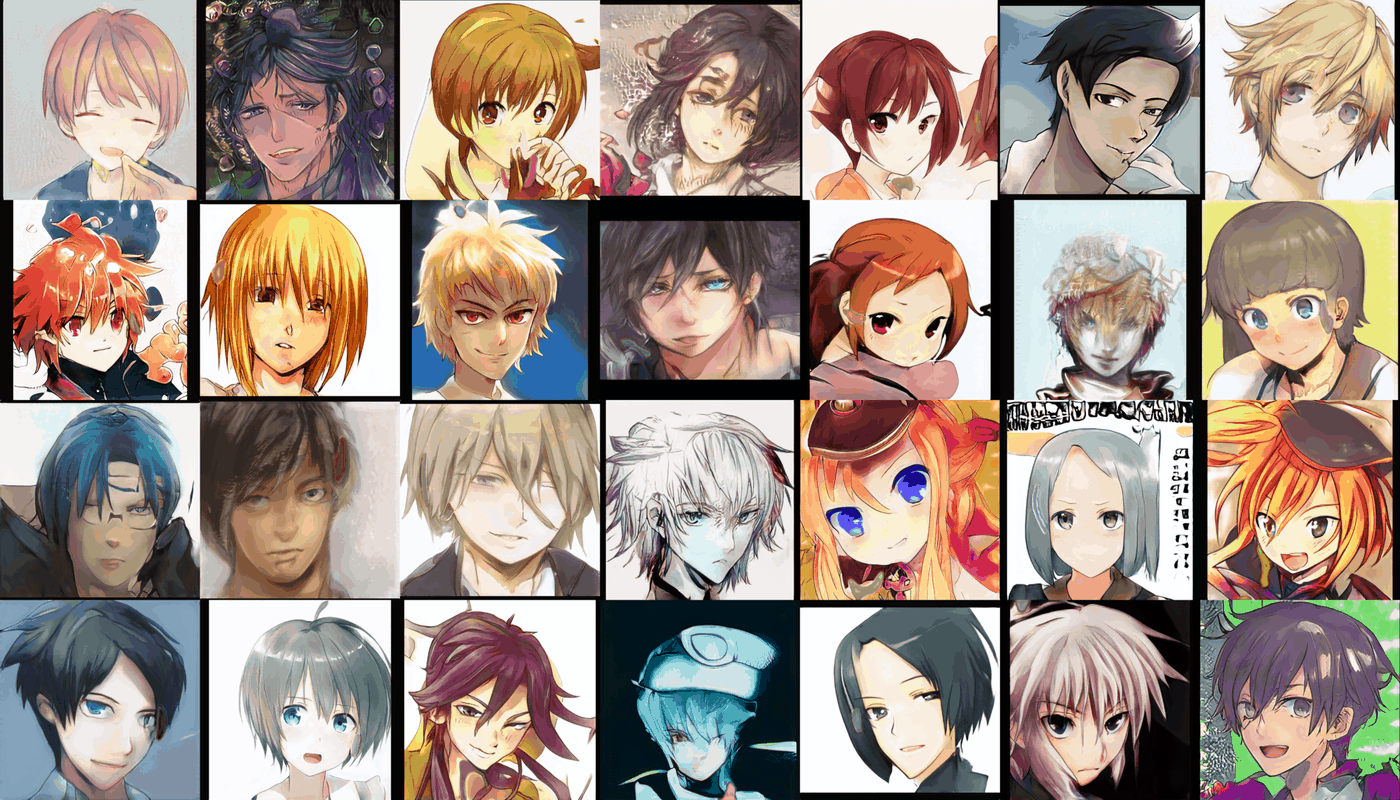
Interpolation video (4×4) of the Danbooru2018 male faces model initialized from the Danbooru2018 portrait StyleGAN
The male face StyleGAN model is available for download, as is 1000 random faces with 𝛙=0.7 (mirror; partial Imgur album).
Anime Faces → Ukiyo-E Faces
In January 2020, Justin (@Buntworthy) used 5000 ukiyo-e faces cropped with Amazon Rekognition from Ukiyo-e Search to do transfer learning. After ~24h training:
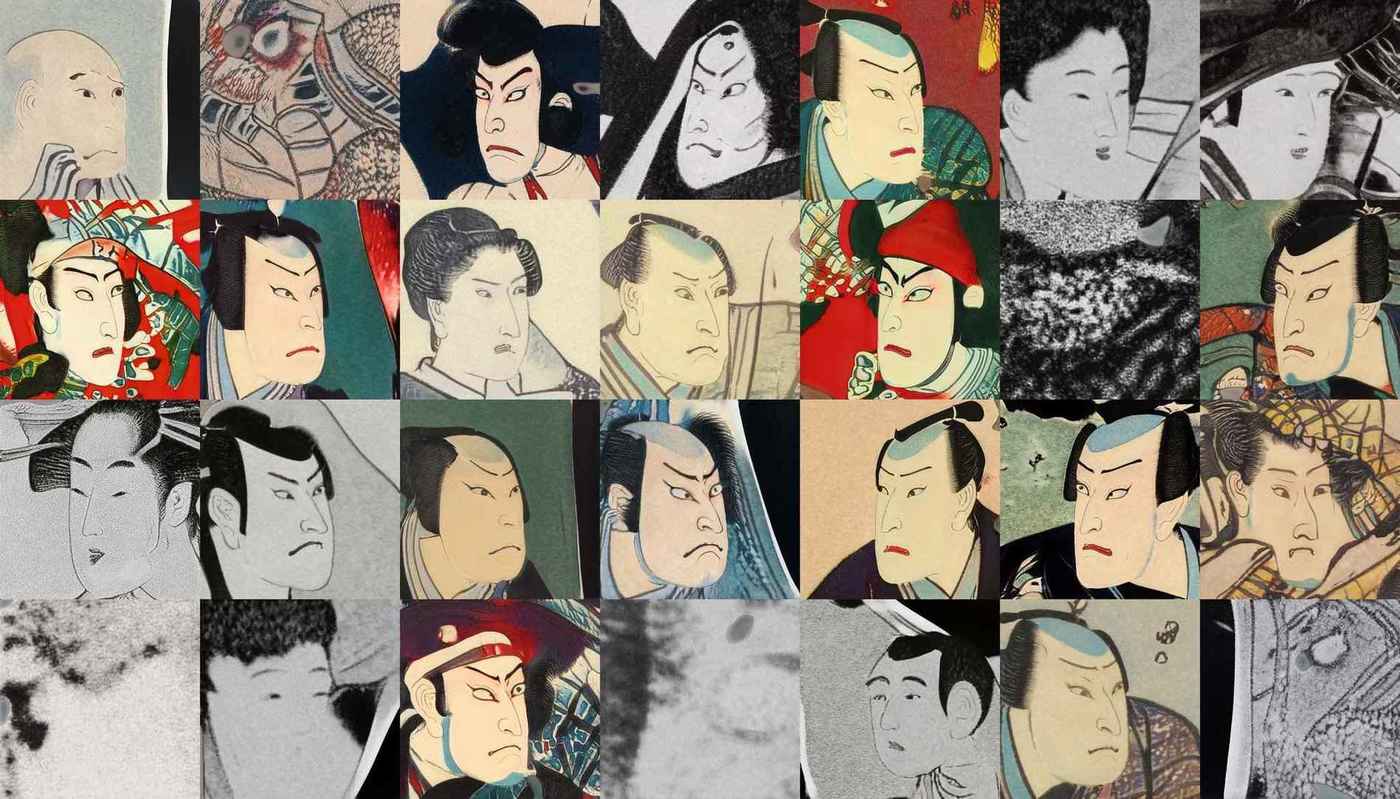
Anime Faces → Western Portrait Faces
In 2019, aydao experimented with transfer learning to European portrait faces drawn from WikiArt; the transfer learning was done via Nathan Shipley’s abuse of SWA where two models are simply averaged together, parameter by parameter and layer by layer, to yield a new model. (Surprisingly, this works—as long as the models aren’t too different; if they are, the averaged model will generate only colorful blobs.) The results were amusing. From early in training:
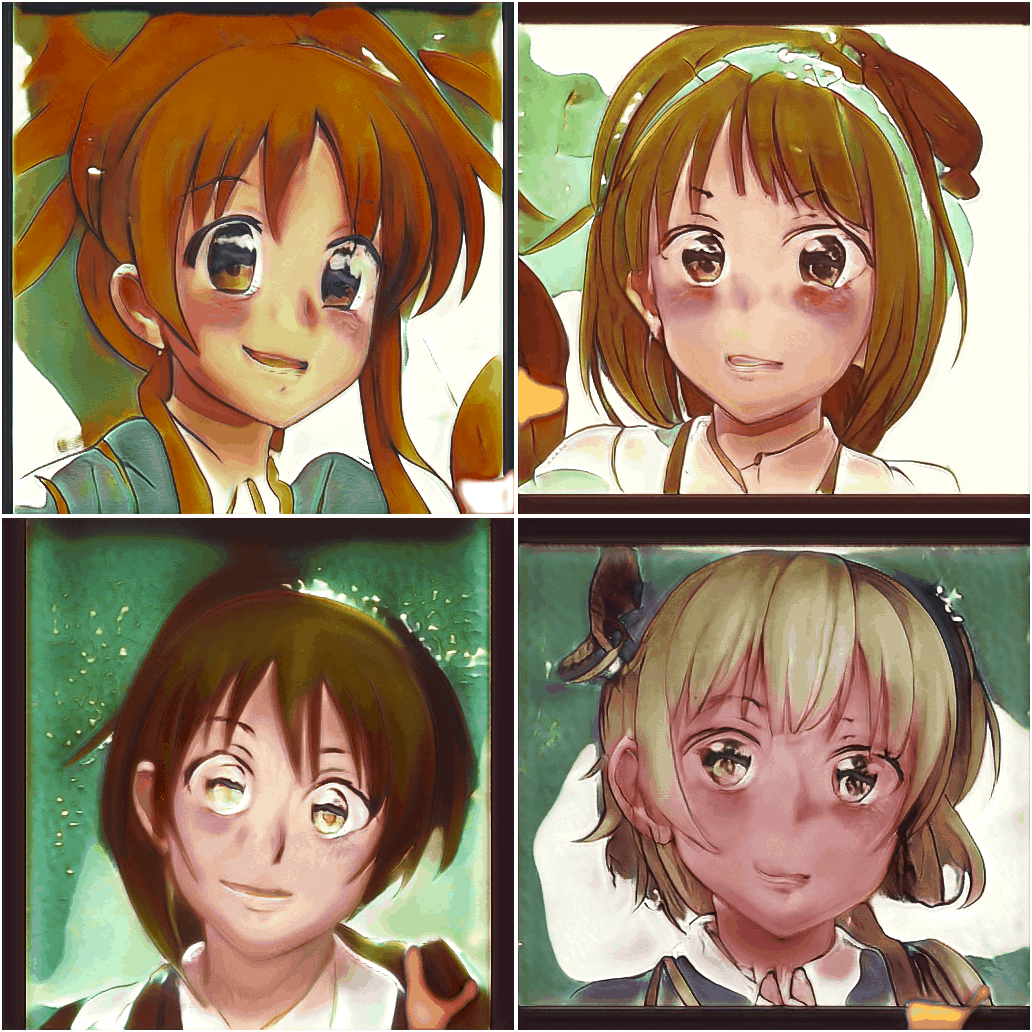
Later:
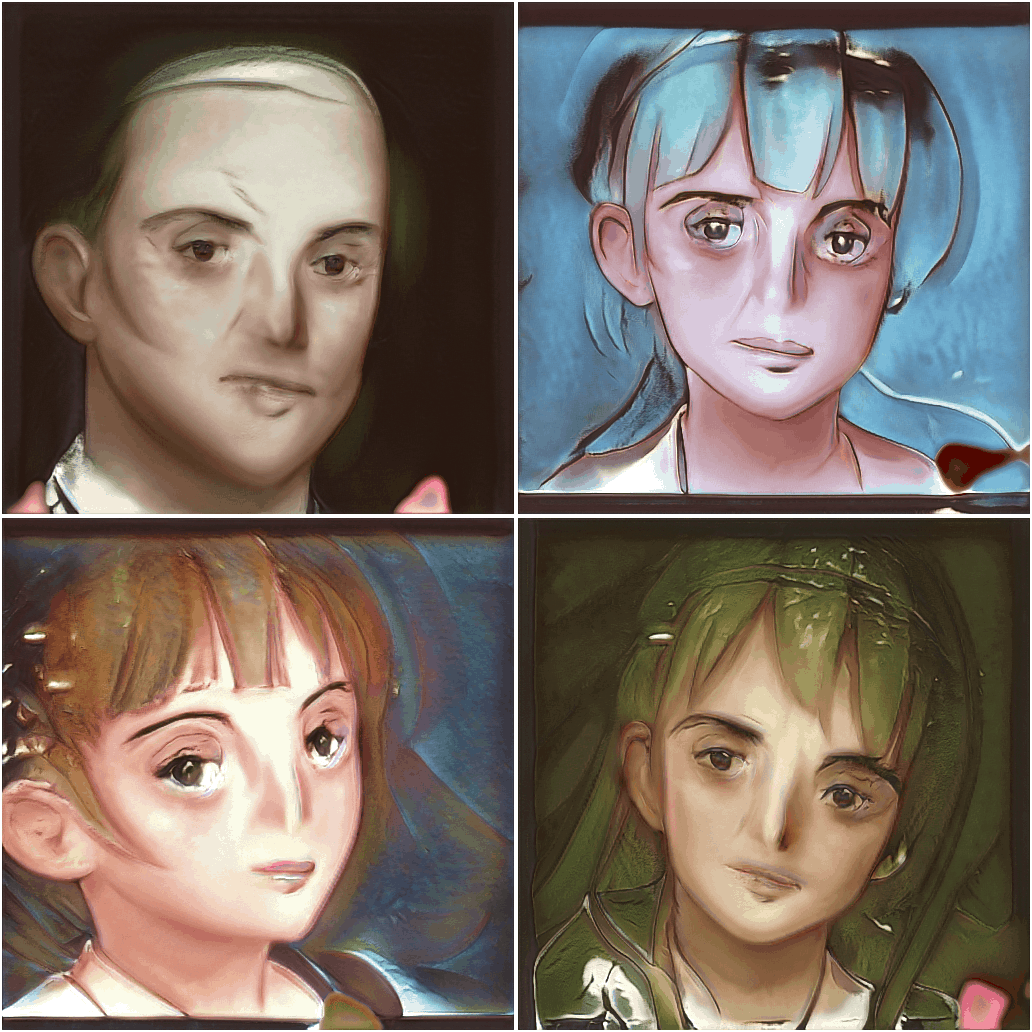
Anime Faces → Danbooru2018
nshepperd began a training run using an early anime face StyleGAN model on the 512px SFW Danbooru2018 subset; after ~3–5 weeks (with many interruptions) on 1 GPU, as of 2019-03-22, the training samples look like this:
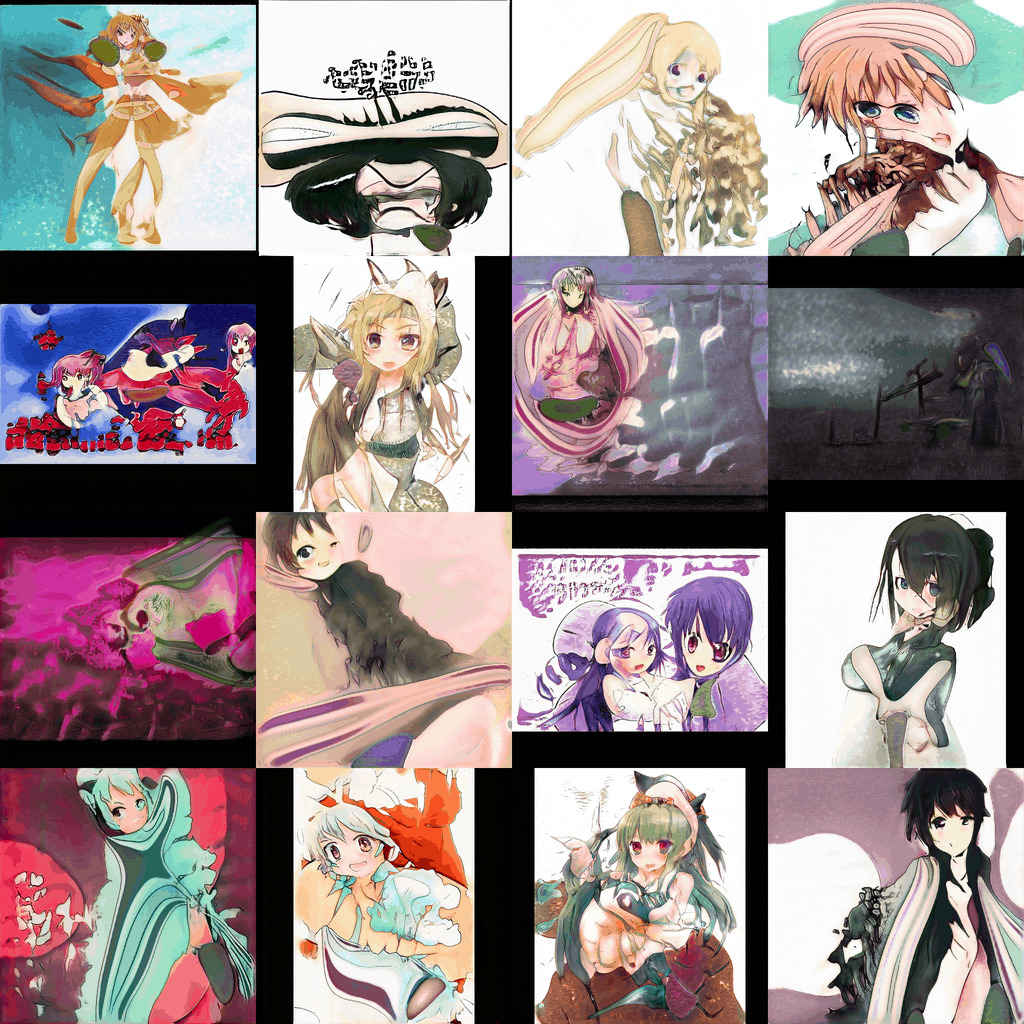
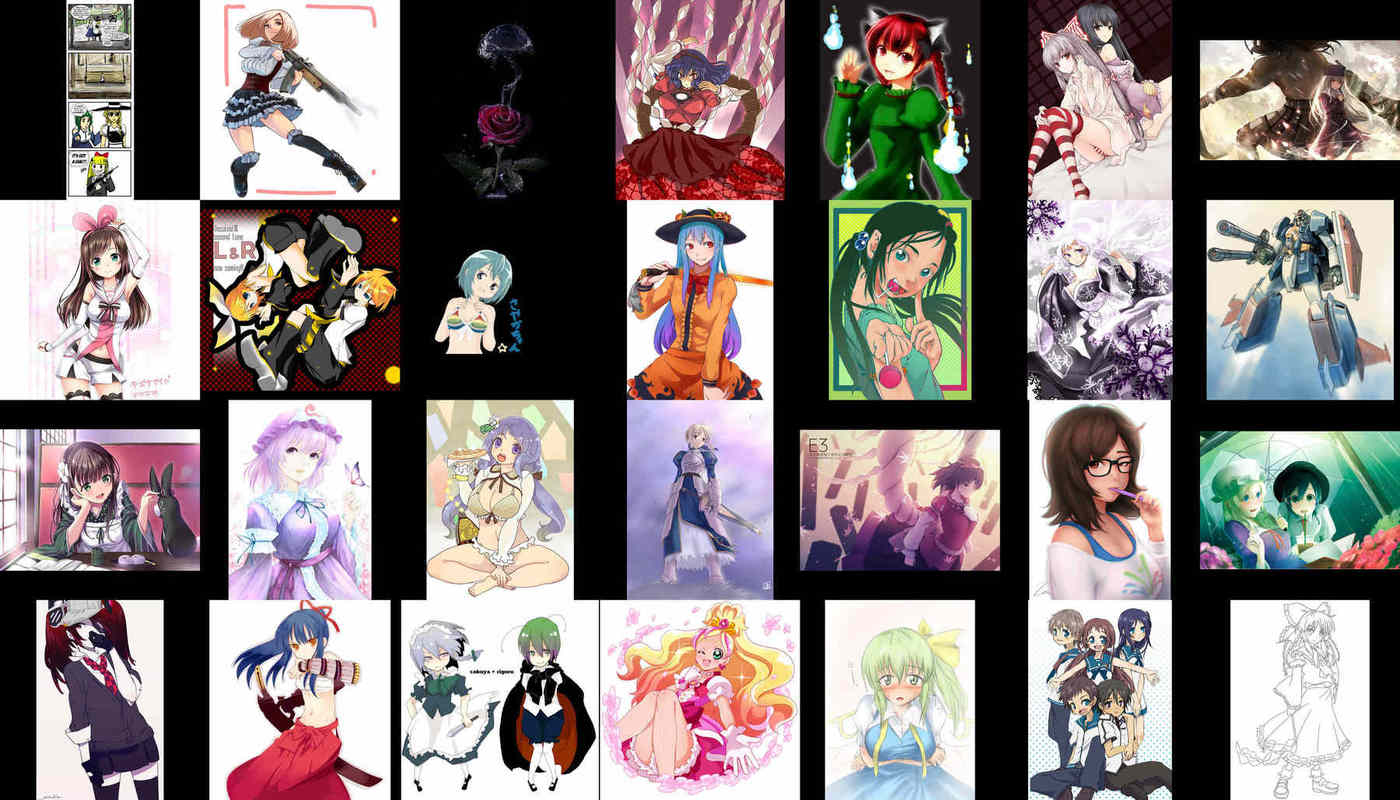
Training montage video of the Danbooru2018 model (up to #14204, 2019-03-22), trained by nshepperd
The StyleGAN is able to pick up global structure and there are recognizably anime figures, despite the sheer diversity of images, which is promising. The fine details are seriously lacking, and training, to my eye, is wandering around without any steady improvement or sharp details (except perhaps the faces which are inherited from the previous model). I suspect that the learning rate is still too high and, especially with only 1 GPU/n = 4, such small minibatches don’t cover enough modes to enable steady improvement. If so, the LR will need to be set much lower (or gradient accumulation used in order to fake having large minibatches where large LRs are stable) & training time extended to multiple months. Another possibility would be to restart with added self-attention layers, which I have noticed seem to particularly help with complicated details & sharpness; the style noise approach may be adequate for the job but just a few vanilla convolution layers may be too few (pace the BigGAN results on the benefits of increasing depth while decreasing parameter count).
FFHQ Variations
Anime Faces → FFHQ Faces
If StyleGAN can smoothly warp anime faces among each other and express global transforms like hair length+color with Ψ, could Ψ be a quick way to gain control over a single large-scale variable? For example, male vs female faces, or… anime ↔︎ real faces? (Given a particular image/latent vector, one would simply flip the sign to convert it to the opposite; this would give the opposite version of each random face, and if one had an encoder, one could do automatically anime-fy or real-fy an arbitrary face by encoding it into the latent vector which creates it, and then flipping.40)
Since Karras et al 2801 provide a nice FFHQ download script (albeit slower than I’d like once Google Drive rate-limits you a wallclock hour into the full download) for the full-resolution PNGs, it would be easy to downscale to 512px and create a 512px FFHQ dataset to train on, or even create a combined anime+FFHQ dataset.
The first and fastest thing was to do transfer learning from the anime faces to FFHQ real faces. It was unlikely that the model would retain much anime knowledge & be able to do morphing, but it was worth a try.
The initial results early in training are hilarious and look like zombies:
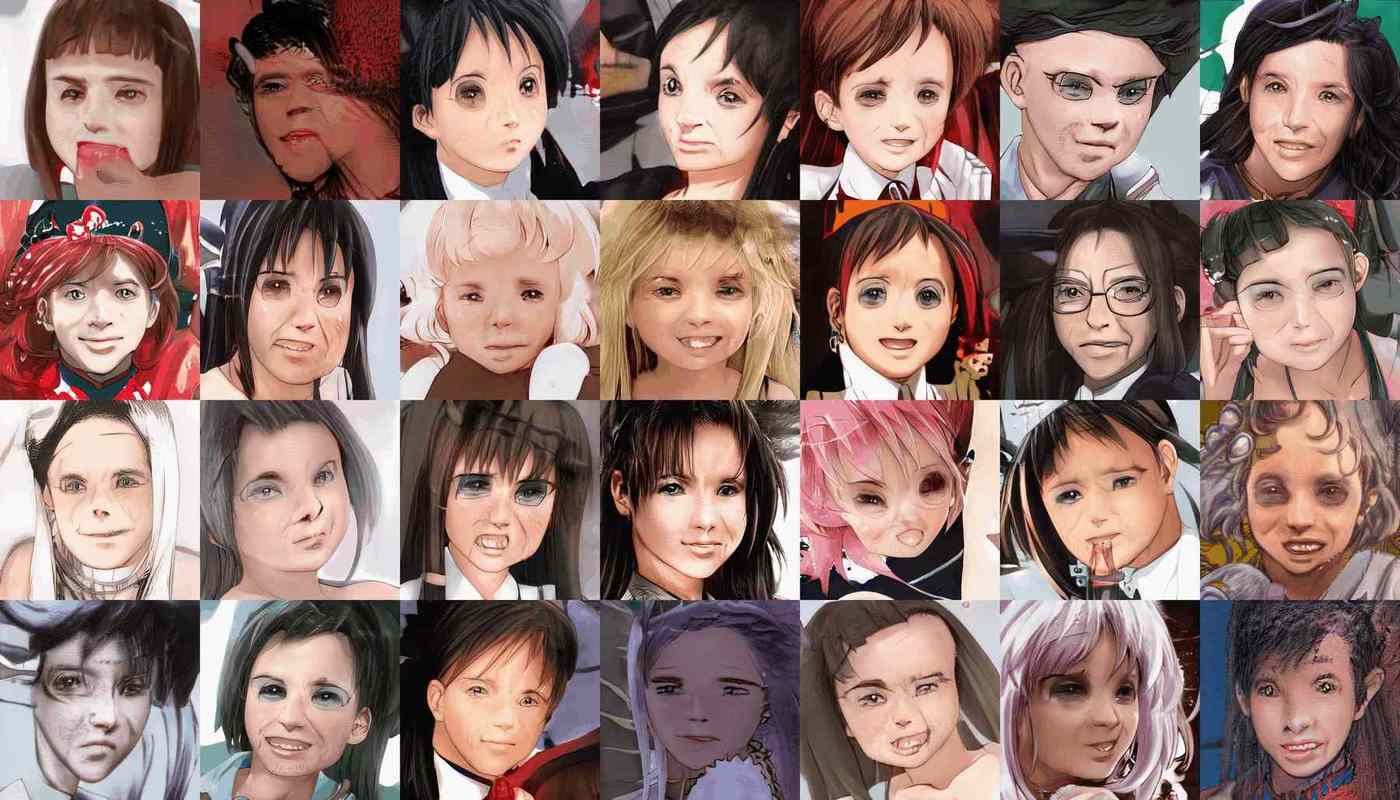
Interpolation video (4×4) of the FFHQ face model initialized from the anime face StyleGAN, a few ticks into training, showing bizarre artifacts
After 97 ticks, the model has converged to a boringly normal appearance, with the only hint of its origins being perhaps some excessively-fabulous hair in the training samples:
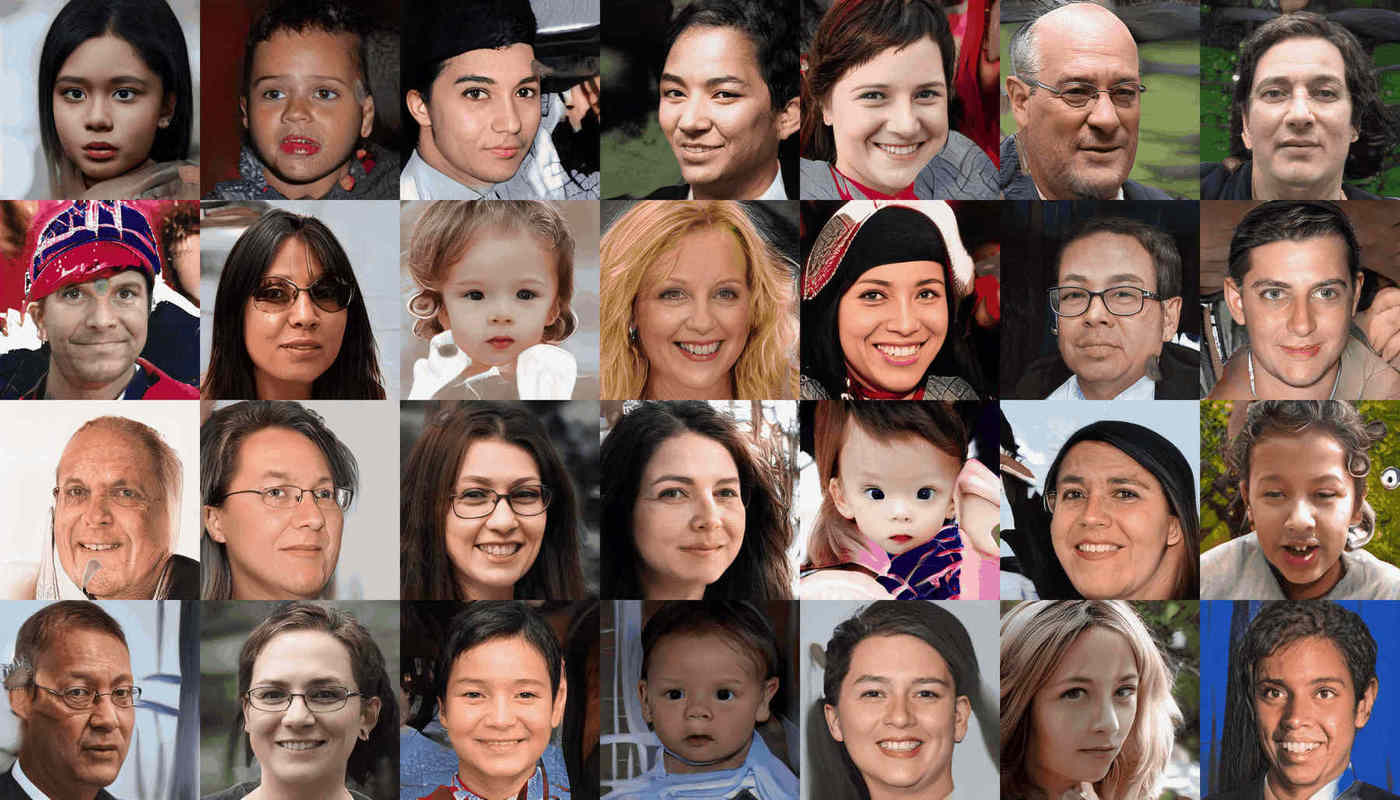
Anime Faces → Anime Faces + FFHQ Faces
So, that was a bust. The next step is to try training on anime & FFHQ faces simultaneously; given the stark difference between the datasets, would positive vs negative Ψ wind up splitting into real vs anime and provide a cheap & easy way of converting arbitrary faces?
This simply merged the 512px FFHQ faces with the 512px anime faces and resumed training from the previous FFHQ model (I reasoned that some of the anime-ness should still be in the model, so it would be slightly faster than restarting from the original anime face model). I trained it for 812 iterations, #11,359–12,171 (somewhat over 2 GPU-days), at which point it was mostly done.
It did manage to learn both kinds of faces quite well, separating them clearly in random samples:
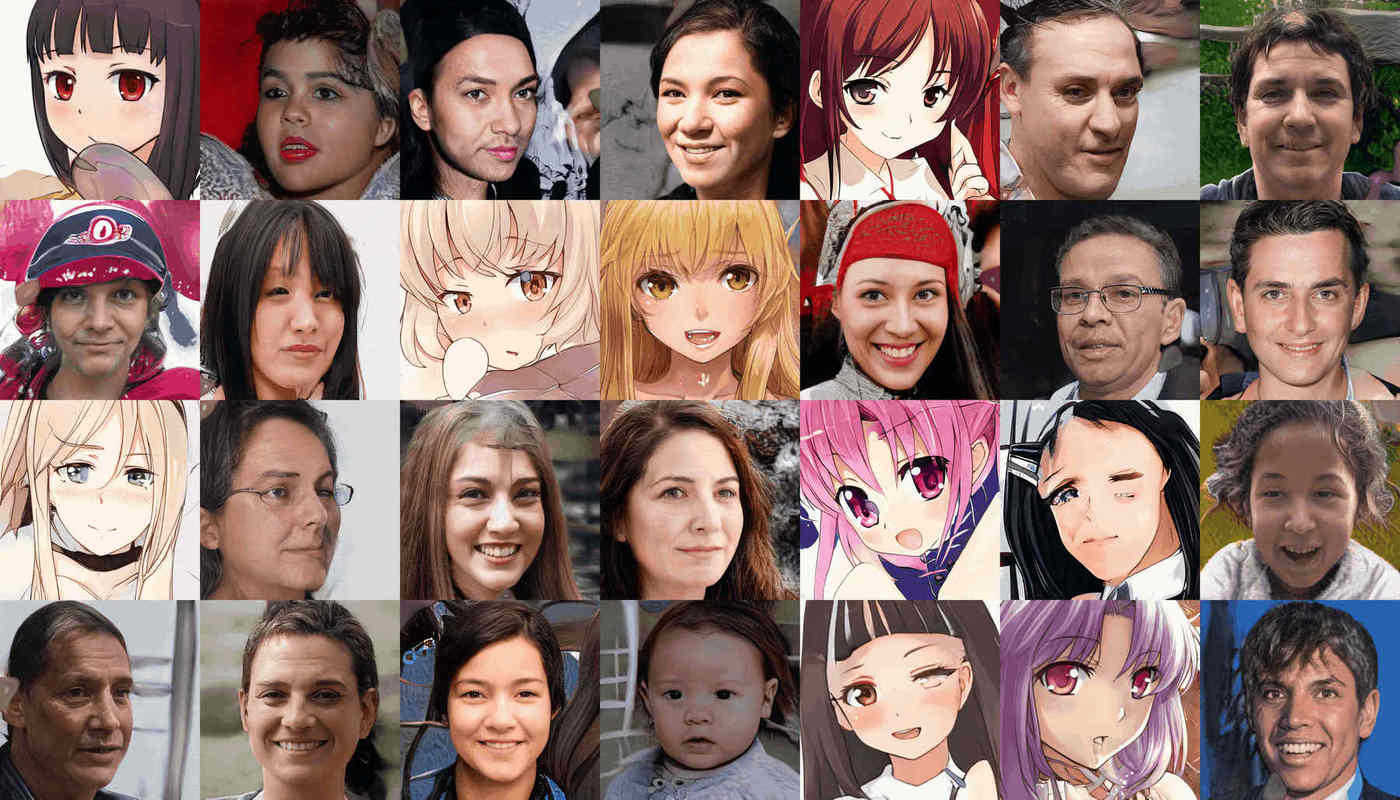
However, the style transfer & Ψ samples were disappointments. The style mixing shows limited ability to modify faces cross-domain or convert them, and the truncation trick chart shows no clear disentanglement of the desired factor (indeed, the various halves of Ψ correspond to nothing clear):
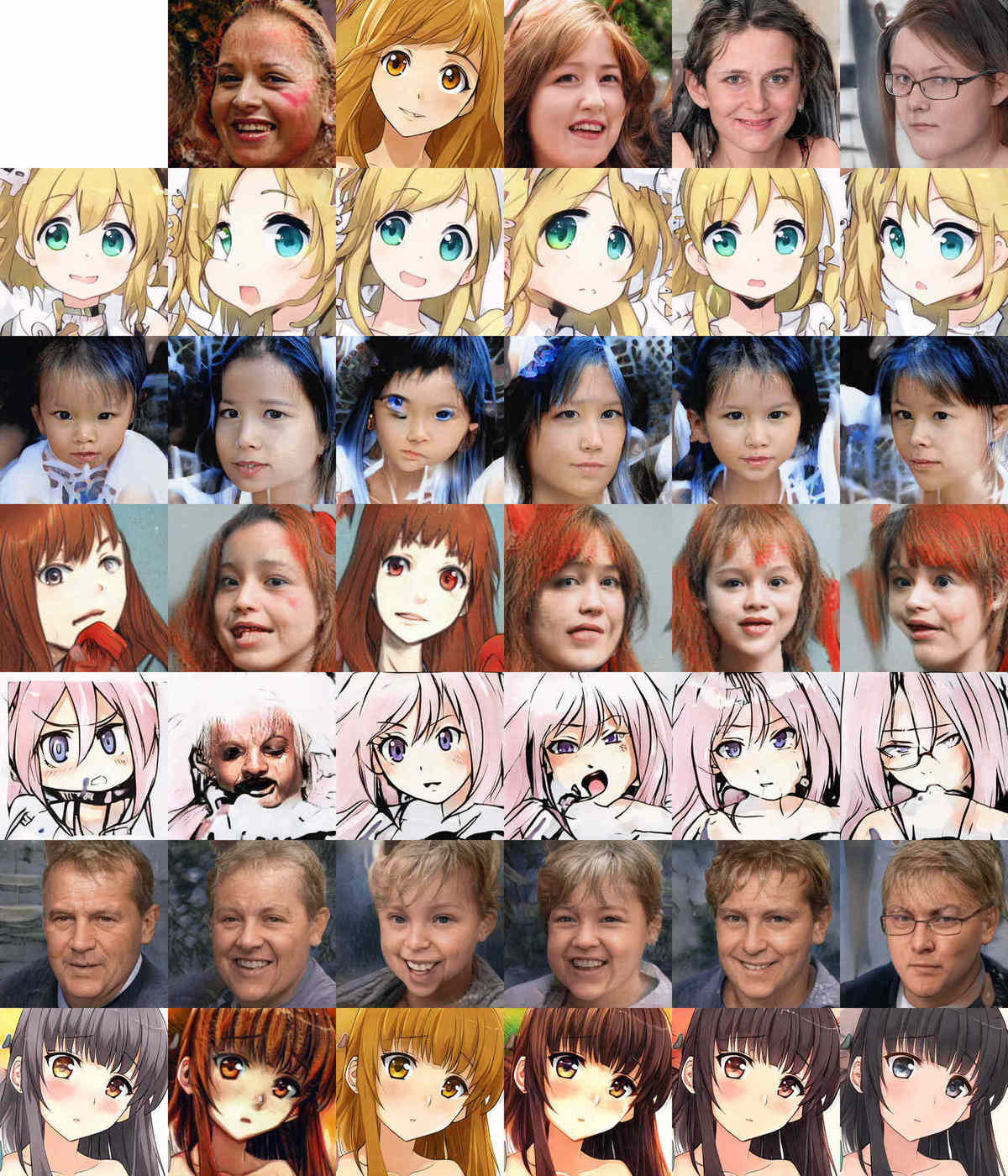
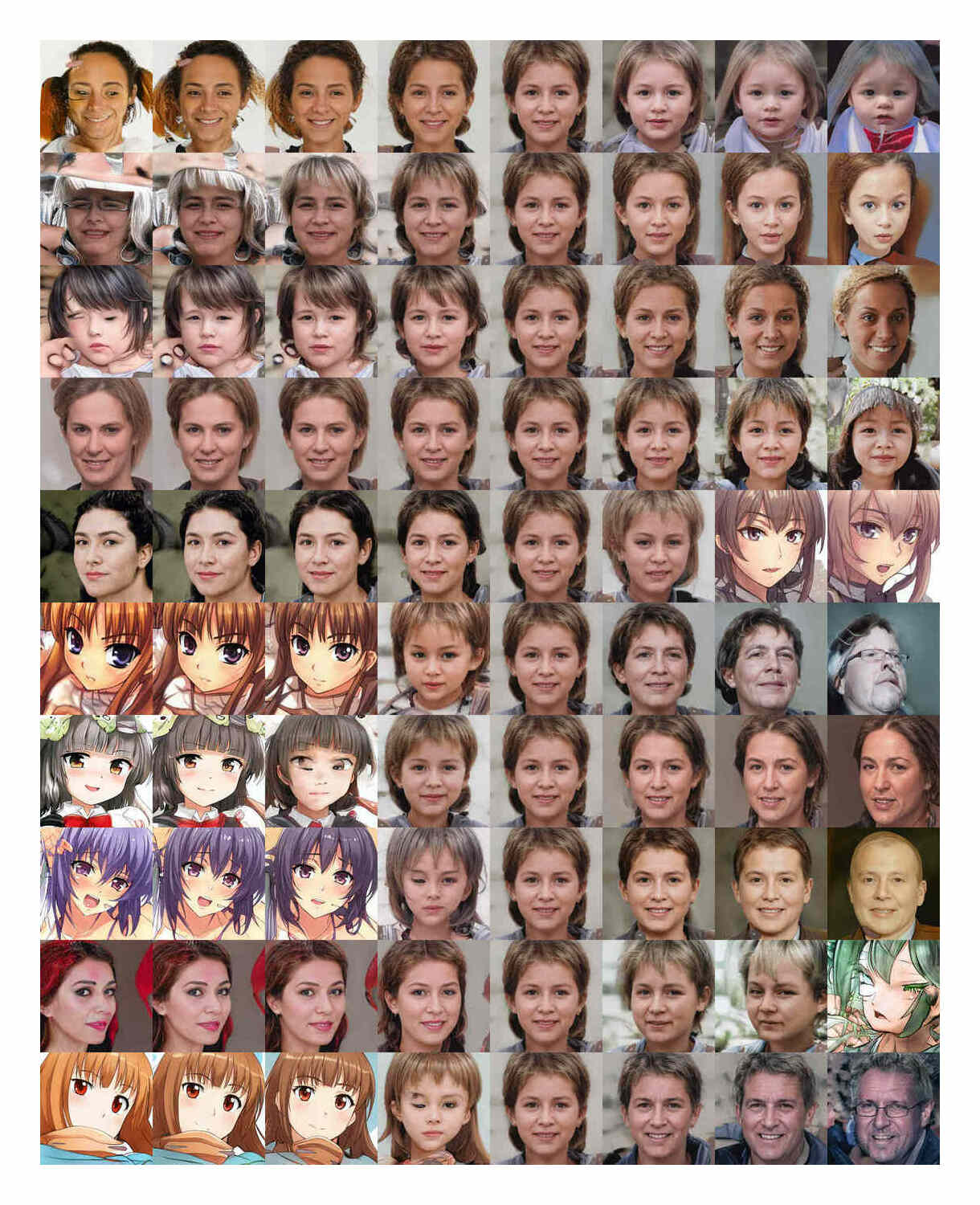
The interpolation video does show that it learned to interpolate slightly between real & anime faces, giving half-anime/half-real faces, but it looks like it only happens sometimes—mostly with young female faces41:
Interpolation video (4×4) of the FFHQ+anime face model, after convergence.
They’re hard to spot in the interpolation video because the transition happens abruptly, so I generated samples & selected some of the more interesting anime-ish faces:
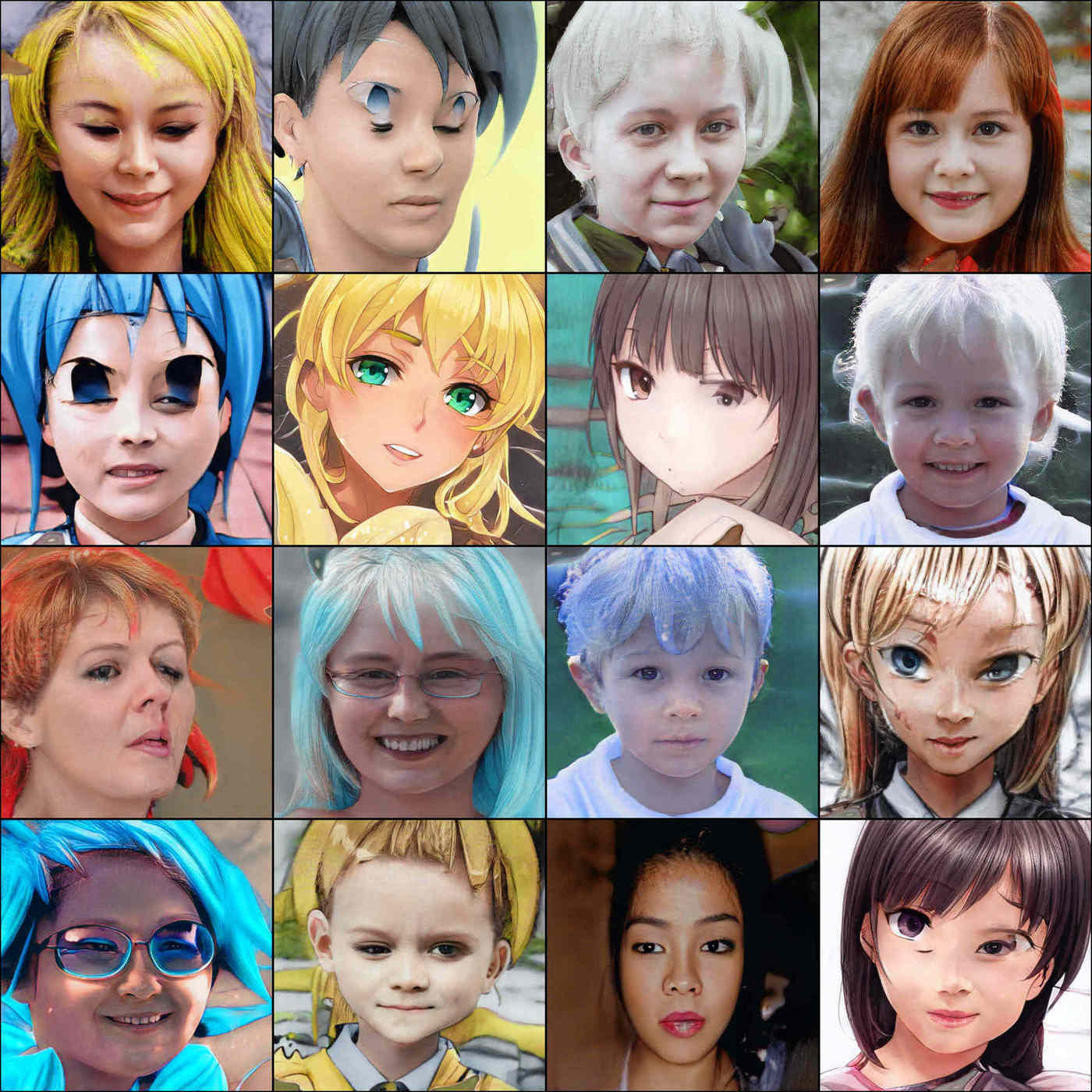
Similarly, Alexander Reben trained a StyleGAN on FFHQ+Western portrait illustrations, and the interpolation video is much smoother & more mixed, suggesting that more realistic & more varied illustrations are easier for StyleGAN to interpolate between.
Anime Faces + FFHQ → Danbooru2018
While I didn’t have the compute to properly train a Danbooru2018 StyleGAN, after nshepperd’s results, I was curious and spent some time (817 iterations, so ~2 GPU-days?) retraining the anime face+FFHQ model on Danbooru2018 SFW 512px images.
The training montage is interesting for showing how faces get repurposed into figures:
Training montage video of a Danbooru2018 StyleGAN initialized on an anime faces+FFHQ StyleGAN.
One might think that it is a bridge too far for transfer learning, but it seems not.
Reversing StyleGAN To Control & Modify Images
Discussion of how to modify existing images with GANs. There are several possibilities: train another NN to turn an image back into the original encoding; run blackbox search on encodings, repeatedly tweaking it to approximate a target face; or the whitebox approach, directly backpropagating through the model from the image to the encoding while holding the model fixed. All of these have been implemented for StyleGAN, and a combination works best. There are even GUIs for editing StyleGAN anime, pony, & furry faces!
Modifying images is harder than generating them. If we had a conditional anime face GAN like Arfafax’s, then we are fine, but if we have an unconditional architecture of some sort, then what? An unconditional GAN architecture is, by default, ‘one-way’: the latent vector z gets generated from a bunch of 𝒩(0,1) variables, fed through the GAN, and out pops an image. There is no way to run the unconditional GAN ‘backwards’ to feed in an image and pop out the z instead.42
If one could, one could take an arbitrary image and encode it into the z and by jittering z, generate many new versions of it; or one could feed it back into StyleGAN and play with the style noises at various levels in order to transform the image; or do things like ‘average’ two images or create interpolations between two arbitrary faces’; or one could (assuming one knew what each variable in z ‘means’) edit the image to changes things like which direction their head tilts or whether they are smiling.
There are some attempts at learning control in an unsupervised fashion (eg. 2020, GANSpace) but while excellent starting points, they have limits and may not find a specific control that one wants.
The most straightforward way would be to switch to a conditional GAN architecture based on a text or tag embedding. Then to generate a specific character wearing glasses, one simply says as much as the conditional input: "character glasses"
. Or if they should be smiling, add "smile"
. And so on. This would create images of said character with the desired modifications. This option is not available at the moment as creating a tag embedding & training StyleGAN requires quite a bit of modification. It also is not a complete solution as it wouldn’t work for the cases of editing an existing image.
For an unconditional GAN, there are two complementary approaches to inverting the G:
-
what one NN can learn to decode, another can learn to encode (eg. et al 2016 , 2019):
If StyleGAN has learned z→image, then train a second encoder NN on the supervised learning problem of image→z! The sample size is infinite (just keep running G) and the mapping is fixed (given a fixed G), so it’s ugly but not that hard.
-
backpropagate a pixel or feature-level loss to ‘optimize’ a latent code (eg. 2018):
While StyleGAN is not inherently reversible, it’s not a blackbox as, being a NN trained by backpropagation, it must admit of gradients. In training neural networks, there are 3 components: inputs, model parameters, and outputs/losses, and thus there are 3 ways to use backpropagation, even if we usually only use 1. One can hold the inputs fixed, and vary the model parameters in order to change (usually reduce) the fixed outputs in order to reduce a loss, which is training a NN; one can hold the inputs fixed and vary the outputs in order to change (often increase) internal parameters such as layers, which corresponds to neural network visualizations & exploration; and finally, one can hold the parameters & outputs fixed, and use the gradients to iteratively find a set of inputs which creates a specific output with a low loss (eg. optimize a wheel-shape input for rolling-efficiency output, or a Galton quinx/bean-machine for a tri-modal distribution, cf. et al 2018 ).43
This can be used to create images which are ‘optimized’ in some sense. For example, et al 2016 uses activation maximization, demonstrating how images of ImageNet classes can be pulled out of a standard CNN classifier by backprop over the classifier to maximize a particular output class; or redesign a fighter jet’s camouflage for easier classification by a model; more amusingly, in “Image Synthesis from Yahoo’s
open_nsfw
”, the gradient ascent44 on the individual pixels of an image is done to minimize/maximize a NSFW classifier’s prediction. (Other examples include horrifying rotating faces or minimizing face scores.) This can also be done on a higher level by trying to maximize similarity to a NN embedding of an image to make it as ‘similar’ as possible, as was done originally in et al 2014 for style transfer, or for more complicated kinds of style transfer like in “Differentiable Image Parameterizations: A powerful, under-explored tool for neural network visualizations and art”.In this case, given an arbitrary desired image’s z, one can initialize a random z, run it forward through the GAN to get an image, compare it at the pixel level with the desired (fixed) image, and the total difference is the ‘loss’; holding the GAN fixed, the backpropagation goes back through the model and adjusts the inputs (the unfixed z) to make it slightly more like the desired image. Done many times, the final z will now yield something like the desired image, and that can be treated as its true z. Comparing at the pixel-level can be improved by instead looking at the higher layers in a NN trained to do classification (often an ImageNet VGG), which will focus more on the semantic similarity (more of a “perceptual loss”) rather than misleading details of static & individual pixels. The latent code can be the original z, or z after it’s passed through the stack of 8 FC layers and has been transformed, or it can even be the various per-layer style noises inside the CNN part of StyleGAN; the last is what
style-image-prior
uses & 201945 argue that it works better to target the layer-wise encodings than the original z. The best approach as of 2021 for optimizing GANs is to use CLIP.This may not work too well as the local optima might be bad or the GAN may have trouble generating precisely the desired image no matter how carefully it is optimized, the pixel-level loss may not be a good loss to use, and the whole process may be quite slow, especially if one runs it many times with many different initial random z to try to avoid bad local optima. But it does mostly work.
-
Encode+Backpropagate is an useful hybrid strategy: the encoder makes its best guess at the z, which will usually be close to the true z, and then backpropagation is done for a few iterations to finetune the z. This can be much faster (one forward pass vs many forward+backward passes) and much less prone to getting stuck in bad local optima (since it starts at a good initial z thanks to the encoder).
Comparison with editing in flow-based models On a tangent, editing/reversing is one of the great advantages46 of ‘flow’-based NN models such as Glow, which is one of the families of NN models competitive with GANs for high-quality image generation (along with autoregressive pixel prediction models like PixelRNN, and VAEs). Flow models have the same shape as GANs in pushing a random latent vector z through a series of upscaling convolution or other layers to produce final pixel values, but flow models use a carefully-limited set of primitives which make the model runnable both forwards and backwards exactly. This means every set of pixels corresponds to an unique z and vice-versa, and so an arbitrary set of pixels can put in and the model run backwards to yield the exact corresponding z. There is no need to fight with the model to create an encoder to reverse it or use backpropagation optimization to try to find something almost right, as the flow model can already do this. This makes editing easy: plug the image in, get out the exact z with the equivalent of a single forward pass, figure out which part of z controls a desired attribute like ‘glasses’, change that, and run it forward. The downside of flow models, which is why I do not (yet) use them, is that the restriction to reversible layers means that they are typically much larger and slower to train than a more-or-less perceptually equivalent GAN model, by easily an order of magnitude (for Glow). When I tried Glow, I could barely run an interesting model despite aggressive memory-saving techniques, and I didn’t get anywhere interesting with the several GPU-days I spent (which was unsurprising when I realized how many GPU-months OA had spent). Since high-quality photorealistic GANs are at the limit of 2019 trainability for most researchers or hobbyists, flow models are clearly out of the question despite their many practical & theoretical advantages—they’re just too expensive! However, there is no known reason flow models couldn’t be competitive with GANs (they will probably always be larger, but because they are more correct & do more), and future improvements or hardware scaling may make them more viable, so flow-based models are an approach to keep an eye on.
One of those 3 approaches will encode an image into a latent z. So far so good, that enables things like generating randomly-different versions of a specific image or interpolating between 2 images, but how does one control the z in a more intelligent fashion to make specific edits?
If one knew what each variable in the z meant, one could simply slide them in the −1/+1 range, change the z, and generate the corresponding edited image. But there are 512 variables in z (for StyleGAN), which is a lot to examine manually, and their meaning is opaque as StyleGAN doesn’t necessarily map each variable onto a human-recognizable factor like ‘smiling’. A recognizable factor like ‘eyeglasses’ might even be governed by multiple variables simultaneously which are nonlinearly interacting.
As always, the solution to one model’s problems is yet more models; to control the z, like with the encoder, we can simply train yet another model (perhaps just a linear classifier or random forests this time) to take the z of many images which are all labeled ‘smiling’ or ‘not smiling’, and learn what parts of z cause ‘smiling’ (eg. et al 2019 ). These additional models can then be used to control a z. The necessary labels (a few hundred to a few thousand will be adequate since the z is only 512 variables) can be obtained by hand or by using a pre-existing classifier.
So, the pieces of the puzzle & putting it all together:
-
For anime faces as of March 2019, KichangKim’s DeepDanbooru is available as a service and as a downloadable Keras model, which provides tags for many traits.
Note that explicit classification/tagging may be overkill; if there is a mechanical way of controlling an attribute, direct control of the latents can be skipped. For example, an interpolation of mirroring a face can be done by taking a face+latent, mirroring it (using an encoder to get the mirror’s latents), and then simply linearly interpolating between the two sets of latents; since they differ only in orientation, their latents must also differ only in orientation, and interpolation=control.
-
An example of interactively controlling a CelebA face GAN in a convenient GUI is SummitKwan’s TL-GAN (Kaggle interactive demo, discussion).
-
Dmitry Nikitko has written a StyleGAN encoder (discussion; alternative encoder with additional losses, using resnets) using the backpropagation approach on ImageNet VGG features (but not a direct encoder)
He has trained 3 classification models for age/gender/smiling, and so can do things like edit Donald Trump or Hillary Clinton photos to smile.
-
snowy halcy has reused the encoder with the VGG loss+Discriminator loss, linear models trained on DeepDanbooru tags from n = 6.5k, allowing control of generated anime faces. (A later independent implementation of backpropagation-only was done by et al 2019 .) Some of the encodings work well on solo faces & others don’t (on multiple faces), so sticking close to anime face StyleGAN samples is advised. Links:
-
videos: transforming into red-eyed/black-haired versions (image; brightness editing), TL-GAN GUI demonstration of general editing, version 2 face editing
-
mouth flap control: Kyle McLean’s “Waifu Synthesis” video & Aiterasu repo (demos: 1, 2), which use the Halcy tagger to open/close mouths, allowing for lip-syncinggw ’ar
-
Artbreeder also implements face editing for my anime StyleGAN (demo video) along with a number of other models such as Western art portraits
-
the This Fursona Does Not Exist model can be used to edit furry faces through GANspace (as presumably can “This Pony Does Not Exist” for pony faces)
-
GANSpace (et al 2020 ) is a semi-automated approach to discovering useful latent vector controls: it tries to find ‘large’ changes in images, under the assumption those correspond to interesting disentangled factors. A human tweaking the layers it uses and which ones are selected can find interesting (eg. a “stoned” face vector in FFHQ StyleGAN), and it can be used in Colab.
The final result is interactive editing of anime faces along many different factors:
snowy halcy (MP4) demonstrating interactive editing of StyleGAN anime faces using anime-face-StyleGAN+DeepDanbooru+StyleGAN-encoder+TL-GAN
Editing Rare Attributes
A strategy of hand-editing or using a tagger to classify attributes works for common ones which will be well-represented in a sample of a few thousand since the classifier needs a few hundred cases to work with, but what about rarer attributes which might appear only on one in a thousand random samples, or attributes too rare in the dataset for StyleGAN to have learned, or attributes which may not be in the dataset at all? Editing “red eyes” should be easy, but what about something like “bunny ears”? It would be amusing to be able to edit portraits to add bunny ears, but there aren’t that many bunny ear samples (although cat ears might be much more common); is one doomed to generate & classify hundreds of thousands of samples to enable bunny ear editing? That would be infeasible for hand labeling, and difficult even with a tagger.
One suggestion I have for this use-case would be to briefly train another StyleGAN model on an enriched or boosted dataset, like a dataset of 50:50 bunny ear images & normal images. If one can obtain a few thousand bunny ear images, then this is adequate for transfer learning (combined with a few thousand random normal images from the original dataset), and one can retrain the StyleGAN on an equal balance of images. The high presence of bunny ears will ensure that the StyleGAN quickly learns all about those, while the normal images prevent it from overfitting or catastrophic forgetting of the full range of images.
This new bunny-ear StyleGAN will then produce bunny-ear samples half the time, circumventing the rare base rate issue (or failure to learn, or nonexistence in dataset), and enabling efficient training of a classifier. And since normal faces were used to preserve its general face knowledge despite the transfer learning potentially degrading it, it will remain able to encode & optimize normal faces. (The original classifiers may even be reusable on this, depending on how extreme the new attribute is, as the latent space z might not be too affected by the new attribute and the various other attributes approximately maintain the original relationship with z as before the retraining.)
StyleGAN 2
How to use StyleGAN2, an improvement to StyleGAN released in December 2019, which removes the blob artifacts and is generally of somewhat higher visual quality.
StyleGAN 2 is tricky to use because it requires custom local compilation of optimized code. Aaron Gokaslan provided tips on getting StyleGAN 2 running and trained a StyleGAN 2 on my anime portraits, which is available for download and which I use to create TWDNEv3.
StyleGAN 2 (source (PyTorch), video), eliminates blob artifacts, adds a native encoding ‘projection’ feature for editing, simplifies the runtime by scrapping progressive growing in favor of MSG-GAN-like multi-scale architecture, & has higher overall quality—but similar total training time/requirements47
I used a 512px anime portrait S2 model trained by Aaron Gokaslan to create ThisWaifuDoesNotExist v3:
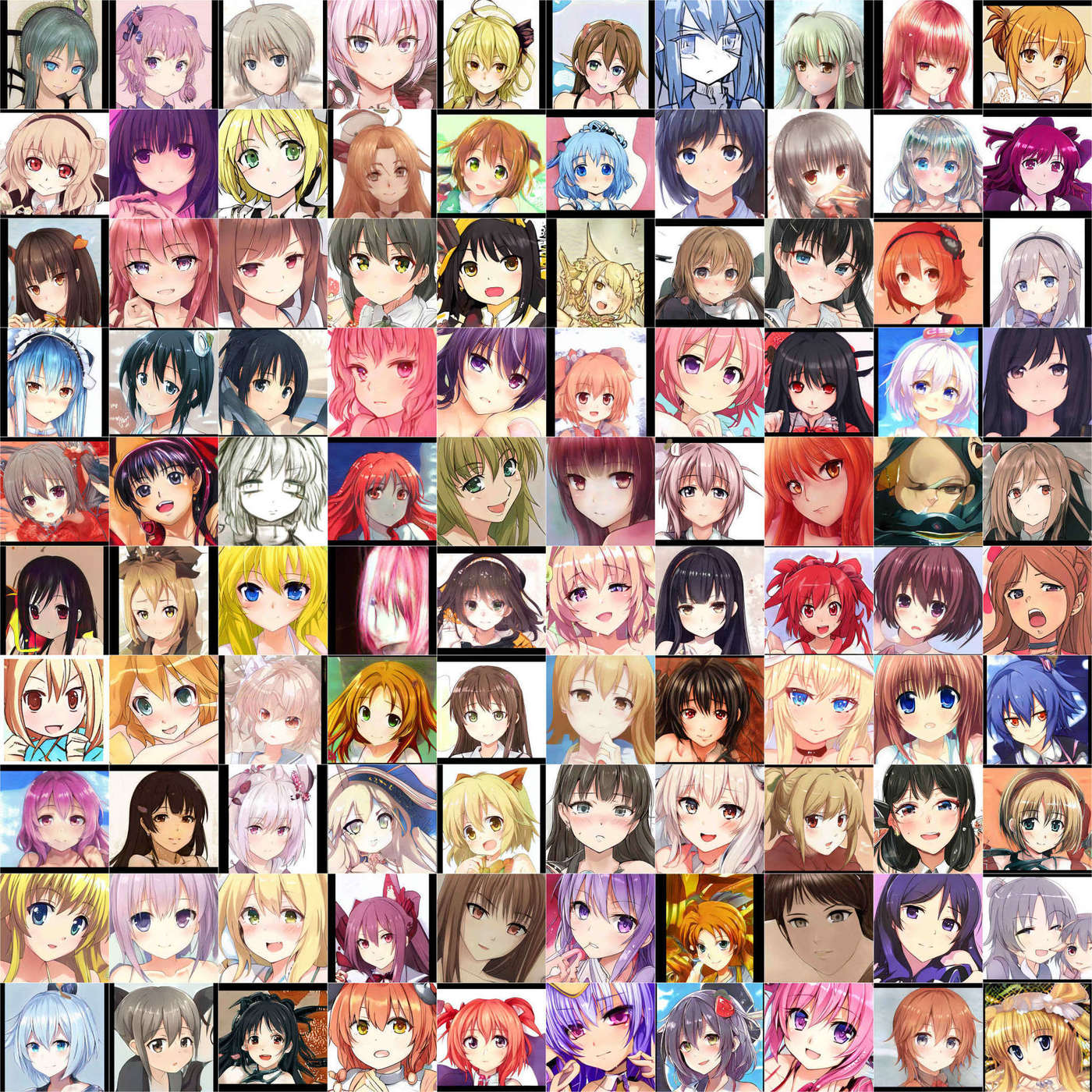
Training samples:
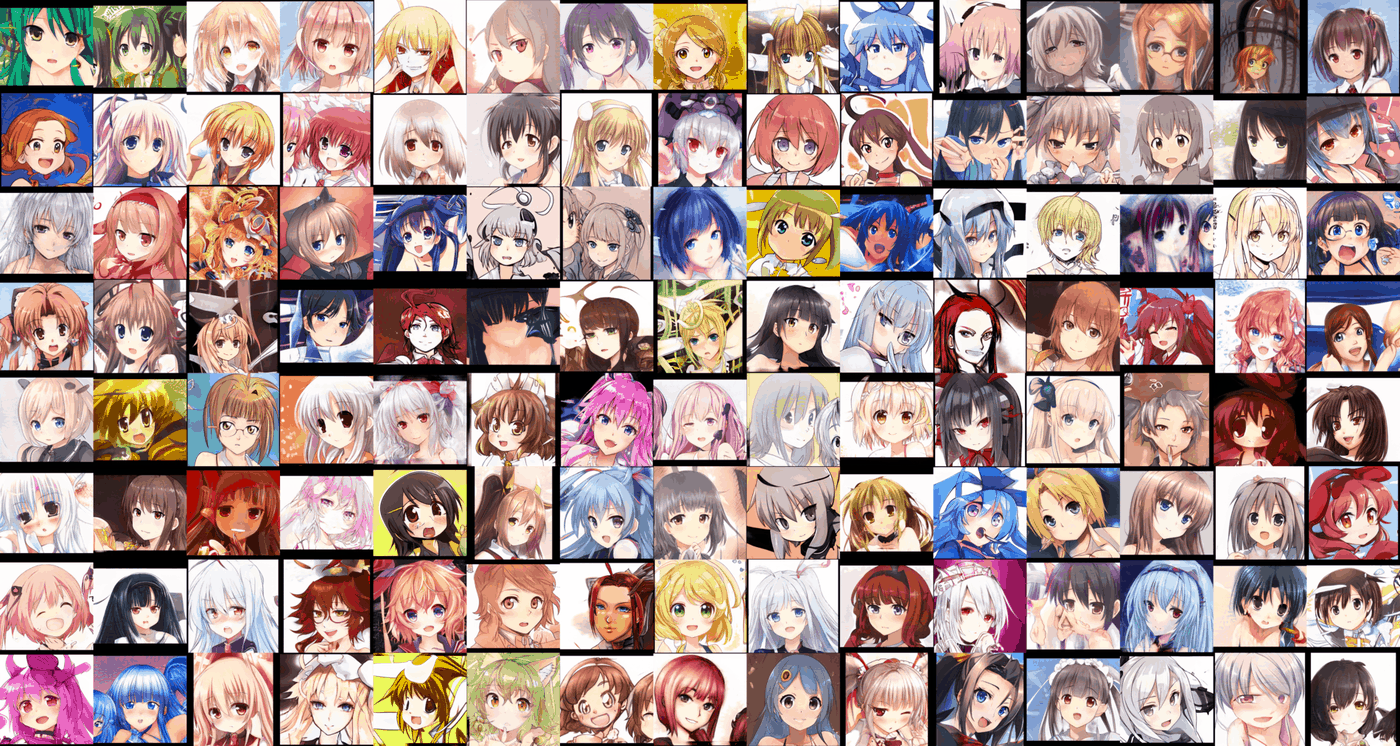
The model was trained to iteration #24,664 for >2 weeks on 4 Nvidia 2080ti GPUs at 35–70s per 1k images. The Tensorflow S2 model is available for download (320MB).48 (PyTorch & Onnx versions have been made by Anton using a custom repo Note that both my face & portrait models can be run via the GenForce PyTorch repo as well.) This model can be used in Google Colab (demonstration notebook, although it seems it may pull in an older S2 model) & the model can also be used with the S2 codebase for encoding anime faces.
Running S2
Because of the optimizations, which requires custom local compilation of CUDA code for maximum efficiency, getting S2 running can be more challenging than getting S1 running.
-
No TensorFlow 2 compatibility: the TF version must be 1.14/1.15. Trying to run with TF 2 will give errors like:
TypeError: int() argument must be a string, a bytes-like object or a number, not 'Tensor'
.I ran into cuDNN compatibility problems with TF 1.15 (which requires cuDNN >7.6.0, 2019-05-20, for CUDA 10.0), which gave errors like this:
...[2020-01-11 23:10:35.234784: E tensorflow/stream_executor/cuda/cuda_dnn.cc:319] Loaded runtime CuDNN library: 7.4.2 but source was compiled with: 7.6.0. CuDNN library major and minor version needs to match or have higher minor version in case of CuDNN 7.0 or later version. If using a binary install, upgrade your CuDNN library. If building from sources, make sure the library loaded at runtime is compatible with the version specified during compile configuration...
But then with 1.14, the
tpu-estimator
library was not found! (I ultimately took the risk of upgrading my installation withlibcudnn7_7.6.0.64-1+cuda10.0_amd64.deb
, and thankfully, that worked and did not seem to break anything else.) -
Getting the entire pipeline to compile the custom ops in a Conda environment was annoying so Gokaslan tweaked it to use 1.14 on Linux, used
cudatoolkit-dev
from Conda Forge, and changed the build script to usegcc-7
(sincegcc-8
was unsupported) -
one issue with TensorFlow 1.14 is you need to force
allow_growth
or it will error out on Nvidia 2080tis -
config name change:
train.py
has been renamed (again) torun_training.py
-
buggy learning rates: S2 (but not S1) accidentally uses the same LR for both G & D; either fix this or keep it in mind when doing LR tuning—changes to
D_lrate
do nothing! -
n = 1 minibatch problems: S2 is not a large NN so it can be trained on low-end GPUs; however, the S2 code make an unnecessary assumption that n≥2; to fix this in
training/loss.py
(fixed in Shawn Presser’s TPU/self-attention oriented fork):@@ -157,9 +157,8 @@ def G_logistic_ns_pathreg(G, D, opt, training_set, minibatch_size, pl_minibatch_ with tf.name_scope('PathReg'): # Evaluate the regularization term using a smaller minibatch to conserve memory. if pl_minibatch_shrink > 1 and minibatch_size > 1: assert minibatch_size % pl_minibatch_shrink == 0 pl_minibatch = minibatch_size // pl_minibatch_shrink if pl_minibatch_shrink > 1: pl_minibatch = tf.maximum(1, minibatch_size // pl_minibatch_shrink) pl_latents = tf.random_normal([pl_minibatch] + G.input_shapes[0][1:]) pl_labels = training_set.get_random_labels_tf(pl_minibatch) fake_images_out, fake_dlatents_out = G.get_output_for(pl_latents, pl_labels, is_training=True, return_dlatents=True)
-
S2 has some sort of memory leak, possibly related to the FID evaluations, requiring regular restarts, like putting it into a loop
Once S2 was running, Gokaslan trained the S2 portrait model with generally default hyperparameters.
Future Work
Some open questions about StyleGAN’s architecture & training dynamics:
-
is progressive growing still necessary with StyleGAN? (StyleGAN 2 implies that it is not, as it uses a MSG-GAN-like approach)
-
are 8×512 FC layers necessary? (Preliminary BigGAN work suggests that they are not necessary for BigGAN.)
-
what are the wrinkly-line/cracks noise artifacts which appear at the end of training?
-
how does StyleGAN compare to BigGAN in final quality?
Further possible work:
-
exploration of “curriculum learning”: can training be sped up by training to convergence on small n and then periodically expanding the dataset?
-
bootstrapping image generation by starting with a seed corpus, generating many random samples, selecting the best by hand, and retraining; eg. expand a corpus of a specific character, or explore ‘hybrid’ corpuses which mix A/B images & one then selects for images which look most A+B-ish
-
improved transfer learning scripts to edit trained models so 512px pretrained models can be promoted to work with 1024px images and vice versa
-
better Danbooru tagger CNN for providing classification embeddings for various purposes, particularly FID loss monitoring, minibatch discrimination/auxiliary loss, and style transfer for creating a ‘StyleDanbooru’
-
with a StyleDanbooru, I am curious if that can be used as a particularly Powerful Form Of Data Augmentation for small n character datasets, and whether it leads to a reversal of training dynamics with edges coming before colors/textures—it’s possible that a StyleDanbooru could make many GAN architectures, not just StyleGAN, stable to train on anime/illustration datasets
-
-
borrowing architectural enhancements from BigGAN: self-attention layers, spectral norm regularization, large-minibatch training, and a rectified Gaussian distribution for the latent vector z
-
text → image conditional GAN architecture (à la StackGAN):
This would take the text tag descriptions of each image compiled by Danbooru users and use those as inputs to StyleGAN, which, should it work, would mean you could create arbitrary anime images simply by typing in a string like
1_boy samurai facing_viewer red_hair clouds sword armor blood
etc.This should also, by providing rich semantic descriptions of each image, make training faster & stabler and converge to higher final quality.
-
meta-learning for few-shot face or character or artist imitation (eg. Set-CGAN or FIGR or perhaps FUNIT, or 2019—the last of which achieves few-shot learning with samples of n = 25 TWDNE StyleGAN anime faces)
ImageNet StyleGAN
As part of experiments in scaling up StyleGAN 2, using TRC research credits, we ran StyleGAN on large-scale datasets including Danbooru2019, ImageNet, and subsets of the Flickr YFCC100M dataset. Despite running for millions of images, no S2 run ever achieved remotely the realism of S2 on FFHQ or BigGAN on ImageNet: while the textures could be surprisingly good, the semantic global structure never came together, with glaring flaws—there would be too many heads, or they would be detached from bodies, etc.
Aaron Gokaslan took the time to compute the FID on ImageNet, estimating a terrible score of FID ~120. (Higher=worse; for comparison, BigGAN with EvoNorm can be as good as FID ~7, and regular BigGAN typically surpasses FID 120 within a few thousand iterations.) Even experiments in increasing the S2 model size up to ~1GB (by increasing the feature map multiplier) improved quality relatively modestly, and showed no signs of ever approaching BigGAN-level quality. We concluded that StyleGAN is in fact fundamentally limited as a GAN, trading off stability for power, and switched over to BigGAN work.
For those interested, we provide our 512px ImageNet S2 (step 1,394,688):
rsync --verbose rsync://176.9.41.242:873/biggan/2020-04-07-shawwn-stylegan-imagenet-512px-run52-1394688.pkl.xz ./
Shawn Presser, S2 ImageNet interpolation video from partway through training (~45 hours on a TPUv3-512, 3k images/s)
BigGAN
See Also
External Links
-
“Pretrained Anime StyleGAN 2—convert to Pytorch and editing images by encoder”, Allen Ng
-
“Video shows off hundreds of beautiful AI-created anime girls in less than a minute【Video】”
-
“Talking Head Anime from a Single Image”, Pramook Khungurn
-
“StyleGAN in WebAssembly using tensor4”, Stanislav Pidhorskyi (code)
-
“StyleGAN2: Align, Project, Animate, Mix Styles and Train”, Nikhil Tirumala (“easy-to-use utility functions to generate animations and mix styles”)
-
“StyleGAN v2: notes on training and latent space exploration”, Alex Martinelli
-
“Practical aspects of StyleGAN2 training”/“Scaling up StyleGAN2”, l4rz (improving topless image generation by hyperparameter tuning, model size increase, & face-cropping data augmentation)
-
“Deep Generative Modelling: A Comparative Review of VAEs, GANs, Normalizing Flows, Energy-Based and Autoregressive Models”, Bond-et al 2021
-
“State-of-the-Art Image Generative Models”, Aran Komatsuzaki; “Generative Modeling by Estimating Gradients of the Data Distribution”
-
Discussion: Reddit
Appendix
For failed anime experiments, see Anime Neural Net Graveyard
-
Turns out that when training goes really wrong, you can crash many GAN implementations with either a segfault, integer overflow, or division by zero error.↩︎
-
StackGAN/StackGAN++/PixelCNN et al are difficult to run as they require an unique image embedding which could only be computed in the unmaintained Torch framework using Reed’s prior work on a joint text+image embedding which however doesn’t run on anything but the Birds & Flowers datasets, and so no one has ever, as far as I am aware, run those implementations on anything else—certainly I never managed to despite quite a few hours trying to reverse-engineer the embedding & various implementations.↩︎
-
Be sure to check out Artbreeder.↩︎
-
Glow’s reported results required >40 GPU-weeks; BigGAN’s total compute is unclear as it was trained on a TPUv3 Google cluster but it would appear that a 128px BigGAN might be ~4 GPU-months assuming hardware like an 8-GPU machine, 256px ~8 GPU-months, and 512px ≫8 GPU-months, with VRAM being the main limiting factor for larger models (although progressive growing might be able to cut those estimates).↩︎
-
illustration2vec
is an old & small CNN trained to predict a few -booru tags on anime images, and so provides an embedding—but not a good one. The lack of a good embedding is the major limitation for anime deep learning as of February 2019. (DeepDanbooru, while performing well apparently, has not yet been used for embeddings.) An embedding is necessary for text → image GANs, image searches & nearest-neighbor checks of overfitting, FID errors for objectively comparing GANs, minibatch discrimination to help the D/provide an auxiliary loss to stabilize learning, anime style transfer (both for its own sake & for creating a ‘StyleDanbooru2018’ to reduce texture cheating), encoding into GAN latent spaces for manipulation, data cleaning (to detect anomalous datapoints like failed face crops), perceptual losses for encoders or as an additional auxiliary loss/pretraining (like “NoGAN”, which trains a Generator on a perceptual loss and does GAN training only for finetuning) etc. A good tagger is also a good starting point for doing pixel-level semantic segmentation (via “weak supervision”, with Transformers working especially well), which metadata is key for training something like Nvidia’s GauGAN successor to pix2pix (et al 2019 ; source).↩︎ -
Technical note: I typically train NNs using my workstation with 2×1080ti GPUs. For easier comparison, I convert all my times to single-GPU equivalent (ie. “6 GPU-weeks” means 3 realtime/wallclock weeks on my 2 GPUs).↩︎
-
et al 2019 observes (§4 “Using precision and recall to analyze and improve StyleGAN”) that StyleGAN with progressive growing disabled does work but at some cost to precision/recall quality metrics; whether this reflects inferior performance on a given training budget or an inherent limit—BigGAN and other self-attention-using GANs do not use progressive growing at all, suggesting it is not truly necessary—is not investigated. In December 2019, StyleGAN 2 successfully dropped progressive growing entirely at modest performance cost.↩︎
-
This has confused some people, so to clarify the sequence of events: I trained my anime face StyleGAN and posted notes on Twitter, releasing an early model; roadrunner01 generated an interpolation video using said model (but a different random seed, of course); this interpolation video was retweeted by the Japanese Twitter user _Ryobot, upon which it went viral and was ‘liked’ by Elon Musk, further driving virality (19k reshares, 65k likes, 1.29m watches as of 2019-03-22).↩︎
-
Google Colab is a free service includes free GPU time (up to 12 hours on a small GPU). Especially for people who do not have a reasonably capable GPU on their personal computers (such as all Apple users) or do not want to engage in the admitted hassle of renting a real cloud GPU instance, Colab can be a great way to play with a pretrained model, like generating GPT-2-117M text completions or StyleGAN interpolation videos, or prototype on tiny problems.
However, it is a bad idea to try to train real models, like 512–1024px StyleGANs, on a Colab instance as the GPUs are low VRAM, far slower (6 hours per StyleGAN tick!), unwieldy to work with (as one must save snapshots constantly to restart when the session runs out), doesn’t have a real command-line, etc. Colab is just barely adequate for perhaps 1 or 2 ticks of transfer learning, but not more. If you harbor greater ambitions but still refuse to spend any money (rather than time), Kaggle has a similar service with P100 GPU slices rather than K80s. Otherwise, one needs to get access to real GPUs.↩︎
-
Curiously, the benefit of many more FC layers than usual may have been stumbled across before: IllustrationGAN found that adding some FC layers seemed to help their DCGAN generate anime faces, and when I & FeepingCreature experimented with adding 2–4 FC layers to WGAN-GP along IllustrationGAN’s lines, it did help our lackluster results, and at the time I speculated that “the fully-connected layers are transforming the latent-z/noise into a sort of global template which the subsequent convolution layers can then fill in more locally.” But we never dreamed of going as deep as 8!↩︎
-
The ProGAN/StyleGAN codebase reportedly does work with conditioning, but none of the papers report on this functionality and I have not used it myself.↩︎
-
The latent embedding z is usually generated in about the simplest possible way: draws from the Normal distribution, 𝒩(0,1). A Uniform(−1,1) is sometimes used instead. There is no good justification for this and some reason to think this can be bad (how does a GAN easily map a discrete or binary latent factor, such as the presence or absence of the left ear, onto a Normal variable?).
The BigGAN paper explores alternatives, finding improvements in training time and/or final quality from using instead (in ascending order): a Normal + binary Bernoulli (p = 0.5; personal communication, Brock) variable, a binary (Bernoulli), and a Rectified Gaussian (sometimes called a “censored normal” even though that sounds like a truncated normal distribution rather than the rectified one). The rectified Gaussian distribution “outperforms 𝒩(0,1)(in terms of IS) by 15–20% and tends to require fewer iterations.”
The downside is that the “truncation trick”, which yields even larger average improvements in image quality (at the expense of diversity) doesn’t quite apply, and the rectified Gaussian sans truncation produced similar results as the Normal+truncation, so BigGAN reverted to the default Normal distribution+truncation (2020, personal communication).
The truncation trick either directly applies to some of the other distributions, particularly the Rectified Gaussian, or could easily be adapted—possibly yielding an improvement over either approach. The Rectified Gaussian can be truncated just like the default Normals can. And for the Bernoulli, one could decrease p during the generation, or what is probably equivalent, re-sample whenever the variance (ie. squared sum) of all the Bernoulli latent variables exceeds a certain constant. (With p = 0.5, a latent vector of 512 Bernouillis would on average all sum up to simply 0.5 × 512 = 256, with the 2.5%–97.5% quantiles being 234–278, so a ‘truncation trick’ here might be throwing out every vector with a sum above, say, the 80% quantile of 266.)
One also wonders about vectors which draw from multiple distributions rather than just one. Could the StyleGAN 8-FC-layer learned-latent-variable be reverse-engineered? Perhaps the first layer or two merely converts the normal input into a more useful distribution & parameters/training could be saved or insight gained by imitating that.↩︎
-
Which raises the question: if you added any or all of those features, would StyleGAN become that much better? Unfortunately, while theorists & practitioners have had many ideas, so far theory has proven more fecund than fatidical and the large-scale GAN experiments necessary to truly test the suggestions are too expensive for most. Half of these suggestions are great ideas—but which half?↩︎
-
For more on the choice of convolution layers/kernel sizes, see 2015 notes for “CS231n: Convolutional Neural Networks for Visual Recognition”, or take a look at these Convolution animations & Yang’s interactive “Convolution Visualizer”.↩︎
-
One thing people keep trying GANs on, but which never works well, is pixel art, such as Pokemon. The results are always bad: the individual data points will be memorized and generated fine, but the variations and interpolations will be poor, and the GANs are unable to generate meaningfully ‘new’ pixel art, whereas with photographs or art, there is a much greater diversity and genuinely novel compositions.
The problem is that ‘pixel art’ is underspecified. Pixel art is by design an ultra-impoverished representation of art or the real world: under the extreme constraints of a palette enabling a few colors at a time or objects which might max out at 8x8 tiles, it is only enough pixels, carefully reduced to a parody or caricature or abstraction—just enough to trigger the association in the human viewer. Perhaps if one had a lot of the relevant art, that could brute force the problem, but so far no one has convincingly done so.
If you look at 8-bit art and standard block sizes like Mario in NES Mario, if you were not already intimately familiar with the distribution of human faces, and had to learn starting with a completely blank slate like a GAN would, how would you ever understand what a human face was such that you could imagine correct variations like Princess Peach or Luigi? Or if you wanted to generate Pokemon, which are all based heavily on real animals, how would the model know anything about horses or zebras or hamsters or butterflies and be able to generate a sprite of Butterfree independently? (One is reminded of the joke about the mathematicians telling jokes. They knew all each other’s favorites, you see, so they only needed to call out the number. “#9.” Sensible chuckles. “#81.” Laughter. “#17!” Chortling all aroud. The new grad student ventures his own joke: “…#112?” Outright hysteria! You see, they had never heard that one before…)
Successful applications to pixelart tend to inject real-world knowledge, such as through models pretrained on FFHQ, or focus on tasks involving ‘throwing away’ information rather than generating it, such as style transfer of pixelart styles.
Thus, if I wanted to make a Pokemon GAN, I would not attempt to train on solely pixel art scraped from a few games. I would instead start with a large dataset of animals, perhaps from ImageNet or iNaturalist or Wikipedia, real or fictional, and grab all Pokemon art of any kind from anywhere, including dumping individual frames from the Pokemon anime and exploiting CGI models of animals/Pokemon to densely sample all possible images, and would focus on generating as high-quality and diverse a distribution of fantastic beasts as possible; and when that succeeded, treat ‘Pokemon-style pixelization’ as a second phase, to be applied to the high-quality high-resolution photographic fantasy animals generated by the first model.
It is noteworthy that the first really good pixel art neural generative model, CLIPIT PixelDraw, relies entirely on the pretrained CLIP model which was trained on n = 400m Internet images.↩︎
-
This observations apply only to the Generator in GANs (which is what we primarily care about); curiously, there’s some reason to think that GAN Discriminators are in fact mostly memorizing (see later).↩︎
-
A possible alternative is ESRGAN (et al 2018 ); JoeyBallantine’s PyTorch anime models like
4x_NMKD-YandereNeoXL_200k.pth
work much better than waifu2x does (as of 2021-05-28).↩︎ -
Based on eyeballing the ‘cat’ bar graph in Figure 3 of et al 2015 .↩︎
-
CATS offer an amusing instance of the dangers of data augmentation: ProGAN used horizontal flipping/mirroring for everything, because why not? This led to strange Cyrillic text captions showing up in the generated cat images. Why not Latin alphabet captions? Because every cat image was being shown mirrored as well as normally! For StyleGAN, mirroring was disabled, so now the lolcat captions are recognizably Latin alphabetical, and even almost English words. This demonstrates that even datasets where left/right doesn’t seem to matter, like cat photos, can surprise you.↩︎
-
I estimated the total cost using AWS EC2 preemptible hourly costs on 2019-03-15 as follows:
-
1 GPU:
p2.xlarge
instance inus-east-2a
, Half of a K80 (12GB VRAM): $0.3235/hour -
2 GPUs: NA—there is no P2 instance with 2 GPUs, only 1/8/16
-
8 GPUs:
p2.8xlarge
inus-east-2a
, 8 halves of K80s (12GB VRAM each): $2.160/hour
As usual, there is sublinear scaling, and larger instances cost disproportionately more, because one is paying for faster wallclock training (time is valuable) and for not having to create a distributed infrastructure which can exploit the cheap single-GPU instances.
This cost estimate does not count additional costs like hard drive space. In addition to the dataset size (the StyleGAN data encoding is ~18× larger than the raw data size, so a 10GB folder of images → 200GB of
.tfrecords
), you would need at least 100GB HDD (50GB for the OS, and 50GB for checkpoints/images/etc to avoid crashes from running out of space).↩︎ -
-
I regard this as a flaw in StyleGAN & TF in general. Computers are more than fast enough to load & process images asynchronously using a few worker threads, and working with a directory of images (rather than a special binary format 10–20× larger) avoids imposing serious burdens on the user & hard drive. PyTorch GANs almost always avoid this mistake, and are much more pleasant to work with as one can freely modify the dataset between (and even during) runs.↩︎
-
For example, my Danbooru2018 anime portrait dataset is 16GB, but the StyleGAN encoded dataset is 296GB.↩︎
-
This may be why some people report that StyleGAN just crashes for them & they can’t figure out why. They should try changing their dataset JPG ↔︎ PNG.↩︎
-
That is, in training G, the G’s fake images must be augmented before being passed to the D for rating; and in training D, both real & fake images must be augmented the same way before being passed to D. Previously, all GAN researchers appear to have assumed that one should only augment real images before passing to D during D training, which conveniently can be done at dataset creation; unfortunately, this hidden assumption turns out to be about the most harmful way possible!↩︎
-
I would describe the distinctions as: Software 0.0 was imperative programming for hammering out clockwork mechanism; Software 1.0 was declarative programming with specification of policy; and Software 2.0 is deep learning by gardening loss functions (with everything else, from model arch to which datapoints to label ideally learned end-to-end). Continuing the theme, we might say that dialogue with models, like “prompt programming”, are “Software 3.0”…↩︎
-
But you may not want to–remember the lolcat captions!↩︎
-
Note: If you use a different command to resize, check it thoroughly. With ImageMagick, if you use the
^
operator like-resize 512x512^
, you will not get exactly 512×512px images as you need; while if you use the!
operator like-resize 512x512!
, the images will be exactly 512×512px but the aspect ratios will distorted to make images fit, and this may confuse anything you are training by introducing unnecessary meaningless distortions & will make any generated images look bad.↩︎ -
If you are using Python 2, you will get
print
syntax error messages; if you are using Python 3–3.6, you will get ‘type hint’ errors.↩︎ -
Stas Podgorskiy has demonstrated that the StyleGAN 2 correction can be reverse-engineered and applied back to StyleGAN 1 generators if necessary.↩︎
-
This makes it conform to a truncated normal distribution; why truncated rather than rectified/winsorized at a max like 0.5 or 1.0 instead? Because then many, possibly most, of the latent variables would all be at the max, instead of smoothly spread out over the permitted range.↩︎
-
No minibatches are used, so this is much slower than necessary.↩︎
-
Simply encoding each possible tag as a one-hot categorical variable would scale poorly: in the worst case, Danbooru2020 has >434,000 possible tags. If that was passed into a fully-connected layer, which output a 1024-long embedding, then that would use up 434,000 × 1,024 = 444 million parameters! The embedding would be larger than the actual StyleGAN model, and accordingly expensive. RNNs historically are commonly used to convert text inputs to an embedding for a CNN to process, but they are finicky and hard to work with. Word2vec doesn’t work because, as the name suggests, it only converts a single tag/word at a time into an embedding; doc2vec is its equivalent for sequences of text. If we were doing it in 2021, we would probably just throw a Transformer at the raw text (attention is all you need!) with a window of 512 tokens or something. (You don’t need that many tags, and it’s unlikely that a feasible model would make good use of ~100 tags anyway.)↩︎
-
Keep this change in mind if you run into errors like
ValueError: Cannot feed value of shape (1024,) for Tensor 'G/dlatent_avg/new_value:0', which has shape '(512,)'
trying to reuse the model. Try increase the size of thelatent_size
anddlatent_size
512 → 1024 in thenetworks_stylegan2.py
config.↩︎ -
Random cropping takes the sub-image from anywhere in the image which results in a square; ‘top-cropping’ aligns to the top edge, and trims an equal amount left/right. This works better with images containing humanoid figures (avoiding cutting off their heads or the top of their body), and the increased consistency appears critical for avoiding destabilizing BigGAN early on.↩︎
-
Moving self-attention around in BigGAN also makes surprisingly little difference. We discussed it with BigGAN’s Brock, and he noted that self-attention was expensive & never seemed to be as important to BigGAN as one would assume (compared to other improvements like the orthogonal regularization, large models, and large minibatches). Given examples like Jukebox, VQGAN, not-so-BigGAN, or DALL·E 1, I suspect that the benefits of self-attention may be relatively minimal at the raw pixel level, and better focused on the ‘semantic level’ in some sense, such as in processing the latent vector or VQ-VAE tokens.↩︎
-
The question is not whether one is to start with an initialization at all, but whether to start with one which does everything poorly, or one which does a few similar things well. Similarly, from a Bayesian statistics perspective, the question of what prior to use is one that everyone faces; however, many approaches sweep it under the rug and effectively assume a default flat prior that is consistently bad and optimal for no meaningful problem ever.↩︎
-
StyleGAN2-ADA is reportedly much more sample-efficient and reduces the need for transfer learning: et al 2020 . But if a relevant model is available, it should still be used. Backporting the ADA data augmentation trick to StyleGAN1–2 will be a major upgrade.↩︎
-
There are more real Asuka images than Holo to begin with, but there is no particular reason for the 10× data augmentation compared to the Holo’s 3×—the data augmentations were just done at different times and happened to have less or more augmentations enabled.↩︎
-
A famous example is character designer Yoshiyuki Sadamoto demonstrating how to turn Nadia (Nadia: The Secret of Blue Water) into Shinji Ikari (Evangelion).↩︎
-
It turns out that this latent vector trick does work. Intriguingly, it works even better to do ‘model averaging’ or ‘model blending’ (“StyleGAN network blending”/“Resolution Dependent GAN Interpolation for Controllable Image Synthesis Between Domains”, 2020): retrain model A on dataset B, and then take a weighted average of the 2 models (you average them, parameter by parameter, and remarkably, that Just Works, or you can swap out layers between models), and then you can create faces which are arbitrarily in between A and B. So for example, you can blend FFHQ/Western-animation faces (Colab notebook), ukiyo-e/FFHQ faces, furries/foxes/FFHQ faces, or even furries/foxes/FFHQ/anime/ponies.↩︎
-
In retrospect, this shouldn’t’ve surprised me.↩︎
-
There is for other architectures like flow-based ones such as Glow, and this is one of their benefits–while the requirement to be made out of building blocks which can be run backwards & forwards equally well, to be ‘invertible’, is currently extremely expensive and the results not competitive either in final image quality or compute requirements, the invertibility means that encoding an arbitrary real image to get its inferred latents Just Works™ and one can easily morph between 2 arbitrary images, or encode an arbitrary image & edit it in the latent space to do things like add/remove glasses from a face or create an opposites-ex version.↩︎
-
This final approach is, interestingly, the historical reason backpropagation was invented: it corresponds to planning in a model. For example, in planning the flight path of an airplane (1960/1962): the destination or ‘output’ is fixed, the aerodynamics+geography or ‘model parameters’ are also fixed, and the question is what actions determining a flight path will reduce the loss function of time or fuel spent. One starts with a random set of actions picking a random flight path, runs it forward through the environment model, gets a final time/fuel spent, and then backpropagates through the model to get the gradients for the flight path, adjusting the flight path towards a new set of actions which will slightly reduce the time/fuel spent; the new actions are used to plan out the flight to get a new loss, and so on, until a local minimum of the actions has been found. This works with non-stochastic problems; for stochastic ones where the path can’t be guaranteed to be executed, “model-predictive control” can be used to replan at every step and execute adjustments as necessary. Another interesting use of backpropagation for outputs is et al 2019 which tackles the long-standing problem of how to get NNs to output sets rather than list outputs by generating a possible set output & refining it via backpropagation.↩︎
-
SGD is common, but a second-order algorithm like Limited-memory BFGS is often used in these applications in order to run as few iterations as possible.↩︎
-
et al 2019 shows that BigGAN/StyleGAN latent embeddings can also go beyond what one might expect, to include zooms, translations, and other transforms.↩︎
-
Flow models have other advantages, mostly stemming from the maximum likelihood training objective. Since the image can be propagated backwards and forwards losslessly, instead of being limited to generating random samples like a GAN, it’s possible to calculate the exact probability of an image, enabling maximum likelihood as a loss to optimize, and dropping the Discriminator entirely. With no GAN dynamics, there’s no worry about weird training dynamics, and the likelihood loss also forbids ‘mode dropping’: the flow model can’t simply conspire with a Discriminator to forget possible images.↩︎
-
StyleGAN 2 is more computationally expensive but Karras et al optimized the codebase to make up for it, keeping total compute constant.↩︎
-
Backup-backup mirror:
rsync rsync://176.9.41.242:873/biggan/2020-01-11-skylion-stylegan2-animeportraits-networksnapshot-024664.pkl.xz ./
↩︎